|
--- |
|
tags: |
|
- image-classification |
|
- auto-train |
|
- vision |
|
widget: |
|
- src: https://huggingface.co/datasets/mishig/sample_images/resolve/main/tiger.jpg |
|
example_title: Tiger |
|
- src: https://huggingface.co/datasets/mishig/sample_images/resolve/main/teapot.jpg |
|
example_title: Teapot |
|
- src: https://huggingface.co/datasets/mishig/sample_images/resolve/main/palace.jpg |
|
example_title: Palace |
|
datasets: |
|
- Falah/Blood_8_classes_Dataset |
|
license: apache-2.0 |
|
pipeline_tag: image-classification |
|
--- |
|
|
|
# WBC-Classifier(small-sized model) |
|
|
|
WBC-Classifier is a fine-tuned version of [resnet-50](https://huggingface.co/microsoft/resnet-50). This model has been fine tuned on this [dataset](https://huggingface.co/datasets/Falah/Blood_8_classes_Dataset) |
|
|
|
|
|
# ResNet-50 v1.5 |
|
|
|
ResNet model pre-trained on ImageNet-1k at resolution 224x224. It was introduced in the paper [Deep Residual Learning for Image Recognition](https://arxiv.org/abs/1512.03385) by He et al. |
|
|
|
|
|
## Model description |
|
|
|
ResNet (Residual Network) is a convolutional neural network that democratized the concepts of residual learning and skip connections. This enables to train much deeper models. |
|
|
|
This is ResNet v1.5, which differs from the original model: in the bottleneck blocks which require downsampling, v1 has stride = 2 in the first 1x1 convolution, whereas v1.5 has stride = 2 in the 3x3 convolution. This difference makes ResNet50 v1.5 slightly more accurate (\~0.5% top1) than v1, but comes with a small performance drawback (~5% imgs/sec) according to [Nvidia](https://catalog.ngc.nvidia.com/orgs/nvidia/resources/resnet_50_v1_5_for_pytorch). |
|
|
|
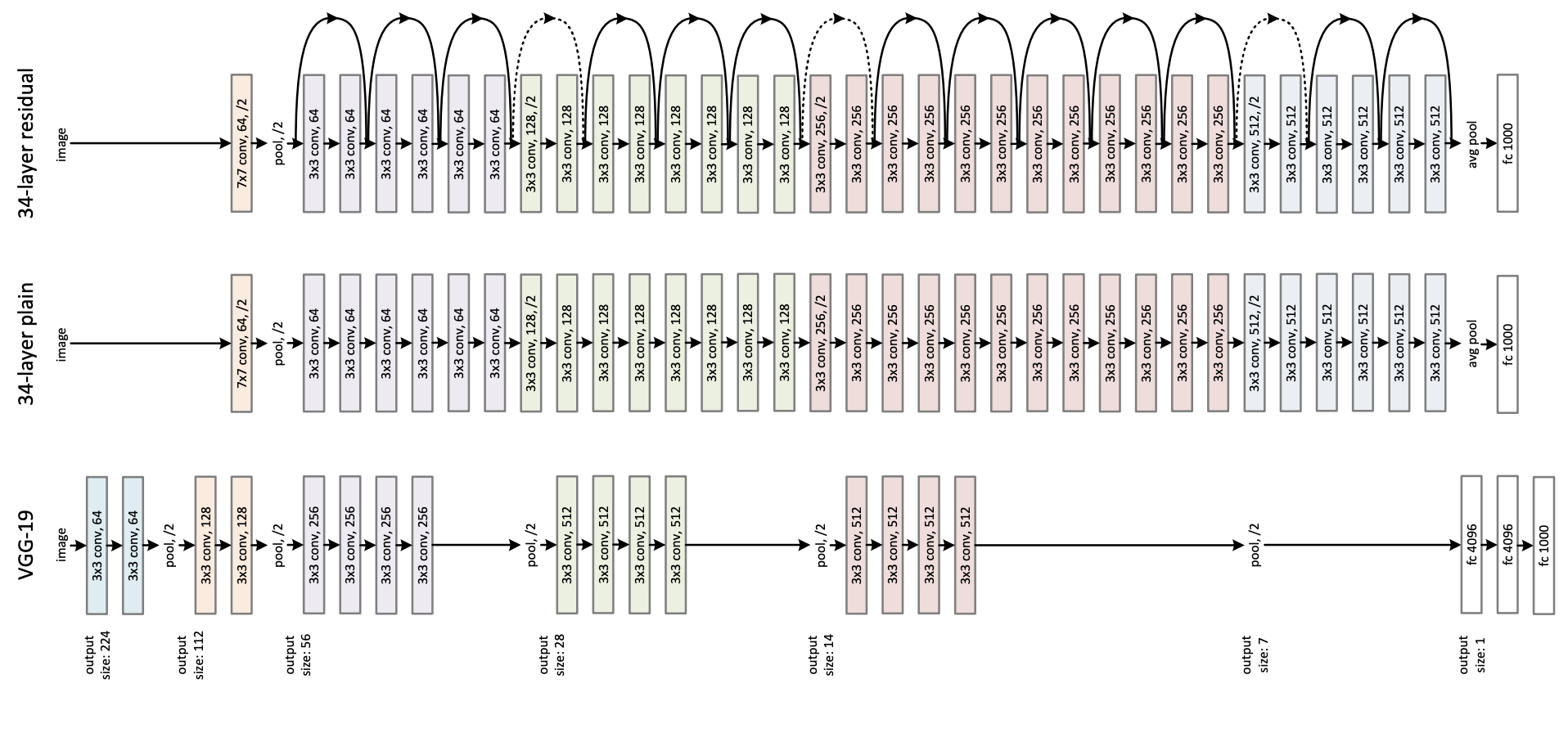 |
|
|
|
|
|
## Validation Metrics |
|
