InternVL-Chat-V1-1
[π GitHub] [π InternVL 1.0] [π InternVL 1.5] [π Mini-InternVL] [π InternVL 2.5]
[π Blog] [π¨οΈ Chat Demo] [π€ HF Demo] [π Quick Start] [π Documents]
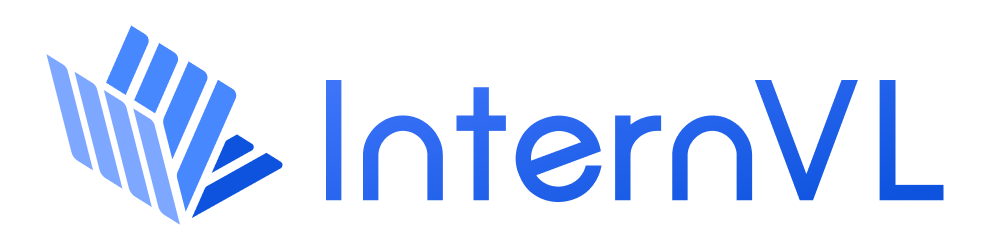
Introduction
We released π€ InternVL-Chat-V1-1, featuring a structure similar to LLaVA, including a ViT, an MLP projector, and an LLM. As shown in the figure below, we connected our InternViT-6B to LLaMA2-13B through a simple MLP projector. Note that the LLaMA2-13B used here is not the original model but an internal chat version obtained by incrementally pre-training and fine-tuning the LLaMA2-13B base model for Chinese language tasks. Overall, our model has a total of 19 billion parameters.
In this version, we explored increasing the resolution to 448 Γ 448, enhancing OCR capabilities, and improving support for Chinese conversations. Since the 448 Γ 448 input image generates 1024 visual tokens after passing through the ViT, leading to a significant computational burden, we use a pixel shuffle (unshuffle) operation to reduce the 1024 tokens to 256 tokens.
For more detailed information about this model, please read our blog.
Examples
In this update, InternVL-Chat has improved support for Chinese and OCR.
As you can see, although the Lynyrd Skynyrd in the image has some letters that are out of the camera's lens, and TOUR's T is blocked, the model is still able to recognize it correctly.
This model can also conduct an in-depth analysis of AAAI's official website and identify important information on the web page.
Model Details
Model Type: multimodal large language model (MLLM)
Model Stats:
- Architecture: InternViT-6B-448px + MLP + LLaMA2-13B (Our internal SFT versions)
- Image size: 448 x 448 (256 tokens)
- Params: 19B
Training Strategy:
- Pre-training Stage
- Learnable Component: ViT + MLP
- Data: Trained on 72M samples, including COYO, LAION, CC12M, CC3M, SBU, Wukong, GRIT, Objects365, OpenImages, and OCR-related datasets.
- Note: In this stage, we load the pretrained weights of the original InternViT-6B-224px and interpolate its position embedding to the size corresponding to 448 x 448 pixels. Moreover, in order to reduce the number of visual tokens, we use a pixel shuffle (unshuffle) operation to reduce 1024 tokens to 256 tokens.
- Supervised Fine-tuning Stage
- Learnable Component: MLP + LLM
- Data: A comprehensive collection of open-source datasets, along with their Chinese translation versions, totaling approximately 6M samples.
- Pre-training Stage
Performance
model | LLaVA-1.5 | InternVL-Chat -V1-0 |
InternVL-Chat -V1-0 |
InternVL-Chat -V1-1 |
---|---|---|---|---|
resolution | 336 | 336 | 448 | 448 |
vision encoder | CLIP-L-336px | InternViT-6B-224px | InternViT-6B-448px | InternViT-6B-448px |
language model | Vicuna-13B | Vicuna-13B | Vicuna-13B | LLaMA2-13B |
VQAv2testdev | 80.0 | 80.2 | 82.0 | 80.9 |
GQAtestdev | 63.3 | 63.9 | 64.1 | 62.5 |
VizWiztest | 53.6 | 54.6 | 60.1 | 57.3 |
SQAtest | 71.6 | 70.1 | 71.6 | 90.1 |
TextVQAval, w/o OCR | - | - | - | 64.2 |
TextVQAval, w/ OCR | 61.3 | 58.7 | 64.8 | 68.6 |
POPE | 85.9 | 87.1 | 87.2 | 87.1 |
MMEperception | 1531.3 | 1546.9 | 1579.0 | 1659.8 |
MMB-ENtest | 67.7 | 66.5 | 68.2 | 75.4 |
MMB-CNtest | 63.6 | 61.9 | 64.0 | 70.3 |
MMVetGPT-4-0613 | 35.4 | 33.7 | 36.7 | 46.7 |
- Note that we use the official evaluation server to test the MMVet scores, with
GPT-4-0613
serving as the judge model. Using different versions of GPT-4 as the judge can result in significant score variations.
