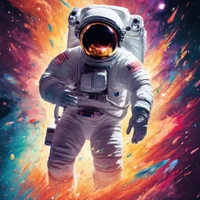
Team Tonic
community
AI & ML interests
vision , multimedia , gradio, accessibility & cool demos
Recent Activity
View all activity
TeamTonic's activity
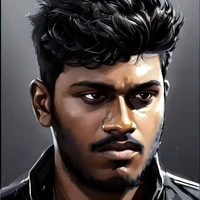
prithivMLmodsΒ
posted
an
update
3 days ago
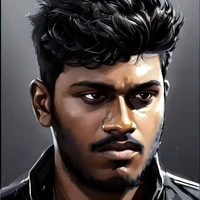
prithivMLmodsΒ
posted
an
update
6 days ago
Post
2031
Qwen2VL Models: Vision and Language Processing π
πFT; [ Latex OCR, Math Parsing, Text Analogy OCRTest ]
βοΈDemo : prithivMLmods/Qwen2-VL-2B . The demo includes the Qwen2VL 2B Base Model.
π―The space handles documenting content from the input image along with standardized plain text. It includes adjustment tools with over 30 font styles, file formatting support for PDF and DOCX, textual alignments, font size adjustments, and line spacing modifications.
πPDFs are rendered using the ReportLab software library toolkit.
π§΅Models :
+ prithivMLmods/Qwen2-VL-OCR-2B-Instruct
+ prithivMLmods/Qwen2-VL-Ocrtest-2B-Instruct
+ prithivMLmods/Qwen2-VL-Math-Prase-2B-Instruct
πSample Document :
+ https://drive.google.com/file/d/1Hfqqzq4Xc-3eTjbz-jcQY84V5E1YM71E/view?usp=sharing
π¦Collection :
+ prithivMLmods/vision-language-models-67639f790e806e1f9799979f
.
.
.
@prithivMLmods π€
πFT; [ Latex OCR, Math Parsing, Text Analogy OCRTest ]
βοΈDemo : prithivMLmods/Qwen2-VL-2B . The demo includes the Qwen2VL 2B Base Model.
π―The space handles documenting content from the input image along with standardized plain text. It includes adjustment tools with over 30 font styles, file formatting support for PDF and DOCX, textual alignments, font size adjustments, and line spacing modifications.
πPDFs are rendered using the ReportLab software library toolkit.
π§΅Models :
+ prithivMLmods/Qwen2-VL-OCR-2B-Instruct
+ prithivMLmods/Qwen2-VL-Ocrtest-2B-Instruct
+ prithivMLmods/Qwen2-VL-Math-Prase-2B-Instruct
πSample Document :
+ https://drive.google.com/file/d/1Hfqqzq4Xc-3eTjbz-jcQY84V5E1YM71E/view?usp=sharing
π¦Collection :
+ prithivMLmods/vision-language-models-67639f790e806e1f9799979f
.
.
.
@prithivMLmods π€
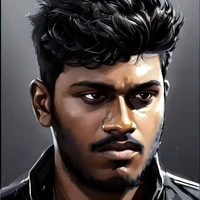
prithivMLmodsΒ
posted
an
update
7 days ago
Post
3165
π Here Before - Xmasπ
β¨
π§π»βπModels
+ [ Xmas 2D Illustration ] : strangerzonehf/Flux-Xmas-Illustration-LoRA
+ [ Xmas 3D Art ] : strangerzonehf/Flux-Xmas-3D-LoRA
+ [ Xmas Chocolate ] : strangerzonehf/Flux-Xmas-Chocolate-LoRA
+ [ Xmas Isometric Kit ] : strangerzonehf/Flux-Xmas-Isometric-Kit-LoRA
+ [ Xmas Realpix ] : strangerzonehf/Flux-Xmas-Realpix-LoRA
+ [ Xmas Anime ] : strangerzonehf/Flux-Anime-Xmas-LoRA
βοΈCollections
+ [ Xmas Art ] : strangerzonehf/christmas-pack-6758b199487adafaddb68f82
+ [ Stranger Zone Collection ] : prithivMLmods/stranger-zone-collections-org-6737118adcf2cb40d66d0c7e
π₯ΆPage
+ [ Stranger Zone ] : https://huggingface.co/strangerzonehf
.
