|
--- |
|
inference: false |
|
license: other |
|
duplicated_from: TheBloke/wizard-vicuna-13B-SuperHOT-8K-fp16 |
|
--- |
|
|
|
<!-- header start --> |
|
<div style="width: 100%;"> |
|
Cloned from TheBloke repo |
|
</div> |
|
<!-- header end --> |
|
|
|
# June Lee's Wizard Vicuna 13B fp16 |
|
|
|
This is fp16 pytorch format model files for [June Lee's Wizard Vicuna 13B](https://huggingface.co/TheBloke/wizard-vicuna-13B-HF) merged with [Kaio Ken's SuperHOT 8K](https://huggingface.co/kaiokendev/superhot-13b-8k-no-rlhf-test). |
|
|
|
[Kaio Ken's SuperHOT 13b LoRA](https://huggingface.co/kaiokendev/superhot-13b-8k-no-rlhf-test) is merged on to the base model, and then 8K context can be achieved during inference by using `trust_remote_code=True`. |
|
|
|
Note that `config.json` has been set to a sequence length of 8192. This can be modified to 4096 if you want to try with a smaller sequence length. |
|
|
|
## Repositories available |
|
|
|
* [4-bit GPTQ models for GPU inference](https://huggingface.co/TheBloke/wizard-vicuna-13B-SuperHOT-8K-GPTQ) |
|
* [2, 3, 4, 5, 6 and 8-bit GGML models for CPU inference](https://huggingface.co/TheBloke/wizard-vicuna-13B-SuperHOT-8K-GGML) |
|
* [Unquantised SuperHOT fp16 model in pytorch format, for GPU inference and for further conversions](https://huggingface.co/TheBloke/wizard-vicuna-13B-SuperHOT-8K-fp16) |
|
* [Unquantised base fp16 model in pytorch format, for GPU inference and for further conversions](https://huggingface.co/junelee/wizard-vicuna-13b) |
|
|
|
## How to use this model from Python code |
|
|
|
First make sure you have Einops installed: |
|
|
|
``` |
|
pip3 install auto-gptq |
|
``` |
|
|
|
Then run the following code. `config.json` has been default to a sequence length of 8192, but you can also configure this in your Python code. |
|
|
|
The provided modelling code, activated with `trust_remote_code=True` will automatically set the `scale` parameter from the configured `max_position_embeddings`. Eg for 8192, `scale` is set to `4`. |
|
|
|
```python |
|
from transformers import AutoConfig, AutoTokenizer, AutoModelForCausalLM, pipeline |
|
import argparse |
|
|
|
model_name_or_path = "gsaivinay/wizard-vicuna-13B-SuperHOT-8K-fp16" |
|
|
|
use_triton = False |
|
|
|
tokenizer = AutoTokenizer.from_pretrained(model_name_or_path, use_fast=True) |
|
|
|
config = AutoConfig.from_pretrained(model_name_or_path, trust_remote_code=True) |
|
# Change this to the sequence length you want |
|
config.max_position_embeddings = 8192 |
|
|
|
model = AutoModelForCausalLM.from_pretrained(model_name_or_path, |
|
config=config, |
|
trust_remote_code=True, |
|
device_map='auto') |
|
|
|
# Note: check to confirm if this is correct prompt template is correct for this model! |
|
prompt = "Tell me about AI" |
|
prompt_template=f'''USER: {prompt} |
|
ASSISTANT:''' |
|
|
|
print("\n\n*** Generate:") |
|
|
|
input_ids = tokenizer(prompt_template, return_tensors='pt').input_ids.cuda() |
|
output = model.generate(inputs=input_ids, temperature=0.7, max_new_tokens=512) |
|
print(tokenizer.decode(output[0])) |
|
|
|
# Inference can also be done using transformers' pipeline |
|
|
|
print("*** Pipeline:") |
|
pipe = pipeline( |
|
"text-generation", |
|
model=model, |
|
tokenizer=tokenizer, |
|
max_new_tokens=512, |
|
temperature=0.7, |
|
top_p=0.95, |
|
repetition_penalty=1.15 |
|
) |
|
|
|
