Sakura-SOLAR-Instruct
- Model creator: Sanji Watsuki
- Original model: Sonya-7B
- Is a merge of:
Quantizations
I also provide zipped quantization because a lot of people find gguf single download convenient. Zipped quantization is relatively smaller in size to download. After extracted, you can use the model folder as usual.
Parameter Explanation
- BPW: Bits per weight of the quantized model.
- Folder Size: Size of the model folder in GB when stored on disk or after extracted from zip file.
- Zipped Folder Size: Size of the zipped file after downloaded.
- VRAM Usage: VRAM usage of the model measured using ExLlamav2_HF and 4096 max_seq_len with Oobabooga's Text Generation WebUI.
- Loss: Accuracy loss of the quantized model compared to the original model.
- Description: Recommended GPU and/or settings for the model.
On the table, I included VRAM usages of both 4096 max_seq_len and 8192 max_seq_len as a comparison. Sonya-7B default max_seq_len is 8192.
Branch | BPW | Folder Size | Zipped File Size | VRAM Usage (4096/8192) | Loss | Description |
---|---|---|---|---|---|---|
2.0bpw/2.0bpw-zip | 2.0BPW | 2.15GB | 1.80GB | 3.1GB/3.5GB | ~14% | For >=4GB VRAM cards with idle VRAM atleast or below 500MB (headroom for other things) |
2.4bpw/2.4bpw-zip | 2.4BPW | 2.29GB | 1.98GB | 3.3GB/3.7GB | ~13% | For >=4GB VRAM cards (use 4096 max_seq_len) |
3.5bpw/3.5bpw-zip | 3.5BPW | 3.19GB | 2.91GB | 4.2GB/4.6GB | ~7% | For >=5GB VRAM cards (use 4096 max_seq_len) |
4.5bpw (main)/4.5bpw-zip | 4.5BPW | 4.0GB | 3.77GB | 5.0GB/5.3GB | ~4% | For >=6GB VRAM cards with idle VRAM atleast or below 500MB (headroom for other things) |
4.75bpw/4.75bpw-zip | 4.75BPW | 4.2GB | 4.0GB | 5.2GB/5.6GB | ~3% | For >=6GB VRAM cards (use 4096 max_seq_len) |
7.0bpw/7.0bpw-zip | 7.0BPW | 6.03GB | 5.74GB | 7GB/7.3GB | ~1% | For >=8GB VRAM cards with idle VRAM atleast or below 500MB (headroom for other things) |
Unfortunately I couldn't make higher quants than 7.0bpw. No error on the console, just "Killed" after creating output directory for the model.
Calibration Dataset
- Exllamav2 default dataset
Prompt template: Alpaca
Below is an instruction that describes a task. Write a response that appropriately completes the request.
### Instruction:
{prompt}
### Response:
Original Info
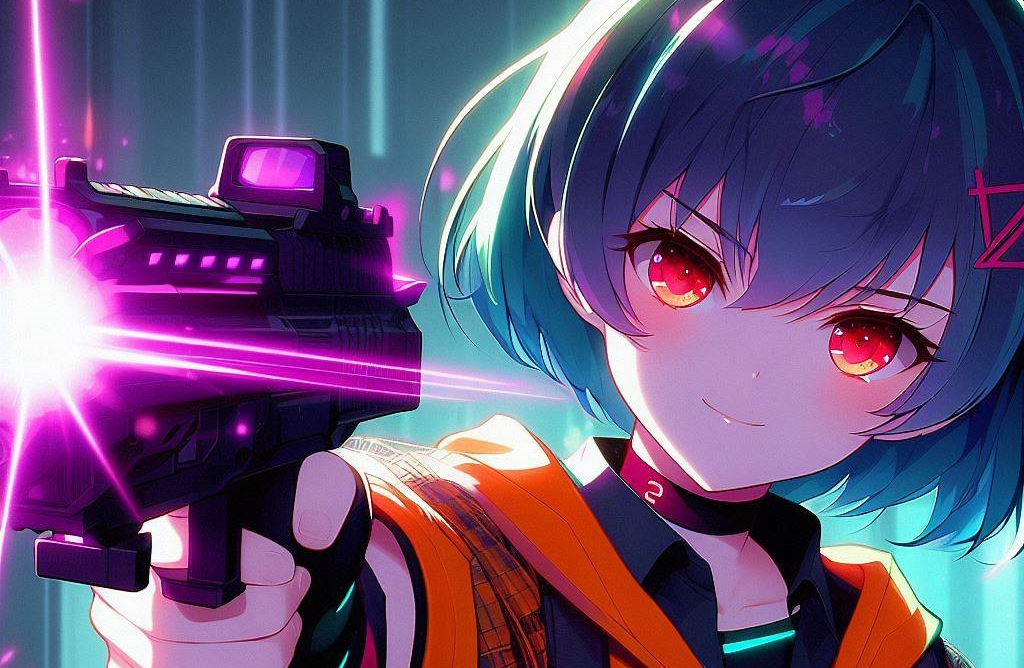
Top 1 Performer MT-bench π€ͺ
WTF is This?