.
.
@prithivMLmods π€
π§π»βπModels
+ [ Xmas 2D Illustration ] : strangerzonehf/Flux-Xmas-Illustration-LoRA
+ [ Xmas 3D Art ] : strangerzonehf/Flux-Xmas-3D-LoRA
+ [ Xmas Chocolate ] : strangerzonehf/Flux-Xmas-Chocolate-LoRA
+ [ Xmas Isometric Kit ] : strangerzonehf/Flux-Xmas-Isometric-Kit-LoRA
+ [ Xmas Realpix ] : strangerzonehf/Flux-Xmas-Realpix-LoRA
+ [ Xmas Anime ] : strangerzonehf/Flux-Anime-Xmas-LoRA
βοΈCollections
+ [ Xmas Art ] : strangerzonehf/christmas-pack-6758b199487adafaddb68f82
+ [ Stranger Zone Collection ] : prithivMLmods/stranger-zone-collections-org-6737118adcf2cb40d66d0c7e
π₯ΆPage
+ [ Stranger Zone ] : https://huggingface.co/strangerzonehf
.
.
.
@prithivMLmods π€
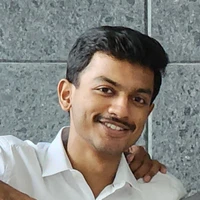
peaceAshΒ
authored
a
paper
8 days ago
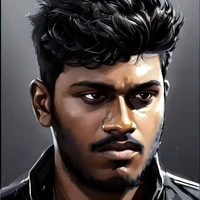
prithivMLmodsΒ
posted
an
update
11 days ago
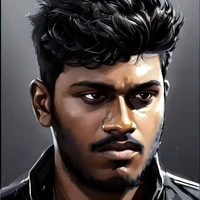
prithivMLmodsΒ
posted
an
update
19 days ago
Post
3816
Near 3:2 { 1280*832 } Adapters π₯
π§ͺThe datasets were prepared for a 3:2 aspect ratio by processing images of any dimension (width Γ height) in alignment with the adapter's concept. This involved using techniques such as magic expand, magic fill, or outpainting to adjust the remaining parts of the image to achieve the 3:2 ratio & posts training. This approach enhanced the desired image quality to up to 2 MB for detailed prompts and reduced artifacts in images sized at 1280 Γ 832.
πThis approach was used instead of cropping down the 2x or 3x zoomed positions in the actual image. It generative filling to adjust the image's aspect ratio proportionally within the dataset.
π§I used Canva's Magic Expand, Firefly's Generative Fill, and Flux's Outpaint for aspect ratio adjustments.
β¬οΈModel DLC :
+ [ Microworld Nft ] : strangerzonehf/Flux-Microworld-NFT-LoRA
+ [ Creative Stocks ] : strangerzonehf/Flux-Creative-Stocks-LoRA
+ [ Icon-Kit ] : strangerzonehf/Flux-Icon-Kit-LoRA
+ [ Claymation ] : strangerzonehf/Flux-Claymation-XC-LoRA
+ [ Super Portrait ] : strangerzonehf/Flux-Super-Portrait-LoRA
+ [ Ghibli Art ] : strangerzonehf/Flux-Ghibli-Art-LoRA
+ [ Isometric Site ] : strangerzonehf/Flux-Isometric-Site-LoRA
π§¨Page :
1] Stranger Zone: https://huggingface.co/strangerzonehf
π£Space :
1] Flux LoRA DLC: prithivMLmods/FLUX-LoRA-DLC
π¦Collections :
1] strangerzonehf/flux-3dxl-engine-674833c14a001d5b1fdb5139
2] prithivMLmods/flux-lora-collections-66dd5908be2206cfaa8519be
3] strangerzonehf/animaker-engine-673714956dec98c400c30cf6
4] strangerzonehf/mixer-engine-673582c9c5939d8aa5bf9533
.