Quick Start
We provide an example code to run InternVL-Chat-V1-1 using transformers
.
Please use transformers>=4.37.2 to ensure the model works normally.
Model Loading
16-bit (bf16 / fp16)
import torch
from transformers import AutoTokenizer, AutoModel
path = "OpenGVLab/InternVL-Chat-V1-1"
model = AutoModel.from_pretrained(
path,
torch_dtype=torch.bfloat16,
low_cpu_mem_usage=True,
use_flash_attn=True,
trust_remote_code=True).eval().cuda()
BNB 8-bit Quantization
import torch
from transformers import AutoTokenizer, AutoModel
path = "OpenGVLab/InternVL-Chat-V1-1"
model = AutoModel.from_pretrained(
path,
torch_dtype=torch.bfloat16,
load_in_8bit=True,
low_cpu_mem_usage=True,
use_flash_attn=True,
trust_remote_code=True).eval()
BNB 4-bit Quantization
β οΈ Warning: Due to significant quantization errors with BNB 4-bit quantization on InternViT-6B, the model may produce nonsensical outputs and fail to understand images. Therefore, please avoid using BNB 4-bit quantization.
Multiple GPUs
The reason for writing the code this way is to avoid errors that occur during multi-GPU inference due to tensors not being on the same device. By ensuring that the first and last layers of the large language model (LLM) are on the same device, we prevent such errors.
import math
import torch
from transformers import AutoTokenizer, AutoModel
def split_model(model_name):
device_map = {}
world_size = torch.cuda.device_count()
num_layers = {'InternVL-Chat-V1-1': 40}[model_name]
# Since the first GPU will be used for ViT, treat it as half a GPU.
num_layers_per_gpu = math.ceil(num_layers / (world_size - 0.5))
num_layers_per_gpu = [num_layers_per_gpu] * world_size
num_layers_per_gpu[0] = math.ceil(num_layers_per_gpu[0] * 0.5)
layer_cnt = 0
for i, num_layer in enumerate(num_layers_per_gpu):
for j in range(num_layer):
device_map[f'language_model.model.layers.{layer_cnt}'] = i
layer_cnt += 1
device_map['vision_model'] = 0
device_map['mlp1'] = 0
device_map['language_model.model.tok_embeddings'] = 0
device_map['language_model.model.embed_tokens'] = 0
device_map['language_model.output'] = 0
device_map['language_model.model.norm'] = 0
device_map['language_model.lm_head'] = 0
device_map[f'language_model.model.layers.{num_layers - 1}'] = 0
return device_map
path = "OpenGVLab/InternVL-Chat-V1-1"
device_map = split_model('InternVL-Chat-V1-1')
model = AutoModel.from_pretrained(
path,
torch_dtype=torch.bfloat16,
low_cpu_mem_usage=True,
use_flash_attn=True,
trust_remote_code=True,
device_map=device_map).eval()
Inference with Transformers
Pure-text conversation
from transformers import AutoTokenizer, AutoModel
import torch
path = "OpenGVLab/InternVL-Chat-V1-1"
model = AutoModel.from_pretrained(
path,
torch_dtype=torch.bfloat16,
low_cpu_mem_usage=True,
use_flash_attn=True,
trust_remote_code=True).eval().cuda()
tokenizer = AutoTokenizer.from_pretrained(path, trust_remote_code=True, use_fast=False)
generation_config = dict(max_new_tokens=1024, do_sample=True)
question = 'Hello, who are you?'
response, history = model.chat(tokenizer, None, question, generation_config, history=None, return_history=True)
print(f'User: {question}')
print(f'Assistant: {response}')
question = 'Can you tell me a story?'