print(pipe(prompt_template)[0]['generated_text']) |
|
``` |
|
|
|
## Using other UIs: monkey patch |
|
|
|
Provided in the repo is `llama_rope_scaled_monkey_patch.py`, written by @kaiokendev. |
|
|
|
It can be theoretically be added to any Python UI or custom code to enable the same result as `trust_remote_code=True`. I have not tested this, and it should be superseded by using `trust_remote_code=True`, but I include it for completeness and for interest. |
|
|
|
|
|
|
|
# Original model card: Kaio Ken's SuperHOT 8K |
|
|
|
### SuperHOT Prototype 2 w/ 8K Context |
|
|
|
This is a second prototype of SuperHOT, this time 30B with 8K context and no RLHF, using the same technique described in [the github blog](https://kaiokendev.github.io/til#extending-context-to-8k). |
|
Tests have shown that the model does indeed leverage the extended context at 8K. |
|
|
|
You will need to **use either the monkeypatch** or, if you are already using the monkeypatch, **change the scaling factor to 0.25 and the maximum sequence length to 8192** |
|
|
|
#### Looking for Merged & Quantized Models? |
|
- 30B 4-bit CUDA: [tmpupload/superhot-30b-8k-4bit-safetensors](https://huggingface.co/tmpupload/superhot-30b-8k-4bit-safetensors) |
|
- 30B 4-bit CUDA 128g: [tmpupload/superhot-30b-8k-4bit-128g-safetensors](https://huggingface.co/tmpupload/superhot-30b-8k-4bit-128g-safetensors) |
|
|
|
|
|
#### Training Details |
|
I trained the LoRA with the following configuration: |
|
- 1200 samples (~400 samples over 2048 sequence length) |
|
- learning rate of 3e-4 |
|
- 3 epochs |
|
- The exported modules are: |
|
- q_proj |
|
- k_proj |
|
- v_proj |
|
- o_proj |
|
- no bias |
|
- Rank = 4 |
|
- Alpha = 8 |
|
- no dropout |
|
- weight decay of 0.1 |
|
- AdamW beta1 of 0.9 and beta2 0.99, epsilon of 1e-5 |
|
- Trained on 4-bit base model |
|
|
|
# Original model card: June Lee's Wizard Vicuna 13B |
|
|
|
# Wizard-Vicuna-13B-HF |
|
|
|
This is a float16 HF format repo for [junelee's wizard-vicuna 13B](https://huggingface.co/junelee/wizard-vicuna-13b). |
|
|
|
June Lee's repo was also HF format. The reason I've made this is that the original repo was in float32, meaning it required 52GB disk space, VRAM and RAM. |
|
|
|
This model was converted to float16 to make it easier to load and manage. |
|
|
|
## Repositories available |
|
|
|
* [4bit GPTQ models for GPU inference](https://huggingface.co/TheBloke/wizard-vicuna-13B-GPTQ). |
|
* [4bit and 5bit GGML models for CPU inference](https://huggingface.co/TheBloke/wizard-vicuna-13B-GGML). |
|
* [float16 HF format model for GPU inference](https://huggingface.co/TheBloke/wizard-vicuna-13B-HF). |
|
|
|
|
|
|
|
# Original WizardVicuna-13B model card |
|
|
|
Github page: https://github.com/melodysdreamj/WizardVicunaLM |
|
|
|
# WizardVicunaLM |
|
### Wizard's dataset + ChatGPT's conversation extension + Vicuna's tuning method |
|
I am a big fan of the ideas behind WizardLM and VicunaLM. I particularly like the idea of WizardLM handling the dataset itself more deeply and broadly, as well as VicunaLM overcoming the limitations of single-turn conversations by introducing multi-round conversations. As a result, I combined these two ideas to create WizardVicunaLM. This project is highly experimental and designed for proof of concept, not for actual usage. |
|
|
|
|
|
## Benchmark |
|
### Approximately 7% performance improvement over VicunaLM |
|
