lumaticai/BongLlama-1.1B-Chat-alpha-v0
Introducing BongLlama by LumaticAI. A finetuned version of TinyLlama 1.1B Chat on Bengali Dataset.
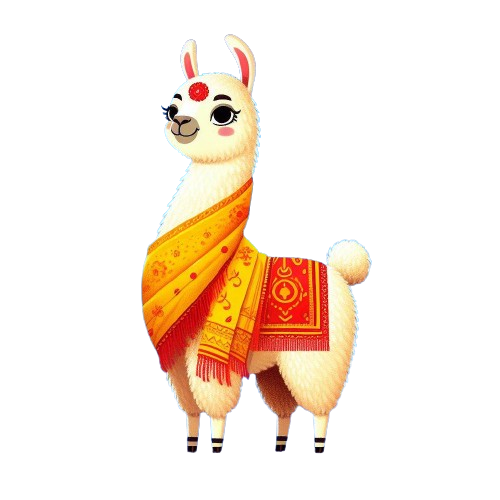
Model Details
Model Description
Bongllama is a sub-part of our company's initiative for developing Indic and Regional Large Language Models. We are LumaticAI continuously working on helping our clients build Custom AI Solutions for their organization. We have taken an initiative to launch open source models specific to regions and languages.
Bongllama is a LLM built for West Bengal on Bengali dataset. It's a 1.1B parameters model. We have used a Bengali dataset of 10k data i.e lumatic-ai/BongChat-10k-v0 and finetuned on TinyLlama/TinyLlama-1.1B-Chat-v1.0 model to get our BongLlama 1.1B Chat Alpha v0 model.
We are continuously working on training and developing this model and improve it. We are also going to launch this model with various sizes of different LLM's and Datasets.
- Developed by: LumaticAI
- Shared by [Optional]: LumaticAI
- Model type: Language model
- Language(s) (NLP): en, bn
- License: mit
- Parent Model: TinyLlama/TinyLlama-1.1B-Chat-v1.0
Uses
Direct Use
- base model for further finetuning
- get an overview of how indic LLM work on specific language
- for fun
Downstream Use
- can be deployed with api
- used to create webapp or app to show demo
Out-of-Scope Use
- cannot be used for production purpose
- cannot be used to generate text for research or academic purposes
Bias, Risks, and Limitations
Significant research has explored bias and fairness issues with language models (see, e.g., Sheng et al. (2021) and Bender et al. (2021)). Predictions generated by the model may include disturbing and harmful stereotypes across protected classes; identity characteristics; and sensitive, social, and occupational groups.
How to Get Started with the Model
Use the code below to get started with the model.
Click to expand
Pipeline
import torch
from transformers import AutoModelForCausalLM, AutoTokenizer
from transformers import pipeline
def formatted_prompt(question)-> str:
return f"<|im_start|>user\n{question}<|im_end|>\n<|im_start|>assistant:"
hub_model_name = "lumatic-ai/BongLlama-1.1B-Chat-alpha-v0"
tokenizer = AutoTokenizer.from_pretrained(hub_model_name)
pipe = pipeline(
"text-generation",
model=hub_model_name,
torch_dtype=torch.float16,
device_map="auto",
)
from time import perf_counter
start_time = perf_counter()
prompt = formatted_prompt('হ্যালো')
sequences = pipe(
prompt,
do_sample=True,
temperature=0.1,
top_p=0.9,
num_return_sequences=1,
eos_token_id=tokenizer.eos_token_id,
max_new_tokens=256
)
for seq in sequences:
print(f"Result: {seq['generated_text']}")
output_time = perf_counter() - start_time
print(f"Time taken for inference: {round(output_time,2)} seconds")
Streaming Response (ChatGPT, Bard like)
import torch
from transformers import AutoModelForCausalLM, AutoTokenizer, TextStreamer
def formatted_prompt(question)-> str:
return f"<|im_start|>user\n{question}<|im_end|>\n<|im_start|>assistant:"
hub_model_name = "lumatic-ai/BongLlama-1.1B-Chat-alpha-v0"
tokenizer = AutoTokenizer.from_pretrained(hub_model_name)
model = AutoModelForCausalLM.from_pretrained(hub_model_name)
prompt = formatted_prompt('prompt here')
inputs = tokenizer([prompt], return_tensors="pt")
streamer = TextStreamer(tokenizer)
_ = model.generate(**inputs, eos_token_id=[tokenizer.eos_token_id],streamer=streamer, max_new_tokens=256)
Using Generation Config
import torch
from transformers import GenerationConfig
from time import perf_counter
def formatted_prompt(question)-> str:
return f"<|im_start|>user\n{question}<|im_end|>\n<|im_start|>assistant:"
hub_model_name = "lumatic-ai/BongLlama-1.1B-Chat-alpha-v0"
tokenizer = AutoTokenizer.from_pretrained(hub_model_name)
model = AutoModelForCausalLM.from_pretrained(hub_model_name)
prompt = formatted_prompt('হ্যালো')
# Check for GPU availability
if torch.cuda.is_available():
device = "cuda"
else:
device = "cpu"
# Move model and inputs to the GPU (if available)
model.to(device)
inputs = tokenizer(prompt, return_tensors="pt").to(device)
generation_config = GenerationConfig(
penalty_alpha=0.6,
do_sample=True,
top_k=5,
temperature=0.5,
repetition_penalty=1.2,
max_new_tokens=256,
pad_token_id=tokenizer.eos_token_id
)
start_time = perf_counter()
outputs = model.generate(**inputs, generation_config=generation_config)
print(tokenizer.decode(outputs[0], skip_special_tokens=True))
output_time = perf_counter() - start_time
print(f"Time taken for inference: {round(output_time, 2)} seconds")
Training Details
Training Data
we used our dataset of 10k data which consists of Questions and Responses. The dataset name is lumatic-ai/BongChat-v0-10k.