response, history = model.chat(tokenizer, None, question, generation_config, history=history, return_history=True)
print(f'User: {question}')
print(f'Assistant: {response}')
Single-image single-round conversation
from transformers import AutoTokenizer, AutoModel, CLIPImageProcessor
from PIL import Image
import torch
path = "OpenGVLab/InternVL-Chat-V1-1"
model = AutoModel.from_pretrained(
path,
torch_dtype=torch.bfloat16,
low_cpu_mem_usage=True,
use_flash_attn=True,
trust_remote_code=True).eval().cuda()
tokenizer = AutoTokenizer.from_pretrained(path, trust_remote_code=True, use_fast=False)
image_processor = CLIPImageProcessor.from_pretrained(path)
image = Image.open('./examples/image2.jpg').resize((448, 448))
pixel_values = image_processor(images=image, return_tensors='pt').pixel_values.to(torch.bfloat16).cuda()
generation_config = dict(max_new_tokens=1024, do_sample=True)
question = '<image>\nPlease describe the image shortly.'
response = model.chat(tokenizer, pixel_values, question, generation_config)
print(f'User: {question}')
print(f'Assistant: {response}')
Single-image multi-round conversation
from transformers import AutoTokenizer, AutoModel, CLIPImageProcessor
from PIL import Image
import torch
path = "OpenGVLab/InternVL-Chat-V1-1"
model = AutoModel.from_pretrained(
path,
torch_dtype=torch.bfloat16,
low_cpu_mem_usage=True,
use_flash_attn=True,
trust_remote_code=True).eval().cuda()
tokenizer = AutoTokenizer.from_pretrained(path, trust_remote_code=True, use_fast=False)
image_processor = CLIPImageProcessor.from_pretrained(path)
image = Image.open('./examples/image2.jpg').resize((448, 448))
pixel_values = image_processor(images=image, return_tensors='pt').pixel_values.to(torch.bfloat16).cuda()
generation_config = dict(max_new_tokens=1024, do_sample=True)
question = '<image>\nPlease describe the image in detail.'
response, history = model.chat(tokenizer, pixel_values, question, generation_config, history=None, return_history=True)
print(f'User: {question}')
print(f'Assistant: {response}')
question = 'Please write a poem according to the image.'
response, history = model.chat(tokenizer, pixel_values, question, generation_config, history=history, return_history=True)
print(f'User: {question}')
print(f'Assistant: {response}')
Multi-image multi-round conversation, combined images
β οΈοΈ Warning: Please note that for this model, we support multi-image chat in the interface, but the results are not very good due to the lack of training with multi-image data.
from transformers import AutoTokenizer, AutoModel, CLIPImageProcessor
from PIL import Image
import torch
path = "OpenGVLab/InternVL-Chat-V1-1"
model = AutoModel.from_pretrained(
path,
torch_dtype=torch.bfloat16,
low_cpu_mem_usage=True,
use_flash_attn=True,
trust_remote_code=True).eval().cuda()
tokenizer = AutoTokenizer.from_pretrained(path, trust_remote_code=True, use_fast=False)
image_processor = CLIPImageProcessor.from_pretrained(path)
image1 = Image.open('./examples/image1.jpg').resize((448, 448))
pixel_values1 = image_processor(images=image1, return_tensors='pt').pixel_values.to(torch.bfloat16).cuda()
image2 = Image.open('./examples/image2.jpg').resize((448, 448))
pixel_values2 = image_processor(images=image2, return_tensors='pt').pixel_values.to(torch.bfloat16).cuda()
pixel_values = torch.cat((pixel_values1, pixel_values2), dim=0)
generation_config = dict(max_new_tokens=1024, do_sample=True)
question = '<image>\nDescribe the two images in detail.'
response, history = model.chat(tokenizer, pixel_values, question, generation_config,
history=None, return_history=True)
print(f'User: {question}')
print(f'Assistant: {response}')
question = 'What are the similarities and differences between these two images.'
response, history = model.chat(tokenizer, pixel_values, question, generation_config,
history=history, return_history=True)
print(f'User: {question}')
print(f'Assistant: {response}')
Multi-image multi-round conversation, separate images
β οΈοΈ Warning: Please note that for this model, we support multi-image chat in the interface, but the results are not very good due to the lack of training with multi-image data.
