|
--- |
|
library_name: transformers |
|
tags: |
|
- LoRA |
|
- unsloth |
|
license: apache-2.0 |
|
language: |
|
- ja |
|
base_model: |
|
- IshiiTakahiro/llm-jp-3-13b-q-it-id2098_4bit |
|
--- |
|
|
|
# Model Card for Model ID |
|
|
|
<!-- Provide a quick summary of what the model is/does. --> |
|
This model is a **LoRA (Low-Rank Adaptation)** fine-tuned version of `IshiiTakahiro/llm-jp-3-13b-q-it-id2098_4bit`, designed for efficient parameter updates and task-specific customization. LoRA enables lightweight fine-tuning by adapting only a subset of model parameters, significantly reducing the computational and storage requirements. |
|
|
|
This llama model was trained 2x faster with [Unsloth](https://github.com/unslothai/unsloth) and Huggingface's TRL library. |
|
|
|
## Model Details |
|
|
|
### Model Architecture and Visual Abstract |
|
|
|
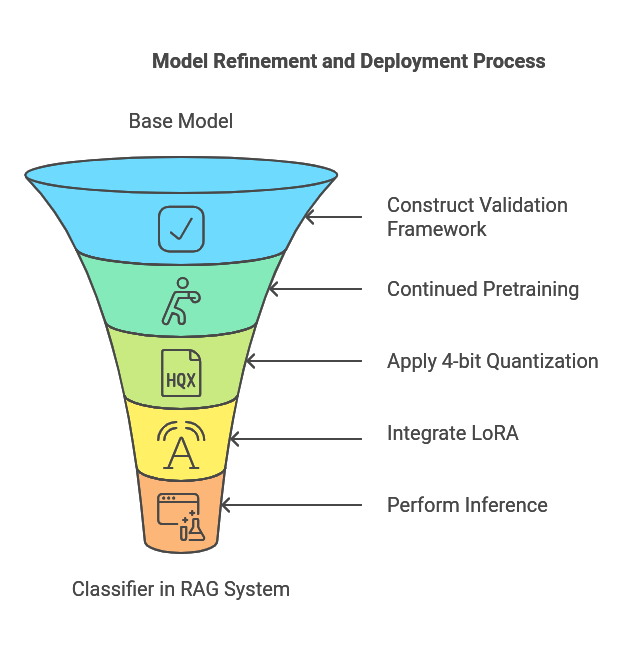 |
|
|
|
### Model Overview |
|
|
|
This is a LoRA (Low-Rank Adaptation) fine-tuned version of IshiiTakahiro/llm-jp-3-13b-q-it-id2098_4bit, designed for efficient parameter updates and task-specific customization. LoRA enables lightweight fine-tuning by adapting only a subset of model parameters, significantly reducing the computational and storage requirements. |
|
|
|
**Note**: The base model, "IshiiTakahiro/llm-jp-3-13b-q-it-id2098_16bit," is a further pre-trained version of "llm-jp/llm-jp-3-13b." Please be aware of this distinction. |
|
|
|
This is the model card of a 🤗 transformers model that has been pushed on the Hub. This model card has been automatically generated. |
|
|
|
- **Base Model**: IshiiTakahiro/llm-jp-3-13b-q-it-id2098_4bit |
|
- **Adaptation Type**: LoRA |
|
- **Language**: Japanese |
|
- **License**: Apache 2.0 |
|
|
|
This model specializes in tasks such as sentiment analysis, dialogue generation, and text summarization. |
|
|
|
--- |
|
|
|
## Intended Use |
|
|
|
### Primary Use Cases |
|
This LoRA model is ideal for the following tasks: |
|
1. **Text Generation:** Efficiently generate Japanese text for specific domains or use cases. |
|
2. **Text Classification:** Perform classification tasks with reduced resource consumption. |
|
3. **Domain-Specific Fine-Tuning:** Quickly adapt to niche tasks without retraining the entire model. |
|
|
|
### Out-of-Scope Use Cases |
|
- This LoRA model inherits limitations from the base model and should not be used for: |
|
- Generating harmful or biased content. |
|
- High-stakes decision-making in legal, medical, or critical scenarios. |
|
|
|
--- |
|
|
|
## How to Use |
|
|
|
### Installation |
|
Before running the code, install the required libraries: |
|
|
|
``` |
|
!pip uninstall unsloth -y && pip install "unsloth[colab-new] @ git+https://github.com/unslothai/unsloth.git" |
|
!pip install --no-deps xformers trl peft accelerate bitsandbytes jsonlines |
|
``` |
|
|
|
## Example Code |
|
```python |
|
|
|
from unsloth import FastLanguageModel |
|
import torch |
|
from tqdm import tqdm |
|
import random |
|
import numpy as np |
|
from multiprocessing import Pool, cpu_count |
|
import re |
|
import datetime |
|
import csv |
|
import jsonlines |
|
from google.colab import userdata |
|
HF_TOKEN=userdata.get('HF_TOKEN') |
|
|
|
peft_model, tokenizer = FastLanguageModel.from_pretrained( |
|
model_name="IshiiTakahiro/llm-jp-3-13b-it_lora", |
|
dtype=torch.bfloat16, |
|
load_in_4bit=False, |
|
trust_remote_code=True, |
|
token=HF_TOKEN, |
|
) |
|
def evaluate_task_score(task: str, answer: str) -> int: |
|
return 0 |
|
|
|
def batchify(data, batch_size): |
|
"""データをバッチに分割する関数""" |
|
for i in range(0, len(data), batch_size): |
|
yield data[i:i + batch_size] |
|
|
|
def score_prediction(task_input, predictions, index): |
|
scores = [ |
|
evaluate_task_score(task_input, prediction) |
|
for prediction in predictions |
|
] |
|
# ファイルに出力 |
|
output_file = f"{ID}.prediction_scores.csv" |
|
with open(output_file, mode="a", newline="", encoding="utf-8") as file: |
|
writer = csv.writer(file) |
|
for prediction, score in zip(predictions, scores): |