Example Data
- <|im_start|>user এটি কি ইতিবাচক পর্যালোচনা? এই ফ্র্যাঞ্চাইজি কখনও হার্ট স্টপ স্টান্টস, ফাইট সিকোয়েন্স এবং তারকা শক্তি বন্ধ করে হতাশ করে না।এবার মূল চারটি ছাড়াও নতুন মুখগুলি হলেন আবুয়েলিতা চরিত্রে রিতা মোরেনো এবং দান্তে জেসন মোমোয়া যিনি শোটি চুরি করেছেন।<|im_end|> <|im_start|>assistant এটি একটি খুব ইতিবাচক পর্যালোচনা, এটি ইঙ্গিত করে যে ফ্র্যাঞ্চাইজি শ্রোতাদের জন্য আরও পছন্দসই চরিত্রগুলি যুক্ত করার পাশাপাশি অতীতের প্রত্যাশার ভিত্তিতে বিতরণ করেছে।<|im_end|>
Training Procedure
Preprocessing
- Dataset Format <|im_start|>user <question><|im_end|> <|im_start|>assistant <response><|im_end|>
Training hyperparameters
The following hyperparameters were used during training:
- learning_rate: 0.0002
- train_batch_size: 4
- eval_batch_size: 8
- seed: 42
- gradient_accumulation_steps: 2
- total_train_batch_size: 8
- optimizer: Adam with betas=(0.9,0.999) and epsilon=1e-08
- lr_scheduler_type: cosine
- lr_scheduler_warmup_ratio: 0.03
- num_epochs: 3
- mixed_precision_training: Native AMP
Framework versions
- Transformers 4.35.2
- Pytorch 2.1.0+cu121
- Datasets 2.16.1
- Tokenizers 0.15.0
Evaluation
Metrics
- train/loss
- steps
Results
_runtime | _timestamp | train/epoch | train/total_flos | train/train_loss | train/global_step | train/train_steps_per_second | train/loss | train/train_samples_per_second | train/train_runtime | _step | train/learning_rate | |
---|---|---|---|---|---|---|---|---|---|---|---|---|
0 | 205.76071906089783 | 1705483341.4811552 | 0.08 | 100 | 1.2865 | 0 | 0.0001869158878504673 | |||||
1 | 406.9242510795593 | 1705483542.6446872 | 0.17 | 200 | 1.0698 | 1 | 0.00019964245392895794 | |||||
2 | 607.5763952732086 | 1705483743.2968314 | 0.25 | 300 | 1.0457 | 2 | 0.00019846317589644678 | |||||
3 | 808.9941129684448 | 1705483944.714549 | 0.34 | 400 | 1.0131 | 3 | 0.00019646988832610704 | |||||
4 | 1012.7936038970947 | 1705484148.51404 | 0.42 | 500 | 1.0 | 4 | 0.00019367907001906532 | |||||
5 | 1217.8231673240662 | 1705484353.5436034 | 0.51 | 600 | 0.9913 | 5 | 0.0001901137930801933 | |||||
6 | 1422.651272058487 | 1705484558.3717082 | 0.59 | 700 | 0.9904 | 6 | 0.00018580353217762766 | |||||
7 | 1624.9901471138 | 1705484760.7105832 | 0.67 | 800 | 0.9705 | 7 | 0.0001807839208713596 | |||||
8 | 1827.1909170150757 | 1705484962.911353 | 0.76 | 900 | 0.9661 | 8 | 0.00017509645702535999 | |||||
9 | 2033.6470217704773 | 1705485169.3674579 | 0.84 | 1000 | 0.9588 | 9 | 0.00016878815973864268 | |||||
10 | 2241.5517098903656 | 1705485377.272146 | 0.93 | 1100 | 0.9469 | 10 | 0.00016191118063146672 | |||||
11 | 2446.751221895218 | 1705485582.471658 | 1.01 | 1200 | 0.9453 | 11 | 0.0001545223727002313 | |||||
12 | 2648.367230653763 | 1705485784.0876667 | 1.09 | 1300 | 0.9329 | 12 | 0.0001466828203054036 | |||||
13 | 2849.9791855812073 | 1705485985.6996217 | 1.18 | 1400 | 0.9299 | 13 | 0.0001384573341781387 | |||||
14 | 3050.282051086426 | 1705486186.0024872 | 1.26 | 1500 | 0.9181 | 14 | 0.00012991391562044527 | |||||
15 | 3252.6823406219482 | 1705486388.4027767 | 1.35 | 1600 | 0.917 | 15 | 0.00012112319432843371 | |||||