from transformers import AutoTokenizer, AutoModel, CLIPImageProcessor
from PIL import Image
import torch
path = "OpenGVLab/InternVL-Chat-V1-1"
model = AutoModel.from_pretrained(
path,
torch_dtype=torch.bfloat16,
low_cpu_mem_usage=True,
use_flash_attn=True,
trust_remote_code=True).eval().cuda()
tokenizer = AutoTokenizer.from_pretrained(path, trust_remote_code=True, use_fast=False)
image_processor = CLIPImageProcessor.from_pretrained(path)
image1 = Image.open('./examples/image1.jpg').resize((448, 448))
pixel_values1 = image_processor(images=image1, return_tensors='pt').pixel_values.to(torch.bfloat16).cuda()
image2 = Image.open('./examples/image2.jpg').resize((448, 448))
pixel_values2 = image_processor(images=image2, return_tensors='pt').pixel_values.to(torch.bfloat16).cuda()
pixel_values = torch.cat((pixel_values1, pixel_values2), dim=0)
num_patches_list = [pixel_values1.size(0), pixel_values2.size(0)]
generation_config = dict(max_new_tokens=1024, do_sample=True)
question = 'Image-1: <image>\nImage-2: <image>\nDescribe the two images in detail.'
response, history = model.chat(tokenizer, pixel_values, question, generation_config,
num_patches_list=num_patches_list, history=None, return_history=True)
print(f'User: {question}')
print(f'Assistant: {response}')
question = 'What are the similarities and differences between these two images.'
response, history = model.chat(tokenizer, pixel_values, question, generation_config,
num_patches_list=num_patches_list, history=history, return_history=True)
print(f'User: {question}')
print(f'Assistant: {response}')
Batch inference, single image per sample
from transformers import AutoTokenizer, AutoModel, CLIPImageProcessor
from PIL import Image
import torch
path = "OpenGVLab/InternVL-Chat-V1-1"
model = AutoModel.from_pretrained(
path,
torch_dtype=torch.bfloat16,
low_cpu_mem_usage=True,
use_flash_attn=True,
trust_remote_code=True).eval().cuda()
tokenizer = AutoTokenizer.from_pretrained(path, trust_remote_code=True, use_fast=False)
image_processor = CLIPImageProcessor.from_pretrained(path)
image1 = Image.open('./examples/image1.jpg').resize((448, 448))
pixel_values1 = image_processor(images=image1, return_tensors='pt').pixel_values.to(torch.bfloat16).cuda()
image2 = Image.open('./examples/image2.jpg').resize((448, 448))
pixel_values2 = image_processor(images=image2, return_tensors='pt').pixel_values.to(torch.bfloat16).cuda()
pixel_values = torch.cat((pixel_values1, pixel_values2), dim=0)
num_patches_list = [pixel_values1.size(0), pixel_values2.size(0)]
generation_config = dict(max_new_tokens=1024, do_sample=True)
questions = ['<image>\nDescribe the image in detail.'] * len(num_patches_list)
responses = model.batch_chat(tokenizer, pixel_values,
num_patches_list=num_patches_list,
questions=questions,
generation_config=generation_config)
for question, response in zip(questions, responses):
print(f'User: {question}')
print(f'Assistant: {response}')
Video multi-round conversation
β οΈοΈ Warning: Please note that for this model, we support video chat in the interface, but the results are not very good due to the lack of training with video data.
from transformers import AutoTokenizer, AutoModel, CLIPImageProcessor
from decord import VideoReader, cpu
from PIL import Image
import numpy as np
import torch
def get_index(bound, fps, max_frame, first_idx=0, num_segments=32):
if bound:
start, end = bound[0], bound[1]
else:
start, end = -100000, 100000
start_idx = max(first_idx, round(start * fps))
end_idx = min(round(end * fps), max_frame)
seg_size = float(end_idx - start_idx) / num_segments
frame_indices = np.array([
int(start_idx + (seg_size / 2) + np.round(seg_size * idx))
for idx in range(num_segments)
])
return frame_indices
def load_video(video_path, bound=None, num_segments=32):
vr = VideoReader(video_path, ctx=cpu(0), num_threads=1)
max_frame = len(vr) - 1
fps = float(vr.get_avg_fps())
pixel_values_list, num_patches_list = [], []
image_processor = CLIPImageProcessor.from_pretrained(path)
frame_indices = get_index(bound, fps, max_frame, first_idx=0, num_segments=num_segments)
for frame_index in frame_indices:
img = Image.fromarray(vr[frame_index].asnumpy()).convert('RGB').resize((448, 448))
pixel_values = image_processor(images=img, return_tensors='pt').pixel_values
num_patches_list.append(pixel_values.shape[0])
pixel_values_list.append(pixel_values)
pixel_values = torch.cat(pixel_values_list)
return pixel_values, num_patches_list
path = "OpenGVLab/InternVL-Chat-V1-1"
model = AutoModel.from_pretrained(
path,
torch_dtype=torch.bfloat16,
low_cpu_mem_usage=True,
use_flash_attn=True,
trust_remote_code=True).eval().cuda()
tokenizer = AutoTokenizer.from_pretrained(path, trust_remote_code=True, use_fast=False)
generation_config = dict(max_new_tokens=1024, do_sample=True)
video_path = './examples/red-panda.mp4'
pixel_values, num_patches_list = load_video(video_path, num_segments=8)
pixel_values = pixel_values.to(torch.bfloat16).cuda()
video_prefix = ''.join([f'Frame{i+1}: <image>\n' for i in range(len(num_patches_list))])
question = video_prefix + 'What is the red panda doing?'