.
.
@prithivMLmods
π§ͺThe datasets were prepared for a 3:2 aspect ratio by processing images of any dimension (width Γ height) in alignment with the adapter's concept. This involved using techniques such as magic expand, magic fill, or outpainting to adjust the remaining parts of the image to achieve the 3:2 ratio & posts training. This approach enhanced the desired image quality to up to 2 MB for detailed prompts and reduced artifacts in images sized at 1280 Γ 832.
πThis approach was used instead of cropping down the 2x or 3x zoomed positions in the actual image. It generative filling to adjust the image's aspect ratio proportionally within the dataset.
π§I used Canva's Magic Expand, Firefly's Generative Fill, and Flux's Outpaint for aspect ratio adjustments.
β¬οΈModel DLC :
+ [ Microworld Nft ] : strangerzonehf/Flux-Microworld-NFT-LoRA
+ [ Creative Stocks ] : strangerzonehf/Flux-Creative-Stocks-LoRA
+ [ Icon-Kit ] : strangerzonehf/Flux-Icon-Kit-LoRA
+ [ Claymation ] : strangerzonehf/Flux-Claymation-XC-LoRA
+ [ Super Portrait ] : strangerzonehf/Flux-Super-Portrait-LoRA
+ [ Ghibli Art ] : strangerzonehf/Flux-Ghibli-Art-LoRA
+ [ Isometric Site ] : strangerzonehf/Flux-Isometric-Site-LoRA
π§¨Page :
1] Stranger Zone: https://huggingface.co/strangerzonehf
π£Space :
1] Flux LoRA DLC: prithivMLmods/FLUX-LoRA-DLC
π¦Collections :
1] strangerzonehf/flux-3dxl-engine-674833c14a001d5b1fdb5139
2] prithivMLmods/flux-lora-collections-66dd5908be2206cfaa8519be
3] strangerzonehf/animaker-engine-673714956dec98c400c30cf6
4] strangerzonehf/mixer-engine-673582c9c5939d8aa5bf9533
.
.
.
@prithivMLmods
Post
1488
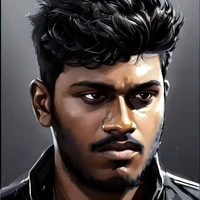
prithivMLmodsΒ
posted
an
update
22 days ago
Post
2626
Milestone for Flux.1 Dev π₯
π’The Flux.1 Dev model has crossed 1οΈβ£0οΈβ£,0οΈβ£0οΈβ£0οΈβ£ creative public adapters! π
π https://huggingface.co/models?other=base_model:adapter:black-forest-labs/FLUX.1-dev
π’This includes:
- 266 Finetunes
- 19 Quants
- 4 Merges
π’ Hereβs the 10,000th public adapter : π
+ strangerzonehf/Flux-3DXL-Partfile-0006
π’ Page :
+ https://huggingface.co/strangerzonehf
π’ Collection :
+ prithivMLmods/flux-lora-collections-66dd5908be2206cfaa8519be
π’The Flux.1 Dev model has crossed 1οΈβ£0οΈβ£,0οΈβ£0οΈβ£0οΈβ£ creative public adapters! π
π https://huggingface.co/models?other=base_model:adapter:black-forest-labs/FLUX.1-dev
π’This includes:
- 266 Finetunes
- 19 Quants
- 4 Merges
π’ Hereβs the 10,000th public adapter : π
+ strangerzonehf/Flux-3DXL-Partfile-0006
π’ Page :
+ https://huggingface.co/strangerzonehf
π’ Collection :
+ prithivMLmods/flux-lora-collections-66dd5908be2206cfaa8519be
Post
432
π The Stanford Institute for Human-Centered AI (https://aiindex.stanford.edu/vibrancy/) has released its 2024 Global AI Vibrancy Tool, a way to explore and compare AI progress across 36 countries.
