gimme an invite! :D
Jordan Legg PRO
takarajordan
AI & ML interests
Chief AI Officer @takara.ai. Diffusion, Inference optimisation and all things MultiModal.
Recent Activity
updated
a Space
3 days ago
open-acc/README
new activity
3 days ago
open-acc/README:Update README.md
liked
a dataset
3 days ago
takarajordan/LastFM_120K
Organizations
takarajordan's activity
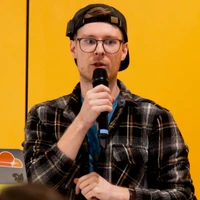
reacted to
s3nh's
post with โค๏ธ
6 days ago
Post
1605
Welcome back,
Small Language Models Enthusiasts and GPU Poor oss enjoyers lets connect.
Just created an organization which main target is to have fun with smaller models tuneable on consumer range GPUs, feel free to join and lets have some fun, much love ;3
https://huggingface.co/SmolTuners
Small Language Models Enthusiasts and GPU Poor oss enjoyers lets connect.
Just created an organization which main target is to have fun with smaller models tuneable on consumer range GPUs, feel free to join and lets have some fun, much love ;3
https://huggingface.co/SmolTuners
Amazing work
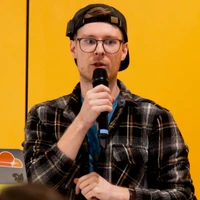
reacted to
merve's
post with ๐
6 days ago
Post
2319
Aya by Cohere For AI can now see! ๐
C4AI community has built Maya 8B, a new open-source multilingual VLM built on SigLIP and Aya 8B ๐ฑ works on 8 languages! ๐ฃ๏ธ
The authors extend Llava dataset using Aya's translation capabilities with 558k examples!
ry it here kkr5155/maya_demo
Dataset maya-multimodal/pretrain
Model maya-multimodal/maya ๐
kudos @nahidalam and team
C4AI community has built Maya 8B, a new open-source multilingual VLM built on SigLIP and Aya 8B ๐ฑ works on 8 languages! ๐ฃ๏ธ
The authors extend Llava dataset using Aya's translation capabilities with 558k examples!
ry it here kkr5155/maya_demo
Dataset maya-multimodal/pretrain
Model maya-multimodal/maya ๐
kudos @nahidalam and team
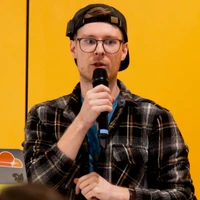
reacted to
merve's
post with ๐
6 days ago
Post
2902
Apollo is a new family of open-source video language models by Meta, where 3B model outperforms most 7B models and 7B outperforms most 30B models ๐งถ
โจ the models come in 1.5B https://huggingface.co/Apollo-LMMs/Apollo-1_5B-t32, 3B https://huggingface.co/Apollo-LMMs/Apollo-3B-t32 and 7B https://huggingface.co/Apollo-LMMs/Apollo-7B-t32 with A2.0 license, based on Qwen1.5 & Qwen2
โจ the authors also release a benchmark dataset https://huggingface.co/spaces/Apollo-LMMs/ApolloBench
The paper has a lot of experiments (they trained 84 models!) about what makes the video LMs work โฏ๏ธ
Try the demo for best setup here https://huggingface.co/spaces/Apollo-LMMs/Apollo-3B
they evaluate sampling strategies, scaling laws for models and datasets, video representation and more!
> The authors find out that whatever design decision was applied to small models also scale properly when the model and dataset are scaled ๐ scaling dataset has diminishing returns for smaller models
> They evaluate frame sampling strategies, and find that FPS sampling is better than uniform sampling, and they find 8-32 tokens per frame optimal
> They also compare image encoders, they try a variation of models from shape optimized SigLIP to DINOv2
they find google/siglip-so400m-patch14-384 to be most powerful ๐ฅ
> they also compare freezing different parts of models, training all stages with some frozen parts give the best yield
They eventually release three models, where Apollo-3B outperforms most 7B models and Apollo 7B outperforms 30B models ๐ฅ
โจ the models come in 1.5B https://huggingface.co/Apollo-LMMs/Apollo-1_5B-t32, 3B https://huggingface.co/Apollo-LMMs/Apollo-3B-t32 and 7B https://huggingface.co/Apollo-LMMs/Apollo-7B-t32 with A2.0 license, based on Qwen1.5 & Qwen2
โจ the authors also release a benchmark dataset https://huggingface.co/spaces/Apollo-LMMs/ApolloBench
The paper has a lot of experiments (they trained 84 models!) about what makes the video LMs work โฏ๏ธ
Try the demo for best setup here https://huggingface.co/spaces/Apollo-LMMs/Apollo-3B
they evaluate sampling strategies, scaling laws for models and datasets, video representation and more!