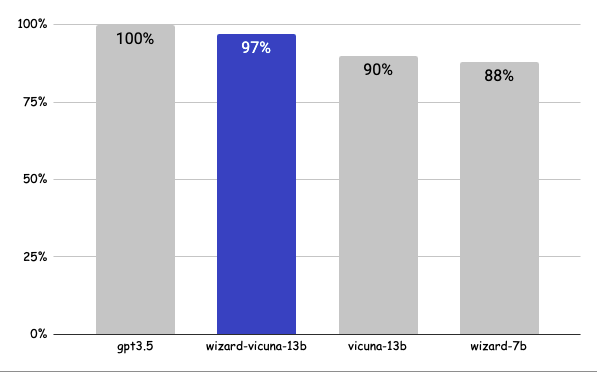 |
|
|
|
|
|
### Detail |
|
|
|
The questions presented here are not from rigorous tests, but rather, I asked a few questions and requested GPT-4 to score them. The models compared were ChatGPT 3.5, WizardVicunaLM, VicunaLM, and WizardLM, in that order. |
|
|
|
| | gpt3.5 | wizard-vicuna-13b | vicuna-13b | wizard-7b | link | |
|
|-----|--------|-------------------|------------|-----------|----------| |
|
| Q1 | 95 | 90 | 85 | 88 | [link](https://sharegpt.com/c/YdhIlby) | |
|
| Q2 | 95 | 97 | 90 | 89 | [link](https://sharegpt.com/c/YOqOV4g) | |
|
| Q3 | 85 | 90 | 80 | 65 | [link](https://sharegpt.com/c/uDmrcL9) | |
|
| Q4 | 90 | 85 | 80 | 75 | [link](https://sharegpt.com/c/XBbK5MZ) | |
|
| Q5 | 90 | 85 | 80 | 75 | [link](https://sharegpt.com/c/AQ5tgQX) | |
|
| Q6 | 92 | 85 | 87 | 88 | [link](https://sharegpt.com/c/eVYwfIr) | |
|
| Q7 | 95 | 90 | 85 | 92 | [link](https://sharegpt.com/c/Kqyeub4) | |
|
| Q8 | 90 | 85 | 75 | 70 | [link](https://sharegpt.com/c/M0gIjMF) | |
|
| Q9 | 92 | 85 | 70 | 60 | [link](https://sharegpt.com/c/fOvMtQt) | |
|
| Q10 | 90 | 80 | 75 | 85 | [link](https://sharegpt.com/c/YYiCaUz) | |
|
| Q11 | 90 | 85 | 75 | 65 | [link](https://sharegpt.com/c/HMkKKGU) | |
|
| Q12 | 85 | 90 | 80 | 88 | [link](https://sharegpt.com/c/XbW6jgB) | |
|
| Q13 | 90 | 95 | 88 | 85 | [link](https://sharegpt.com/c/JXZb7y6) | |
|
| Q14 | 94 | 89 | 90 | 91 | [link](https://sharegpt.com/c/cTXH4IS) | |
|
| Q15 | 90 | 85 | 88 | 87 | [link](https://sharegpt.com/c/GZiM0Yt) | |
|
| | 91 | 88 | 82 | 80 | | |
|
|
|
|
|
## Principle |
|
|
|
We adopted the approach of WizardLM, which is to extend a single problem more in-depth. However, instead of using individual instructions, we expanded it using Vicuna's conversation format and applied Vicuna's fine-tuning techniques. |
|
|
|
Turning a single command into a rich conversation is what we've done [here](https://sharegpt.com/c/6cmxqq0). |
|
|
|
After creating the training data, I later trained it according to the Vicuna v1.1 [training method](https://github.com/lm-sys/FastChat/blob/main/scripts/train_vicuna_13b.sh). |
|
|
|
|
|
## Detailed Method |
|
|
|
First, we explore and expand various areas in the same topic using the 7K conversations created by WizardLM. However, we made it in a continuous conversation format instead of the instruction format. That is, it starts with WizardLM's instruction, and then expands into various areas in one conversation using ChatGPT 3.5. |
|
|
|
After that, we applied the following model using Vicuna's fine-tuning format. |
|
|
|
## Training Process |
|
|
|
Trained with 8 A100 GPUs for 35 hours. |
|
|
|
## Weights |
|
You can see the [dataset](https://huggingface.co/datasets/junelee/wizard_vicuna_70k) we used for training and the [13b model](https://huggingface.co/junelee/wizard-vicuna-13b) in the huggingface. |
|
|
|
## Conclusion |
|
If we extend the conversation to gpt4 32K, we can expect a dramatic improvement, as we can generate 8x more, more accurate and richer conversations. |
|
|
|
## License |
|
The model is licensed under the LLaMA model, and the dataset is licensed under the terms of OpenAI because it uses ChatGPT. Everything else is free. |
|
|
|
## Author |
|
|
|
[JUNE LEE](https://github.com/melodysdreamj) - He is active in Songdo Artificial Intelligence Study and GDG Songdo. |
|
|