π It measures progress across the 8 broad pillars of R&D, Responsible AI, Economy, Education, Diversity, Policy and Governance, Public Opinion and Infrastructure. (Each of these pillars have a number of Sub Indices)
π As a whole it is not surprising that the USA was at the top in terms of overall score as of 2023 (AI investment activity is a large part of the economic pillar for example and that is a large part of the overall USA ranking) but drilling in to more STRATEGIC Macro pillars like Education, Infrastructure or R&D reveal interesting growth patterns in Asia (particularly China) and Western Europe that I suspect the 2024 metrics will bear out.
π€ Hopefully the 2024 Global Vibrancy ranking will break out AI and ML verticals like Computer Vision or NLP and or the AI Agent space as that may also from a global macro level give indications of what is to come globally for AI in 2025.
π It measures progress across the 8 broad pillars of R&D, Responsible AI, Economy, Education, Diversity, Policy and Governance, Public Opinion and Infrastructure. (Each of these pillars have a number of Sub Indices)
π As a whole it is not surprising that the USA was at the top in terms of overall score as of 2023 (AI investment activity is a large part of the economic pillar for example and that is a large part of the overall USA ranking) but drilling in to more STRATEGIC Macro pillars like Education, Infrastructure or R&D reveal interesting growth patterns in Asia (particularly China) and Western Europe that I suspect the 2024 metrics will bear out.
π€ Hopefully the 2024 Global Vibrancy ranking will break out AI and ML verticals like Computer Vision or NLP and or the AI Agent space as that may also from a global macro level give indications of what is to come globally for AI in 2025.
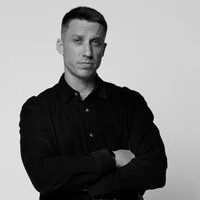
ZennyKennyΒ
posted
an
update
23 days ago
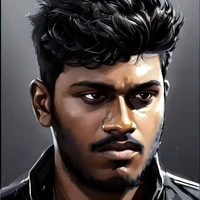
prithivMLmodsΒ
posted
an
update
26 days ago
Post
2725
Fine-Textured [Polygon] Character 3D Design Renders π
Adapters capable of providing better lighting control (Bn+, Bn-) and richer textures compared to previous sets require more contextual prompts for optimal performance.
The ideal settings are achieved at inference steps around 30β35, with the best dimensions being 1280 x 832 [ 3:2 ]. However, it also performs well with the default settings of 1024 x 1024 [ 1:1 ].
π’Models DLC :
+ strangerzonehf/Flux-3DXL-Partfile-0001
+ strangerzonehf/Flux-3DXL-Partfile-0002
+ strangerzonehf/Flux-3DXL-Partfile-0003
+ strangerzonehf/Flux-3DXL-Partfile-0004
+ strangerzonehf/Flux-3DXL-Partfile-C0001
π’Collections :
1] strangerzonehf/flux-3dxl-engine-674833c14a001d5b1fdb5139
2] prithivMLmods/flux-lora-collections-66dd5908be2206cfaa8519be
π’Space :
1] prithivMLmods/FLUX-LoRA-DLC
π’Page :
1] Stranger Zone: https://huggingface.co/strangerzonehf
.
.
.
@prithivMLmods π€
Adapters capable of providing better lighting control (Bn+, Bn-) and richer textures compared to previous sets require more contextual prompts for optimal performance.
The ideal settings are achieved at inference steps around 30β35, with the best dimensions being 1280 x 832 [ 3:2 ]. However, it also performs well with the default settings of 1024 x 1024 [ 1:1 ].
π’Models DLC :
+ strangerzonehf/Flux-3DXL-Partfile-0001
+ strangerzonehf/Flux-3DXL-Partfile-0002
+ strangerzonehf/Flux-3DXL-Partfile-0003
+ strangerzonehf/Flux-3DXL-Partfile-0004
+ strangerzonehf/Flux-3DXL-Partfile-C0001
π’Collections :
1] strangerzonehf/flux-3dxl-engine-674833c14a001d5b1fdb5139
2] prithivMLmods/flux-lora-collections-66dd5908be2206cfaa8519be
π’Space :
1] prithivMLmods/FLUX-LoRA-DLC
π’Page :
1] Stranger Zone: https://huggingface.co/strangerzonehf
.