Sonya-7B is, at the time of writing, the #1 performing model in MT-Bench first turn, ahead of GPT-4, and overall the #2 model in MT-Bench, to the best of my knowledge. Sonya-7B should be a good all-purpose model for all tasks including assistant, RP, etc.
Sonya-7B has a similar structure to my previous model, Silicon-Maid-7B, and uses a very similar merge. It's a merge of xDAN-AI/xDAN-L1-Chat-RL-v1, Jan-Ai's Stealth v1.2, chargoddard/piano-medley-7b, NeverSleep/Noromaid-7B-v0.2, and athirdpath/NSFW_DPO_vmgb-7b. Sauce is below. Somehow, by combining these pieces, it substantially outscores any of its parents on MT-Bench.
I picked these models because:
- MT-Bench normally correlates well with real world model quality and xDAN performs well on it.
- Almost all models in the mix were Alpaca prompt formatted which gives prompt consistency.
- Stealth v1.2 has been a magic sprinkle that seems to increase my MT-Bench scores.
- I added RP models because it boosted the Writing and Roleplay benchmarks π
Based on the parent models, I expect this model to be used with an 8192 context window. Please use NTK scaling alpha of 2.6 to experimentally try out 16384 context.
Let me be candid: Despite the test scores, this model is NOT is a GPT killer. I think it's a very sharp model for a 7B, it probably punches way above its weight for a 7B, but it's still a 7B model. Even for a 7B model, I think it's quirky and has some weird outputs, probably due to how Frankenstein this merge is. Keep your expectations in check π
MT-Bench Average Turn
model | score | size |
---|---|---|
gpt-4 | 8.99 | - |
Sonya-7B | 8.52 | 7b |
xDAN-L1-Chat-RL-v1 | 8.34 | 7b |
Starling-7B | 8.09 | 7b |
Claude-2 | 8.06 | - |
Silicon-Maid | 7.96 | 7b |
Loyal-Macaroni-Maid | 7.95 | 7b |
gpt-3.5-turbo | 7.94 | 20b? |
Claude-1 | 7.90 | - |
OpenChat-3.5 | 7.81 | - |
vicuna-33b-v1.3 | 7.12 | 33b |
wizardlm-30b | 7.01 | 30b |
Llama-2-70b-chat | 6.86 | 70b |
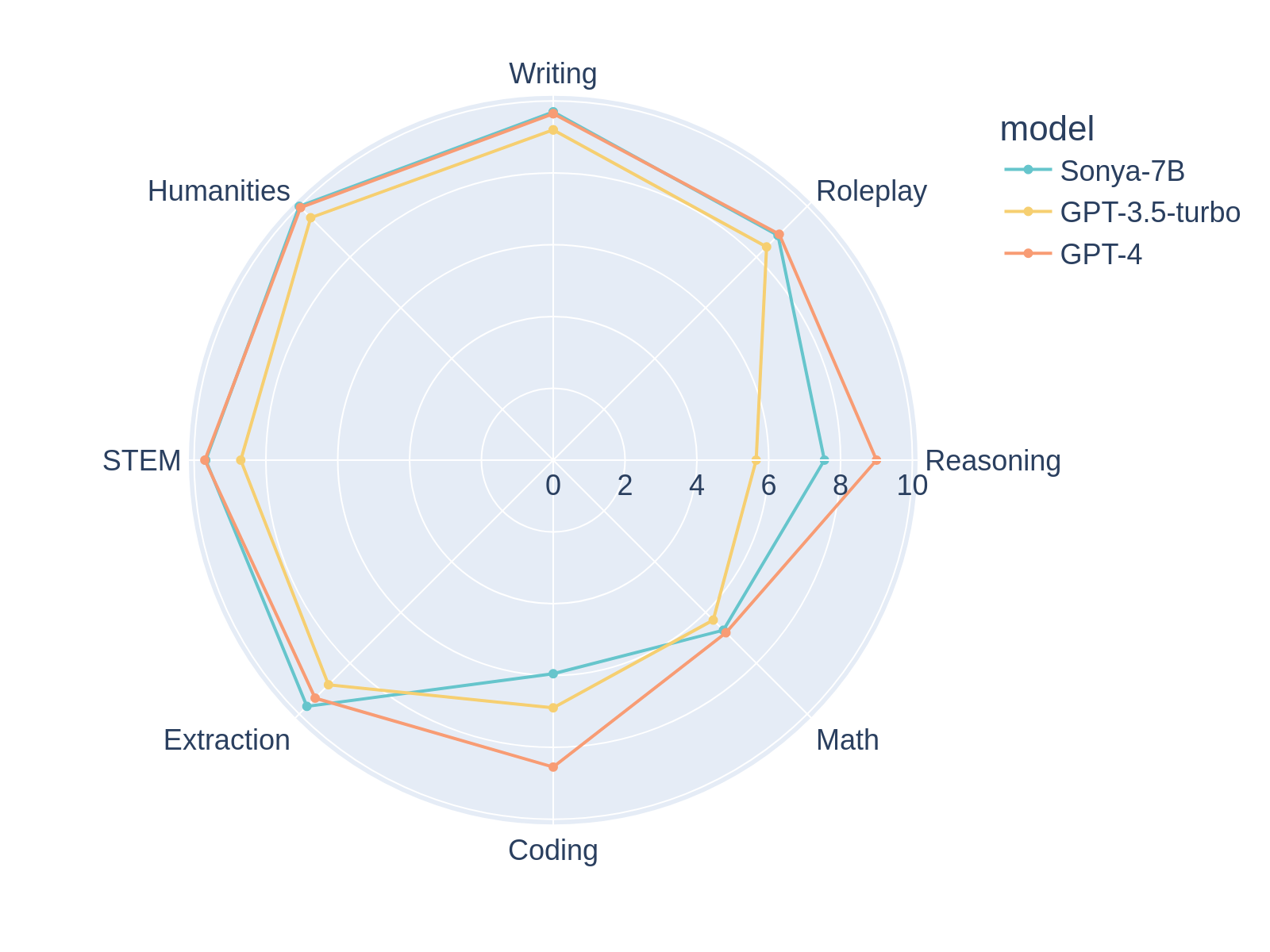
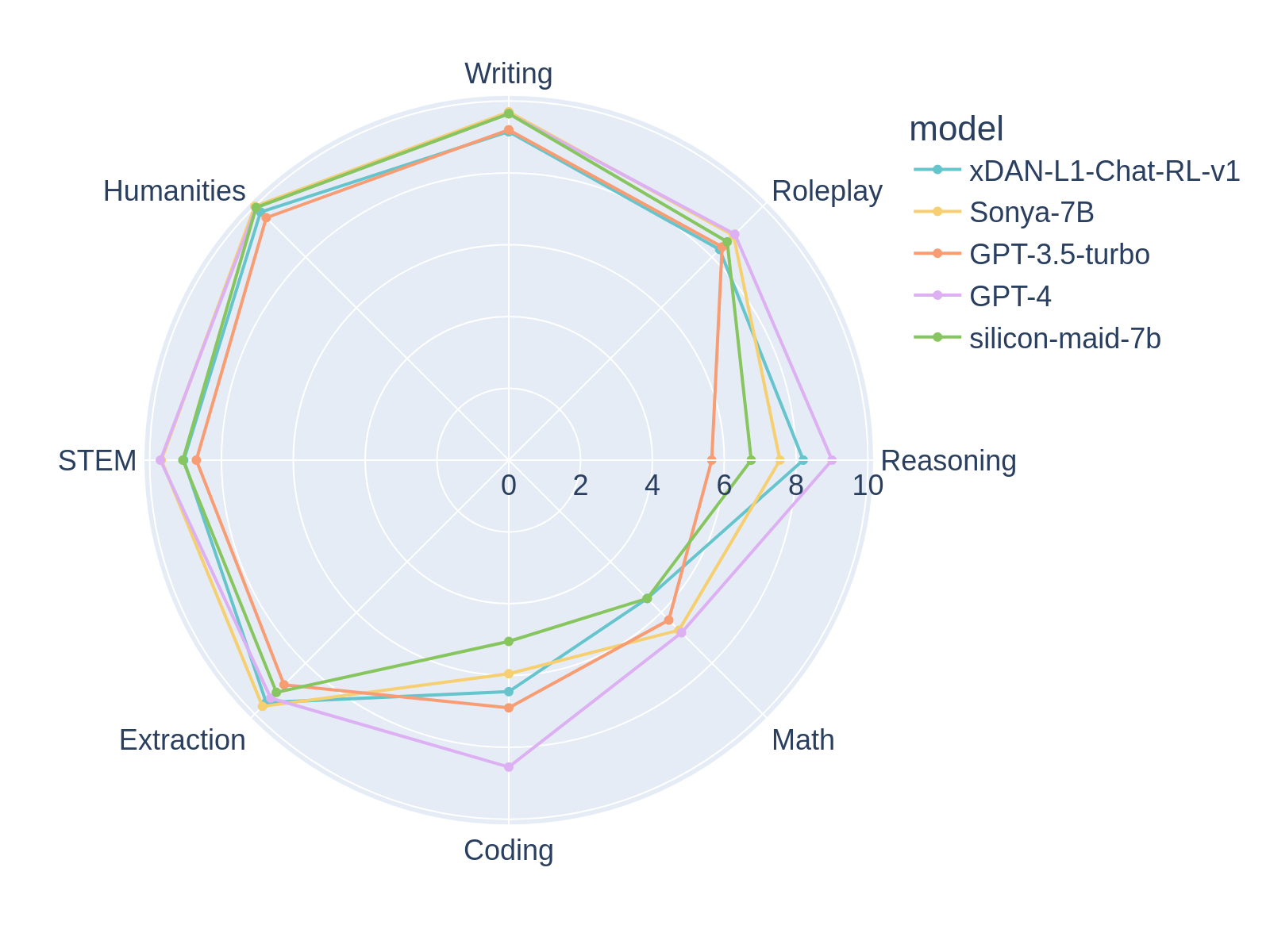
The Sauce
models:
- model: xDAN-AI/xDAN-L1-Chat-RL-v1
parameters:
weight: 1
density: 1
- model: chargoddard/piano-medley-7b
parameters:
weight: 0.3
- model: jan-hq/stealth-v1.2
parameters:
weight: 0.2
- model: NeverSleep/Noromaid-7b-v0.2
parameters:
weight: 0.2
- model: athirdpath/NSFW_DPO_vmgb-7b
parameters:
weight: 0.2
merge_method: ties
base_model: mistralai/Mistral-7B-v0.1
parameters:
density: 0.4
int8_mask: true
normalize: true
dtype: bfloat16
There was no additional training, finetuning, or DPO. This is a straight merger.
Prompt Template (Alpaca)
Below is an instruction that describes a task. Write a response that appropriately completes the request.