# Frame1: <image>\nFrame2: <image>\n...\nFrame8: <image>\n{question}
response, history = model.chat(tokenizer, pixel_values, question, generation_config,
num_patches_list=num_patches_list, history=None, return_history=True)
print(f'User: {question}')
print(f'Assistant: {response}')
question = 'Describe this video in detail.'
response, history = model.chat(tokenizer, pixel_values, question, generation_config,
num_patches_list=num_patches_list, history=history, return_history=True)
print(f'User: {question}')
print(f'Assistant: {response}')
Streaming Output
Besides this method, you can also use the following code to get streamed output.
from transformers import TextIteratorStreamer
from threading import Thread
# Initialize the streamer
streamer = TextIteratorStreamer(tokenizer, skip_prompt=True, skip_special_tokens=True, timeout=10)
# Define the generation configuration
generation_config = dict(max_new_tokens=1024, do_sample=False, streamer=streamer)
# Start the model chat in a separate thread
thread = Thread(target=model.chat, kwargs=dict(
tokenizer=tokenizer, pixel_values=pixel_values, question=question,
history=None, return_history=False, generation_config=generation_config,
))
thread.start()
# Initialize an empty string to store the generated text
generated_text = ''
# Loop through the streamer to get the new text as it is generated
for new_text in streamer:
if new_text == model.conv_template.sep:
break
generated_text += new_text
print(new_text, end='', flush=True) # Print each new chunk of generated text on the same line
License
This project is released under the MIT license. Parts of this project contain code and models (e.g., LLaMA2) from other sources, which are subject to their respective licenses.
Citation
If you find this project useful in your research, please consider citing:
@article{chen2024expanding,
title={Expanding Performance Boundaries of Open-Source Multimodal Models with Model, Data, and Test-Time Scaling},
author={Chen, Zhe and Wang, Weiyun and Cao, Yue and Liu, Yangzhou and Gao, Zhangwei and Cui, Erfei and Zhu, Jinguo and Ye, Shenglong and Tian, Hao and Liu, Zhaoyang and others},
journal={arXiv preprint arXiv:2412.05271},
year={2024}
}
@article{gao2024mini,
title={Mini-internvl: A flexible-transfer pocket multimodal model with 5\% parameters and 90\% performance},
author={Gao, Zhangwei and Chen, Zhe and Cui, Erfei and Ren, Yiming and Wang, Weiyun and Zhu, Jinguo and Tian, Hao and Ye, Shenglong and He, Junjun and Zhu, Xizhou and others},
journal={arXiv preprint arXiv:2410.16261},
year={2024}
}
@article{chen2024far,
title={How Far Are We to GPT-4V? Closing the Gap to Commercial Multimodal Models with Open-Source Suites},
author={Chen, Zhe and Wang, Weiyun and Tian, Hao and Ye, Shenglong and Gao, Zhangwei and Cui, Erfei and Tong, Wenwen and Hu, Kongzhi and Luo, Jiapeng and Ma, Zheng and others},
journal={arXiv preprint arXiv:2404.16821},
year={2024}
}
@inproceedings{chen2024internvl,
title={Internvl: Scaling up vision foundation models and aligning for generic visual-linguistic tasks},
author={Chen, Zhe and Wu, Jiannan and Wang, Wenhai and Su, Weijie and Chen, Guo and Xing, Sen and Zhong, Muyan and Zhang, Qinglong and Zhu, Xizhou and Lu, Lewei and others},
booktitle={Proceedings of the IEEE/CVF Conference on Computer Vision and Pattern Recognition},
pages={24185--24198},
year={2024}
}
- Downloads last month
- 89