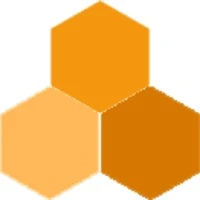
Datasets:
image_id
int64 0
1.48k
| image
imagewidth (px) 1.28k
1.28k
| bounding_box
sequencelengths 4
4
| label
stringclasses 1
value | height
int64 1.28k
1.28k
| width
int64 1.28k
1.28k
|
---|---|---|---|---|---|
0 | [
869,
673,
146.45,
100.29
] | waste | 1,280 | 1,280 |
|
1 | [
571,
45,
115.94,
95.39
] | waste | 1,280 | 1,280 |
|
1 | [
803,
583,
113.6,
134.44
] | waste | 1,280 | 1,280 |
|
2 | [
836,
594,
133.77,
99.06
] | waste | 1,280 | 1,280 |
|
2 | [
223,
251,
103.17,
344.62
] | waste | 1,280 | 1,280 |
|
2 | [
673,
0,
84.47,
162.1
] | waste | 1,280 | 1,280 |
|
2 | [
116,
316,
83.8,
220.99
] | waste | 1,280 | 1,280 |
|
2 | [
428,
552,
177.24,
76.24
] | waste | 1,280 | 1,280 |
|
2 | [
12,
0,
155.09,
287.91
] | waste | 1,280 | 1,280 |
|
2 | [
1030,
376,
110.37,
119.05
] | waste | 1,280 | 1,280 |
|
3 | [
769,
370,
96.37,
59.02
] | waste | 1,280 | 1,280 |
|
3 | [
615,
403,
101.81,
68.02
] | waste | 1,280 | 1,280 |
|
4 | [
247,
0,
138.11,
132.31
] | waste | 1,280 | 1,280 |
|
4 | [
190,
158,
126.41,
195.8
] | waste | 1,280 | 1,280 |
|
4 | [
112,
260,
76.43,
95.97
] | waste | 1,280 | 1,280 |
|
4 | [
118,
394,
113.64,
83.87
] | waste | 1,280 | 1,280 |
|
5 | [
313,
672,
112.08,
199.06
] | waste | 1,280 | 1,280 |
|
5 | [
516,
797,
81.62,
76.98
] | waste | 1,280 | 1,280 |
|
5 | [
528,
1000,
86.67,
126.52
] | waste | 1,280 | 1,280 |
|
5 | [
271,
926,
82.98,
64.22
] | waste | 1,280 | 1,280 |
|
5 | [
624,
555,
143.06,
125.27
] | waste | 1,280 | 1,280 |
|
5 | [
550,
246,
45.4,
272.92
] | waste | 1,280 | 1,280 |
|
5 | [
749,
687,
60.11,
61.33
] | waste | 1,280 | 1,280 |
|
5 | [
428,
674,
61.73,
98.28
] | waste | 1,280 | 1,280 |
|
5 | [
537,
86,
63.4,
72
] | waste | 1,280 | 1,280 |
|
5 | [
308,
163,
239.52,
369.62
] | waste | 1,280 | 1,280 |
|
5 | [
282,
544,
280.23,
125.54
] | waste | 1,280 | 1,280 |
|
5 | [
403,
9,
128.29,
142.26
] | waste | 1,280 | 1,280 |
|
5 | [
595,
684,
75.99,
81.17
] | waste | 1,280 | 1,280 |
|
6 | [
436,
931,
219.05,
250.52
] | waste | 1,280 | 1,280 |
|
6 | [
400,
293,
129.47,
237.69
] | waste | 1,280 | 1,280 |
|
6 | [
866,
965,
54.28,
60.46
] | waste | 1,280 | 1,280 |
|
6 | [
525,
24,
193.41,
260.83
] | waste | 1,280 | 1,280 |
|
6 | [
777,
1096,
49.45,
107.3
] | waste | 1,280 | 1,280 |
|
6 | [
182,
500,
209.23,
113.84
] | waste | 1,280 | 1,280 |
|
6 | [
297,
619,
163.62,
134.7
] | waste | 1,280 | 1,280 |
|
6 | [
888,
201,
195.46,
108.49
] | waste | 1,280 | 1,280 |
|
6 | [
855,
98,
104.73,
92.32
] | waste | 1,280 | 1,280 |
|
6 | [
962,
11,
207.16,
97.11
] | waste | 1,280 | 1,280 |
|
6 | [
1211,
70,
69.31,
100.35
] | waste | 1,280 | 1,280 |
|
6 | [
791,
2,
119.11,
47.01
] | waste | 1,280 | 1,280 |
|
6 | [
947,
314,
83.62,
131.31
] | waste | 1,280 | 1,280 |
|
6 | [
1205,
213,
74.78,
121.69
] | waste | 1,280 | 1,280 |
|
7 | [
869,
355,
181.95,
280.78
] | waste | 1,280 | 1,280 |
|
7 | [
87,
238,
127.8,
142.33
] | waste | 1,280 | 1,280 |
|
7 | [
376,
962,
175.46,
149.21
] | waste | 1,280 | 1,280 |
|
7 | [
357,
502,
50.59,
63.08
] | waste | 1,280 | 1,280 |
|
7 | [
727,
752,
125.62,
86.64
] | waste | 1,280 | 1,280 |
|
7 | [
741,
379,
123.51,
134.95
] | waste | 1,280 | 1,280 |
|
7 | [
732,
642,
110.03,
102.96
] | waste | 1,280 | 1,280 |
|
7 | [
9,
1192,
153.1,
87.79
] | waste | 1,280 | 1,280 |
|
7 | [
196,
613,
126.87,
181.92
] | waste | 1,280 | 1,280 |
|
7 | [
0,
906,
171.69,
182.98
] | waste | 1,280 | 1,280 |
|
7 | [
0,
635,