### Instruction:
{prompt}
### Response:
I found that this model performed worse with the xDAN prompt format so, despite the heavy weight of xDAN in this merger, I recommeend against its use.
Other Benchmark Stuff
########## First turn ##########
model | turn | score | size |
---|---|---|---|
Sonya-7B | 1 | 9.06875 | 7b |
gpt-4 | 1 | 8.95625 | - |
xDAN-L1-Chat-RL-v1 | 1 | 8.87500 | 7b |
xDAN-L2-Chat-RL-v2 | 1 | 8.78750 | 30b |
claude-v1 | 1 | 8.15000 | - |
gpt-3.5-turbo | 1 | 8.07500 | 20b |
vicuna-33b-v1.3 | 1 | 7.45625 | 33b |
wizardlm-30b | 1 | 7.13125 | 30b |
oasst-sft-7-llama-30b | 1 | 7.10625 | 30b |
Llama-2-70b-chat | 1 | 6.98750 | 70b |
########## Second turn ##########
model | turn | score | size |
---|---|---|---|
gpt-4 | 2 | 9.025000 | - |
xDAN-L2-Chat-RL-v2 | 2 | 8.087500 | 30b |
Sonya-7B | 2 | 7.962500 | 7b |
xDAN-L1-Chat-RL-v1 | 2 | 7.825000 | 7b |
gpt-3.5-turbo | 2 | 7.812500 | 20b |
claude-v1 | 2 | 7.650000 | - |
wizardlm-30b | 2 | 6.887500 | 30b |
vicuna-33b-v1.3 | 2 | 6.787500 | 33b |
Llama-2-70b-chat | 2 | 6.725000 | 70b |
If you'd like to replicate the MT-Bench run, please ensure that the Alpaca prompt template is applied to the model. I did this by putting "alpaca" in the model path to trigger the AlpacaAdapter
.
- Downloads last month
- 5