.
.
@prithivMLmods π€
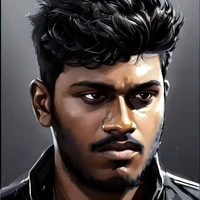
prithivMLmodsΒ
posted
an
update
30 days ago
Post
3271
HF Posts Receipts ππ
[ HF POSTS RECEIPT ] : prithivMLmods/HF-POSTS-RECEIPT
π₯ The one thing that needs to be remembered is the 'username'.
π₯ And yeah, thank you, @maxiw , for creating the awesome dataset and sharing them here! π
π₯ [ Dataset ] : maxiw/hf-posts
.
.
.
@prithivMLmods
[ HF POSTS RECEIPT ] : prithivMLmods/HF-POSTS-RECEIPT
π₯ The one thing that needs to be remembered is the 'username'.
π₯ And yeah, thank you, @maxiw , for creating the awesome dataset and sharing them here! π
π₯ [ Dataset ] : maxiw/hf-posts
.
.
.
@prithivMLmods
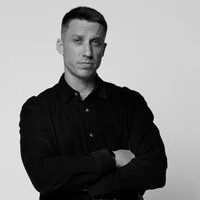
ZennyKennyΒ
posted
an
update
30 days ago
Post
1205
I've joined the Bluesky community. Interested to see what decentralized social media looks like in action: https://bsky.app/profile/kghamilton.bsky.social
Looking forward to following other AI builders, tech enthusiasts, goth doomscrollers, and ironic meme creators.
Looking forward to following other AI builders, tech enthusiasts, goth doomscrollers, and ironic meme creators.
Post
692
π€π» Function Calling is a key component of Agent workflows. To call functions, an LLM needs a way to interact with other systems and run code. This usually means connecting it to a runtime environment that can handle function calls, data, and security.
Per the Berkeley Function-Calling Leaderboard there are only 2 fully open source models (The other 2 in the top 20 that are not closed source have cc-by-nc-4.0 licenses) out of the top 20 models that currently have function calling built in as of 17 Nov 2024.
https://gorilla.cs.berkeley.edu/leaderboard.html
The 2 Open Source Models out of the top 20 that currently support function calling are:
meetkai/functionary-medium-v3.1
Team-ACE/ToolACE-8B
This is a both a huge disadvantage AND an opportunity for the Open Source community as Enterprises, Small Business, Government Agencies etc. quickly adopt Agents and Agent workflows over the next few months. Open Source will have a lot of catching up to do as Enterprises will be hesitant to switch from the closed source models that they may initially build their Agent workflows on in the next few months to an open source alternative later.
Hopefully more open source models will support function calling in the near future.
Per the Berkeley Function-Calling Leaderboard there are only 2 fully open source models (The other 2 in the top 20 that are not closed source have cc-by-nc-4.0 licenses) out of the top 20 models that currently have function calling built in as of 17 Nov 2024.
https://gorilla.cs.berkeley.edu/leaderboard.html
The 2 Open Source Models out of the top 20 that currently support function calling are:
meetkai/functionary-medium-v3.1
Team-ACE/ToolACE-8B
This is a both a huge disadvantage AND an opportunity for the Open Source community as Enterprises, Small Business, Government Agencies etc. quickly adopt Agents and Agent workflows over the next few months. Open Source will have a lot of catching up to do as Enterprises will be hesitant to switch from the closed source models that they may initially build their Agent workflows on in the next few months to an open source alternative later.
Hopefully more open source models will support function calling in the near future.