16 | 3456.3907039165497 | 1705486592.11114 | 1.43 | 1700 | 0.919 | 16 | 0.00011215784448624378 | |||||
17 | 3658.387463569641 | 1705486794.1078997 | 1.52 | 1800 | 0.9156 | 17 | 0.00010309198395788984 | |||||
18 | 3860.850716114044 | 1705486996.5711522 | 1.6 | 1900 | 0.9074 | 18 | 9.400056154399221e-05 | |||||
19 | 4063.906144142151 | 1705487199.6265802 | 1.68 | 2000 | 0.9072 | 19 | 8.49587373690336e-05 | |||||
20 | 4266.29203081131 | 1705487402.012467 | 1.77 | 2100 | 0.9061 | 20 | 7.604126152157019e-05 | |||||
21 | 4468.759161949158 | 1705487604.479598 | 1.85 | 2200 | 0.9104 | 21 | 6.732185608427e-05 | |||||
22 | 4671.109050750732 | 1705487806.8294868 | 1.94 | 2300 | 0.9016 | 22 | 5.8872605662626776e-05 | |||||
23 | 4875.181975841522 | 1705488010.902412 | 2.02 | 2400 | 0.8957 | 23 | 5.076336145093832e-05 | |||||
24 | 5077.5954213142395 | 1705488213.3158574 | 2.11 | 2500 | 0.8948 | 24 | 4.3061163762223156e-05 | |||||
25 | 5280.958572149277 | 1705488416.6790082 | 2.19 | 2600 | 0.8833 | 25 | 3.582968779610564e-05 | |||||
26 | 5483.901570320129 | 1705488619.6220064 | 2.27 | 2700 | 0.9019 | 26 | 2.912871722658781e-05 | |||||
27 | 5684.498034954071 | 1705488820.218471 | 2.36 | 2800 | 0.8921 | 27 | 2.30136499616351e-05 | |||||
28 | 5885.339627027512 | 1705489021.0600631 | 2.44 | 2900 | 0.8897 | 28 | 1.753504016053409e-05 | |||||
29 | 6089.49475812912 | 1705489225.2151942 | 2.53 | 3000 | 0.8765 | 29 | 1.2738180295232205e-05 | |||||
30 | 6291.281028032303 | 1705489427.0014641 | 2.61 | 3100 | 0.889 | 30 | 8.662726710819169e-06 | |||||
31 | 6494.627055644989 | 1705489630.3474917 | 2.69 | 3200 | 0.8846 | 31 | 5.342371780697386e-06 | |||||
32 | 6695.168158054352 | 1705489830.8885942 | 2.78 | 3300 | 0.8908 | 32 | 2.804565366782108e-06 | |||||
33 | 6898.186992406845 | 1705490033.9074285 | 2.86 | 3400 | 0.885 | 33 | 1.0702878874610523e-06 | |||||
34 | 7099.970013856888 | 1705490235.69045 | 2.95 | 3500 | 0.8871 | 34 | 1.5387686939386526e-07 | |||||
35 | 7221.330135822296 | 1705490357.050572 | 3.0 | 8.3571998449877e+16 | 0.9397975607756582 | 3561 | 0.491 | 3.926 | 7259.0631 | 35 |
Model Examination
We will be further finetuning this model on large dataset to see how it performs
Environmental Impact
Carbon emissions can be estimated using the Machine Learning Impact calculator presented in Lacoste et al. (2019).
- Hardware Type: 1 X Tesla T4
- Hours used: 2.21
- Cloud Provider: Google Colab
- Compute Region: India
- Carbon Emitted: 0.14
Technical Specifications
Model Architecture and Objective
Finetuned on Tiny-Llama 1.1B Chat model
Hardware
1 X Tesla T4
Citation
BibTeX:
@misc{BongLlama-1.1B-Chat-alpha-v0,
url={[https://huggingface.co/lumatic-ai/BongLlama-1.1B-Chat-alpha-v0](https://huggingface.co/lumatic-ai/BongLlama-1.1B-Chat-alpha-v0)},
title={BongLlama 1.1B Chat Aplha V0},
author={LumaticAI, Rohan Shaw, Vivek Kushal, Jeet Ghosh},
year={2024}, month={Jan}
}
Model Card Authors
lumatic-ai
Model Card Contact
email : [email protected]
- Downloads last month
- 71