195.53,
264.12
] | waste | 1,280 | 1,280 |
|
7 | [
200,
398,
100.58,
99.26
] | waste | 1,280 | 1,280 |
|
7 | [
438,
734,
146.77,
109.51
] | waste | 1,280 | 1,280 |
|
7 | [
983,
641,
192.45,
169.55
] | waste | 1,280 | 1,280 |
|
7 | [
403,
549,
323.25,
184.46
] | waste | 1,280 | 1,280 |
|
7 | [
225,
1148,
291.73,
132.43
] | waste | 1,280 | 1,280 |
|
7 | [
742,
841,
537.45,
439.39
] | waste | 1,280 | 1,280 |
|
7 | [
204,
808,
162.59,
206.91
] | waste | 1,280 | 1,280 |
|
7 | [
541,
1189,
198.42,
90.67
] | waste | 1,280 | 1,280 |
|
7 | [
2,
315,
79.17,
88.45
] | waste | 1,280 | 1,280 |
|
8 | [
206,
870,
71,
64
] | waste | 1,280 | 1,280 |
|
8 | [
86,
679,
162,
133
] | waste | 1,280 | 1,280 |
|
8 | [
71,
950,
109.76,
56.78
] | waste | 1,280 | 1,280 |
|
9 | [
113,
202,
104.86,
137.23
] | waste | 1,280 | 1,280 |
|
9 | [
148,
341,
81.51,
108.05
] | waste | 1,280 | 1,280 |
|
9 | [
235,
334,
82.34,
98.76
] | waste | 1,280 | 1,280 |
|
9 | [
323,
338,
79.49,
100.61
] | waste | 1,280 | 1,280 |
|
9 | [
402,
389,
72.34,
91.7
] | waste | 1,280 | 1,280 |
|
9 | [
574,
385,
101.23,
104.64
] | waste | 1,280 | 1,280 |
|
9 | [
757,
445,
184.48,
107.1
] | waste | 1,280 | 1,280 |
|
9 | [
677,
497,
77.5,
49.2
] | waste | 1,280 | 1,280 |
|
9 | [
339,
846,
129.34,
120.16
] | waste | 1,280 | 1,280 |
|
9 | [
79,
648,
65.5,
51.13
] | waste | 1,280 | 1,280 |
|
9 | [
440,
543,
83.87,
96.01
] | waste | 1,280 | 1,280 |
|
9 | [
356,
190,
73.77,
73.24
] | waste | 1,280 | 1,280 |
|
9 | [
244,
787,
47.11,
107.96
] | waste | 1,280 | 1,280 |
|
9 | [
37,
563,
41.68,
54.38
] | waste | 1,280 | 1,280 |
|
10 | [
0,
880,
130.44,
168.87
] | waste | 1,280 | 1,280 |
|
11 | [
431,
831,
353.93,
294.46
] | waste | 1,280 | 1,280 |
|
11 | [
182,
545,
249.53,
263.07
] | waste | 1,280 | 1,280 |
|
11 | [
1056,
0,
219.9,
204.32
] | waste | 1,280 | 1,280 |
|
11 | [
956,
300,
321.32,
262.42
] | waste | 1,280 | 1,280 |
|
11 | [
1187,
1008,
92.87,
200.45
] | waste | 1,280 | 1,280 |
|
11 | [
406,
308,
240.25,
237.75
] | waste | 1,280 | 1,280 |
|
11 | [
0,
776,
87.46,
109.26
] | waste | 1,280 | 1,280 |
|
11 | [
134,
924,
278.05,
139.36
] | waste | 1,280 | 1,280 |
|
11 | [
97,
818,
121.62,
95.6
] | waste | 1,280 | 1,280 |
|
11 | [
1101,
574,
179.09,
202.86
] | waste | 1,280 | 1,280 |
|
11 | [
1134,
791,
145.76,
209.1
] | waste | 1,280 | 1,280 |
|
11 | [
798,
688,
247.25,
268.77
] | waste | 1,280 | 1,280 |
|
11 | [
601,
554,
160.19,
268.66
] | waste | 1,280 | 1,280 |
|
11 | [
808,
981,
246.95,
266.57
] | waste | 1,280 | 1,280 |
|
11 | [
684,
1,
274.48,
182.46
] | waste | 1,280 | 1,280 |
|
11 | [
658,
204,
284.17,
340.62
] | waste | 1,280 | 1,280 |
|
12 | [
1192,
750,
84.27,
60.02
] | waste | 1,280 | 1,280 |
|
12 | [
1132,
663,
142.84,
84.08
] | waste | 1,280 | 1,280 |
|
12 | [
1032,
1169,
64.67,
92.38
] | waste | 1,280 | 1,280 |
Dataset for Waste/Dumpsite Detection using drone imagery
Contains 2115 drone images of illegal waste dumpsites
- 1280 x 1280 px resolution
- Nadir perspective (camera pointing straight down at a 90-degree angle to the ground)
Annotations and Images
train | valid | test
- actual images
COCO - annotations_coco.json files in each split directory
.parquet files in data directory with embeded images
The dataset was collected as part of the [ Raven Scan ] project, more details bellow
Platform Overview
The Raven Scan platform leverages advanced drone and satellite imagery to enhance waste management and environmental monitoring through cutting-edge technology.