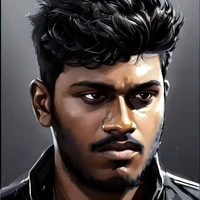
prithivMLmodsΒ
posted
an
update
about 1 month ago
Post
4100
CRISP π₯ [ Isometric-3D-Cinematography / Isometric-3D-Obj / 3D-Kawaii / Long Toons ]
[ Flux DLC ] : prithivMLmods/FLUX-LoRA-DLC
[ Stranger Zone ] : https://huggingface.co/strangerzonehf
π[ Isometric 3D Cinematography ] : strangerzonehf/Flux-Isometric-3D-Cinematography
π[ Isometric 3D ] : strangerzonehf/Flux-Isometric-3D-LoRA
π[ Cute 3D Kawaii ] : strangerzonehf/Flux-Cute-3D-Kawaii-LoRA
π[ Long Toon 3D ] : prithivMLmods/Flux-Long-Toon-LoRA
[ Stranger Zone Collection ] : https://huggingface.co/collections/prithivMLmods/stranger-zone-collections-6737118adcf2cb40d66d0c7e
[ Flux Collection ] : prithivMLmods/flux-lora-collections-66dd5908be2206cfaa8519be
[ Flux Mix ] : prithivMLmods/Midjourney-Flux
.
.
.
@prithivMLmods
[ Flux DLC ] : prithivMLmods/FLUX-LoRA-DLC
[ Stranger Zone ] : https://huggingface.co/strangerzonehf
π[ Isometric 3D Cinematography ] : strangerzonehf/Flux-Isometric-3D-Cinematography
π[ Isometric 3D ] : strangerzonehf/Flux-Isometric-3D-LoRA
π[ Cute 3D Kawaii ] : strangerzonehf/Flux-Cute-3D-Kawaii-LoRA
π[ Long Toon 3D ] : prithivMLmods/Flux-Long-Toon-LoRA
[ Stranger Zone Collection ] : https://huggingface.co/collections/prithivMLmods/stranger-zone-collections-6737118adcf2cb40d66d0c7e
[ Flux Collection ] : prithivMLmods/flux-lora-collections-66dd5908be2206cfaa8519be
[ Flux Mix ] : prithivMLmods/Midjourney-Flux
.
.
.
@prithivMLmods
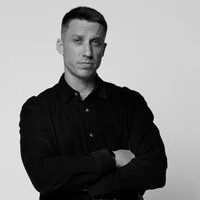
ZennyKennyΒ
posted
an
update
about 1 month ago
Post
345
Using AI to teach English as a Foreign Language? EFL teachers often have busy schedules, variable class sizes, and unexpected cancellations. Introducting VocabSova:
ZennyKenny/VocabSova
VocabSova is a simple chatbot interface that helps teachers create topical vocabulary lists, custom worksheets using that vocabulary, and group activities on a defined theme for a specific English-speaking level (according to CEFR international standards).
There is a great use case for AI in nearly every field, and language learning is a particularly apt domain in my opinion. VocabSova is in active development during its Alpha release, all feedback welcome.
VocabSova is a simple chatbot interface that helps teachers create topical vocabulary lists, custom worksheets using that vocabulary, and group activities on a defined theme for a specific English-speaking level (according to CEFR international standards).
There is a great use case for AI in nearly every field, and language learning is a particularly apt domain in my opinion. VocabSova is in active development during its Alpha release, all feedback welcome.
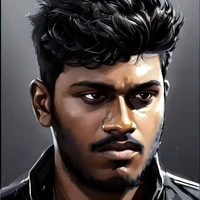
prithivMLmodsΒ
posted
an
update
about 1 month ago
Post
2903
Weekend Dribble π¦πΊ
Adapters for Product Ad Backdrops, Smooth Polaroids, Minimalist Sketch cards, Super Blends!!
π€Demo on: prithivMLmods/FLUX-LoRA-DLC
Stranger Zones :
ππΌ{ Super Blend } : strangerzonehf/Flux-Super-Blend-LoRA
ππΌ{ Product Concept Ad } : prithivMLmods/Flux-Product-Ad-Backdrop
ππΌ{ Frosted Mock-ups } : prithivMLmods/Flux.1-Dev-Frosted-Container-LoRA
ππΌ{ Polaroid Plus } : prithivMLmods/Flux-Polaroid-Plus
ππΌ{Sketch Cards} : prithivMLmods/Flux.1-Dev-Sketch-Card-LoRA
πStranger Zone: https://huggingface.co/strangerzonehf
πFlux LoRA Collections: prithivMLmods/flux-lora-collections-66dd5908be2206cfaa8519be
.
