💵 Donate to OpenAccess AI Collective to help us keep building great tools and models!
Minotaur 15B 8K
Minotaur 15B is an instruct fine-tuned model on top of Starcoder Plus. Minotaur 15B is fine-tuned on only completely open datasets making this model reproducible by anyone. Minotaur 15B has a context length of 8K tokens, allowing for strong recall at long contexts.
Questions, comments, feedback, looking to donate, or want to help? Reach out on our Discord or email [email protected]
Prompts
Chat only style prompts using USER:
,ASSISTANT:
.
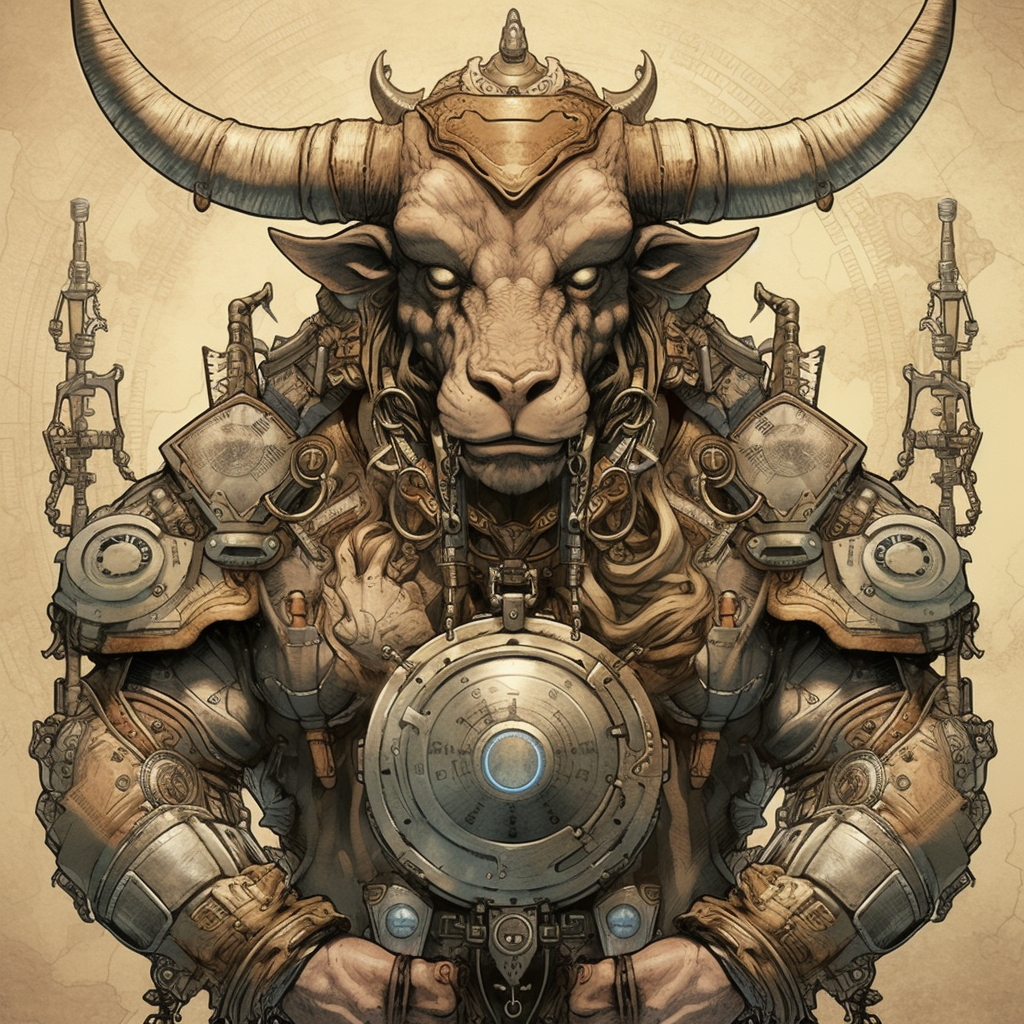
Training Datasets
Minotaur 15B model is fine-tuned on the following openly available datasets:
- WizardLM
- subset of QingyiSi/Alpaca-CoT for roleplay and CoT
- GPTeacher-General-Instruct
- metaeval/ScienceQA_text_only - instruct for concise responses
- openai/summarize_from_feedback - instruct augmented tl;dr summarization
- camel-ai/math
- camel-ai/physics
- camel-ai/chemistry
- camel-ai/biology
- winglian/evals - instruct augmented datasets
- custom sysnthetic datasets around misconceptions, in-context qa, jokes, N-tasks problems, and context-insensitivity
- ARC-Easy & ARC-Challenge - instruct augmented for detailed responses, derived from the
train
split - hellaswag - 30K+ rows of instruct augmented for detailed explanations w 30K+ rows, derived from the
train
split - riddle_sense - instruct augmented, derived from the
train
split - gsm8k - instruct augmented, derived from the
train
split - prose generation
Shoutouts
Special thanks to Nanobit for helping with Axolotl and TheBloke for quantizing these models are more accessible to all.