Utilizing high-resolution images combined with sophisticated image annotation, object detection models, and geospatial analysis, our system offers robust tools to identify illegal dump sites and effectively manage regulated landfills.
User Guides and Documentation
Guides
Explore each feature through our User Guides
Documentation Page
Read our official Documentation
Key Features
Dataset Management
- Manage extensive datasets of drone and satellite images with tools for uploading, categorizing, and maintaining image data.
- Features include tagging, filtering, and robust data integrity checks to ensure dataset accuracy and usability for environmental monitoring tasks.
Image Annotation
- Annotate high-resolution drone and satellite imagery to help train object detection models specifically designed for precise waste detection.
Object Detection Model Training
- Train sophisticated models with diverse image datasets from drone and satellite sources to enhance detection accuracy across varied environmental conditions.
Detection and Monitoring
- Deploy models, both pre-trained and newly trained, to detect waste sites from aerial perspectives.
- Results are displayed on a georeferenced map, providing a clear and actionable visual representation.
Landfill Management
- Advanced tools for managing legal landfills include the submission of waste forms, types, trucks, reports ...
- Integration of 3D point cloud scans derived from drone technology for detailed, real-time monitoring.
Learn more from our detailed Feature Documentation
This repository aims to equip researchers, environmental agencies, and policymakers with the tools needed to monitor and respond to environmental challenges efficiently.
Join us in leveraging these capabilities to maintain ecological integrity and promote sustainable practices in waste management.
Our complete Project Charter.
Acknowledgments
We would like to extend our deepest gratitude to the following organizations and platforms for their invaluable support
UNICEF Venture Fund
We express our profound gratitude to the UNICEF Venture Fund for their generous support of our project. Their commitment to fostering innovation and sponsoring projects that utilize frontier technology is truly commendable and instrumental in driving positive change.
MMDetection
A special thanks to the open-source AI training platform MMDetection. Your robust tools and extensive resources have significantly accelerated our development process.
Third Party Notices
Our project would not have been possible without the myriad of libraries and frameworks that have empowered us along the way. We owe a great debt of gratitude to all the contributors and maintainers of these projects.
Thank you to everyone who has made this project possible. We couldn't have done it without you!
Raven Scan uses third-party libraries or other resources that may be distributed under licenses different than the Raven Scan software.
In the event that we accidentally failed to list a required notice, please bring it to our attention by posting an issue on out GitHub Page.
Each team member has played a pivotal role in bringing this project to fruition, and we are immensely thankful for their hard work and dedication.
- Downloads last month
- 229
Models trained or fine-tuned on INS-IntelligentNetworkSolutions/Waste-Dumpsites-DroneImagery
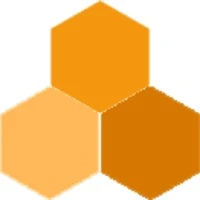