|
writer.writerow([index, task_input, prediction, score]) |
|
return index, scores |
|
|
|
datasets = [] |
|
# タスクとなるデータの読み込み。 |
|
# 事前にデータをアップロードしてください。 |
|
with jsonlines.open("./elyza-tasks-100-TV_0.jsonl", "r") as reader: |
|
datasets = list(reader) |
|
|
|
FastLanguageModel.for_inference(peft_model) |
|
peft_model = peft_model.to(dtype=torch.bfloat16) |
|
|
|
MAX_NEW_TOKENS:int = 2048 |
|
NUM_RETURN_SEQUENCES:int = 1 |
|
BATCH_SIZE:int = 1 |
|
ID:int = 1000 |
|
tasks_with_predictions = [] |
|
torch.cuda.empty_cache() |
|
|
|
for batch in tqdm(batchify(datasets, BATCH_SIZE), desc="Running inference on GPU"): |
|
batch_inputs = [] |
|
batch_task_ids = [] |
|
for dt in batch: |
|
input_text = dt["input"] |
|
annotation = f"### 注釈\n 追加情報: {random.randint(1, 100)}。この追加情報は無視してください。" |
|
prompt = f"### 指示\n{input_text}\n{annotation}\n### 回答\n" |
|
batch_inputs.append(prompt) |
|
batch_task_ids.append(dt["task_id"]) |
|
|
|
inputs = tokenizer( |
|
batch_inputs, |
|
return_tensors="pt", |
|
padding=True, |
|
truncation=True |
|
).to(peft_model.device) |
|
|
|
with torch.no_grad(): |
|
outputs = peft_model.generate( |
|
**inputs, |
|
max_new_tokens=MAX_NEW_TOKENS, |
|
use_cache=True, |
|
do_sample=False, |
|
repetition_penalty=1.07, |
|
early_stopping=True, |
|
num_return_sequences=NUM_RETURN_SEQUENCES, |
|
pad_token_id=tokenizer.pad_token_id, |
|
bos_token_id=tokenizer.bos_token_id, |
|
eos_token_id=tokenizer.eos_token_id |
|
) |
|
batch_predictions = [ |
|
tokenizer.decode(output, skip_special_tokens=True).split('\n### 回答')[-1].strip() |
|
for output in outputs |
|
] |
|
for task_id, input_text, prediction in zip(batch_task_ids, batch_inputs, batch_predictions): |
|
tasks_with_predictions.append((task_id, input_text, [prediction])) |
|
|
|
# CPUでのスコアリング(並列化) |
|
def cpu_scoring(task): |
|
task_id, task_input, predictions = task |
|
return score_prediction(task_input, predictions, task_id) |
|
|
|
with Pool(cpu_count()) as pool: |
|
scoring_results = list( |
|
tqdm(pool.imap(cpu_scoring, tasks_with_predictions), total=len(tasks_with_predictions), desc="Scoring on CPU") |
|
) |
|
|
|
# 最終結果の収集 |
|
results = [] |
|
for (task_id, task_input, predictions), (_, scores) in zip(tasks_with_predictions, scoring_results): |
|
best_index = np.argmax(scores) |
|
print("task_id:", task_id, ", best_index:", best_index) |
|
best_prediction = predictions[best_index] |
|
results.append({ |
|
"task_id": task_id, |
|
"input": task_input, |
|
"output": best_prediction |
|
}) |
|
|
|
# 保存 |
|
output_filename = f"id{ID}.jsonl" |
|
with jsonlines.open(output_filename, mode='w') as writer: |
|
writer.write_all(results) |
|
|
|
print(f"Results saved to {output_filename}") |
|
``` |
|
|
|
|
|
## Bias, Risks, and Limitations |
|
|
|
This LoRA model inherits biases and risks from its base model: |
|
|
|
- Cultural and Linguistic Bias: Outputs may reflect biases present in the Japanese-language training data. |
|
- Domain-Specific Limitations: Performance may degrade outside of the fine-tuned domain or task. |
|
|
|
### Recommendations |
|
|
|
- Validate outputs critically, especially when applied to sensitive domains. |
|
- Fine-tune further or evaluate carefully when adapting this model for a new domain. |
|
|
|
### Training Procedure |
|
|
|
This model was fine-tuned using LoRA, which updates only a small number of low-rank matrices: |
|
|
|
Base Model: IshiiTakahiro/llm-jp-3-13b-q-it-id2098_4bit |
|
LoRA Ranks: 8 |
|
Precision: bf16 |
|
Hardware: NVIDIA L4 GPUs |
|
|
|
LoRA significantly reduces computational overhead compared to full model fine-tuning, while maintaining performance on the target task. |
|
|
|
|
|
|
|
## Citation |
|
|
|
If you use this model, please cite it as follows: |
|
|
|
**BibTeX:** |
|
|
|
@misc{ishii2024lora, |
|
title={LoRA Adaptation of Large Japanese Language Model}, |
|
author={Takahiro Ishii}, |
|
year={2024}, |
|
note={Available at Hugging Face Hub: https://huggingface.co/IshiiTakahiro/llm-jp-3-13b-q-it-id2098_4bit} |
|
} |
|
|