.
.
@prithivMLmods π€
Adapters for Product Ad Backdrops, Smooth Polaroids, Minimalist Sketch cards, Super Blends!!
π€Demo on: prithivMLmods/FLUX-LoRA-DLC
Stranger Zones :
ππΌ{ Super Blend } : strangerzonehf/Flux-Super-Blend-LoRA
ππΌ{ Product Concept Ad } : prithivMLmods/Flux-Product-Ad-Backdrop
ππΌ{ Frosted Mock-ups } : prithivMLmods/Flux.1-Dev-Frosted-Container-LoRA
ππΌ{ Polaroid Plus } : prithivMLmods/Flux-Polaroid-Plus
ππΌ{Sketch Cards} : prithivMLmods/Flux.1-Dev-Sketch-Card-LoRA
πStranger Zone: https://huggingface.co/strangerzonehf
πFlux LoRA Collections: prithivMLmods/flux-lora-collections-66dd5908be2206cfaa8519be
.
.
.
@prithivMLmods π€
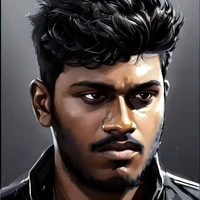
prithivMLmodsΒ
posted
an
update
about 1 month ago
Post
2028
π
Glif App's Remixes feature allows you to slap a logo onto anything, seamlessly integrating the input image (logo) into various contexts. The result is stunning remixes that blend the input logo with generated images (img2img logo mapping) for incredible outcomes.
Check out Any Logo Anywhere remixes on Glif: [Glif Remixes](https://glif.app/glifs/cm3o7dfsd002610z48sz89yih/remixes)
πThe browser extension enables thousands of Glif-based img2img workflows on any image you find online. Experience Glif Remix with WebAI: [Chrome Extension](https://chromewebstore.google.com/detail/glif-remix-the-web-with-a/abfbooehhdjcgmbmcpkcebcmpfnlingo)
.
.
.
π€Have fun with the cool stuff !!
@prithivMLmods
Check out Any Logo Anywhere remixes on Glif: [Glif Remixes](https://glif.app/glifs/cm3o7dfsd002610z48sz89yih/remixes)
πThe browser extension enables thousands of Glif-based img2img workflows on any image you find online. Experience Glif Remix with WebAI: [Chrome Extension](https://chromewebstore.google.com/detail/glif-remix-the-web-with-a/abfbooehhdjcgmbmcpkcebcmpfnlingo)
.
.
.
π€Have fun with the cool stuff !!
@prithivMLmods
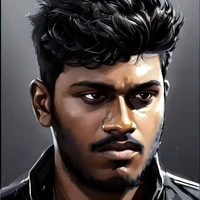
prithivMLmodsΒ
posted
an
update
about 1 month ago
Post
3233
The (768 x 1024) mix of MidJourney and Flux's LoRA is nearly identical to the actual visual design. It hasnβt undergone much concept art development for now. In the meantime, try out the impressive visual designs on:
π₯Midjourney Flux Mix : prithivMLmods/Midjourney-Flux
π₯Adapter for FluxMix : strangerzonehf/Flux-Midjourney-Mix-LoRA
π₯Flux LoRA Collection: prithivMLmods/flux-lora-collections-66dd5908be2206cfaa8519be
.
.
.
@prithivMLmods π€
π₯Midjourney Flux Mix : prithivMLmods/Midjourney-Flux
π₯Adapter for FluxMix : strangerzonehf/Flux-Midjourney-Mix-LoRA
π₯Flux LoRA Collection: prithivMLmods/flux-lora-collections-66dd5908be2206cfaa8519be
.
.
.
@prithivMLmods π€