No validation metrics available |
|
|
|
|
|
### How to use |
|
|
|
Here is how to use this model to identify a neutrophil from a picture of a blood sample: |
|
|
|
```python |
|
from transformers import AutoImageProcessor, AutoModelForImageClassification |
|
from PIL import Image |
|
import requests |
|
|
|
processor = AutoImageProcessor.from_pretrained("NeuronZero/MRI-Reader") |
|
model = AutoModelForImageClassification.from_pretrained("NeuronZero/MRI-Reader") |
|
|
|
#dataset URL: "https://www.kaggle.com/datasets/paultimothymooney/blood-cells |
|
|
|
image_url = "https://storage.googleapis.com/kagglesdsdata/datasets/9232/29380/dataset-master/dataset-master/JPEGImages/BloodImage_00014.jpg?X-Goog-Algorithm=GOOG4-RSA-SHA256&X-Goog-Credential=databundle-worker-v2%40kaggle-161607.iam.gserviceaccount.com%2F20240404%2Fauto%2Fstorage%2Fgoog4_request&X-Goog-Date=20240404T094650Z&X-Goog-Expires=345600&X-Goog-SignedHeaders=host&X-Goog-Signature=8382f4e4b34038ad9176686e9d1155a757bbc41dcf99ee8cf5b5e049fa2994a9b877de41121c3dcea9b35c67076ddd5b3ff5ec970cbf5ac8f5a3eea1149eb68b8a0e79f91084a8598cefca35be190718b402bd6f581051f436ac771c85d3239834adc933e874fa31a6db696a968676610b6da955abd6145974b535b0509d196f68c8964c3dfb2404a0ad2248d1e80eb60d463e5ea58688820b46e6fc95f6fc3919e327905c2920912b8bda2241f8bcae8c886a66513ec62a8960188387322fbd1162caea76b1ecd04c433be5fbc5cd9f9a46e1a696df3cd0981b7e6243c6e5fd041ec928a080ea2845cdbea85fbfb38ff9024627c8a148c47ae50c4154197cfc" |
|
image = Image.open(requests.get(image_url, stream=True).raw) |
|
|
|
inputs = processor(images=image, return_tensors="pt") |
|
outputs = model(**inputs) |
|
logits = outputs.logits |
|
predicted_class_idx = logits.argmax(-1).item() |
|
print("Predicted class:", model.config.id2label[predicted_class_idx]) |
|
``` |