Demo
HF Demo in Spaces available in the Community ChatBot Arena under the OAAIC Chatbots tab.
Release Notes
Build
Minotaur was built with Axolotl on 4XA100 80GB
- 1 epochs taking approximately 30 hours
- Trained using QLoRA techniques
Bias, Risks, and Limitations
Minotaur has not been aligned to human preferences with techniques like RLHF or deployed with in-the-loop filtering of responses like ChatGPT, so the model can produce problematic outputs (especially when prompted to do so). Minotaur was fine-tuned from the base model StarCoder, please refer to its model card's Limitations Section for relevant information. (included below)
Benchmarks
TBD
Examples
TBD
StarCoderPlus
Play with the instruction-tuned StarCoderPlus at StarChat-Beta.
Table of Contents
Model Summary
StarCoderPlus is a fine-tuned version of StarCoderBase on 600B tokens from the English web dataset RedefinedWeb combined with StarCoderData from The Stack (v1.2) and a Wikipedia dataset. It's a 15.5B parameter Language Model trained on English and 80+ programming languages. The model uses Multi Query Attention, a context window of 8192 tokens, and was trained using the Fill-in-the-Middle objective on 1.6 trillion tokens.
- Repository: bigcode/Megatron-LM
- Project Website: bigcode-project.org
- Point of Contact: [email protected]
- Languages: English & 80+ Programming languages
Use
Intended use
The model was trained on English and GitHub code. As such it is not an instruction model and commands like "Write a function that computes the square root." do not work well. However, the instruction-tuned version in StarChat makes a capable assistant.
Feel free to share your generations in the Community tab!
Generation
# pip install -q transformers
from transformers import AutoModelForCausalLM, AutoTokenizer
checkpoint = "bigcode/starcoderplus"
device = "cuda" # for GPU usage or "cpu" for CPU usage
tokenizer = AutoTokenizer.from_pretrained(checkpoint)
model = AutoModelForCausalLM.from_pretrained(checkpoint).to(device)
inputs = tokenizer.encode("def print_hello_world():", return_tensors="pt").to(device)
outputs = model.generate(inputs)
print(tokenizer.decode(outputs[0]))
Fill-in-the-middle
Fill-in-the-middle uses special tokens to identify the prefix/middle/suffix part of the input and output:
input_text = "<fim_prefix>def print_hello_world():\n <fim_suffix>\n print('Hello world!')<fim_middle>"
inputs = tokenizer.encode(input_text, return_tensors="pt").to(device)
outputs = model.generate(inputs)
print(tokenizer.decode(outputs[0]))
Attribution & Other Requirements
The training code dataset of the model was filtered for permissive licenses only. Nevertheless, the model can generate source code verbatim from the dataset. The code's license might require attribution and/or other specific requirements that must be respected. We provide a search index that let's you search through the pretraining data to identify where generated code came from and apply the proper attribution to your code.
Limitations
The model has been trained on a mixture of English text from the web and GitHub code. Therefore it might encounter limitations when working with non-English text, and can carry the stereotypes and biases commonly encountered online. Additionally, the generated code should be used with caution as it may contain errors, inefficiencies, or potential vulnerabilities. For a more comprehensive understanding of the base model's code limitations, please refer to See StarCoder paper.
Training
StarCoderPlus is a fine-tuned version on 600B English and code tokens of StarCoderBase, which was pre-trained on 1T code tokens. Below are the fine-tuning details:
Model
- Architecture: GPT-2 model with multi-query attention and Fill-in-the-Middle objective
- Finetuning steps: 150k
- Finetuning tokens: 600B
- Precision: bfloat16
Hardware
- GPUs: 512 Tesla A100
- Training time: 14 days
Software
- Orchestration: Megatron-LM
- Neural networks: PyTorch
- BP16 if applicable: apex
License
The model is licensed under the BigCode OpenRAIL-M v1 license agreement. You can find the full agreement here.
- Downloads last month
- 13