> The authors find out that whatever design decision was applied to small models also scale properly when the model and dataset are scaled ๐ scaling dataset has diminishing returns for smaller models
> They evaluate frame sampling strategies, and find that FPS sampling is better than uniform sampling, and they find 8-32 tokens per frame optimal
> They also compare image encoders, they try a variation of models from shape optimized SigLIP to DINOv2
they find google/siglip-so400m-patch14-384 to be most powerful ๐ฅ
> they also compare freezing different parts of models, training all stages with some frozen parts give the best yield
They eventually release three models, where Apollo-3B outperforms most 7B models and Apollo 7B outperforms 30B models ๐ฅ
you guys are amazing!
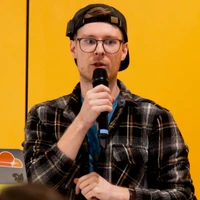
reacted to
lorraine2's
post with ๐
7 days ago
Post
1966
๐ฆNew NVIDIA paper: LLaMA-Mesh ๐ฆ
We enable large language models to generate and understand 3D meshes by representing them as text and fine-tuning. This unifies the 3D and text modalities in a single model and preserves language abilities, unlocking conversational 3D creation with mesh understanding.
๐ Project Page: https://research.nvidia.com/labs/toronto-ai/LLaMA-Mesh/
๐น๏ธ Interactive Demo: Zhengyi/LLaMA-Mesh (courtesy of HuggingFace and Gradio)
๐ Full Paper: https://arxiv.org/abs/2411.09595
๐จโ๐ปCode: https://github.com/nv-tlabs/LLaMa-Mesh
๐พ Model Checkpoint: Zhengyi/LLaMA-Mesh
๐งฉ Blender Addon: https://github.com/huggingface/meshgen (courtesy of Dylan Ebert)
๐ฅ 5-min Overview Video: https://youtu.be/eZNazN-1lPo?si=-idQa5aaceVw0Bbj (courtesy of AI Papers Academy)
We enable large language models to generate and understand 3D meshes by representing them as text and fine-tuning. This unifies the 3D and text modalities in a single model and preserves language abilities, unlocking conversational 3D creation with mesh understanding.
๐ Project Page: https://research.nvidia.com/labs/toronto-ai/LLaMA-Mesh/
๐น๏ธ Interactive Demo: Zhengyi/LLaMA-Mesh (courtesy of HuggingFace and Gradio)
๐ Full Paper: https://arxiv.org/abs/2411.09595
๐จโ๐ปCode: https://github.com/nv-tlabs/LLaMa-Mesh
๐พ Model Checkpoint: Zhengyi/LLaMA-Mesh
๐งฉ Blender Addon: https://github.com/huggingface/meshgen (courtesy of Dylan Ebert)
๐ฅ 5-min Overview Video: https://youtu.be/eZNazN-1lPo?si=-idQa5aaceVw0Bbj (courtesy of AI Papers Academy)
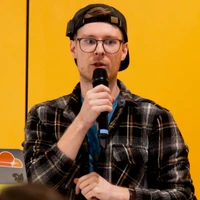
reacted to
DualityAI-RebekahBogdanoff's
post with โค๏ธ๐ฅ
7 days ago
Post
1932
Training YOLO with Synthetic Data from Duality AI's Falcon Simulation Software ๐ฎ๐
Hello again! ๐ Duality.ai has released a second Google Colab and tutorial for training a YOLOv8 model using synthetic data from our Falcon simulation software!
https://falcon.duality.ai/secure/documentation/see-synth-work-no-specs?sidebarMode=learn#download-the-colab-notebook
Train using synthetic images of a soup can twin this time, and see it work on real-world images. ๐ฅซ๐
The tutorial also walks you through how to add your own twin from our FalconCloud library, and our goal is to equip people like you to be able to create your own data for your own projects.
You'll have to create a free account to access the files, but once you do, you'll have access to not only this colab file, but also all of our lessons and our digital twin library. ๐
Instructions for creating the synthetic data accessed by the colab notebook can be found here: https://falcon.duality.ai/secure/documentation/ex2-objdetection-newtwin?sidebarMode=learn
This method is a game-changer for cost-effective, scalable, and customizable datasets in computer vision.
Why Synthetic Data?๐ค
- Precise Annotations: Get bounding boxes, segmentation masks, and more without manual effort.
- Customizable Scenarios: Get comprehensive data and cover all corner cases by simulating diverse conditions like lighting, weather, visual occlusions, and more.
Whatโs in the Notebook?๐
- Training & Evaluation: Train YOLOv8 with synthetic data and test its performance on real-world samples.
Letโs Discuss!๐ฌ
Check out our discord to see how people are using the Falcon simulation software to develop strong datasets and train robust models. https://discord.com/invite/dualityfalconcommunity
Hello again! ๐ Duality.ai has released a second Google Colab and tutorial for training a YOLOv8 model using synthetic data from our Falcon simulation software!
https://falcon.duality.ai/secure/documentation/see-synth-work-no-specs?sidebarMode=learn#download-the-colab-notebook
Train using synthetic images of a soup can twin this time, and see it work on real-world images. ๐ฅซ๐
The tutorial also walks you through how to add your own twin from our FalconCloud library, and our goal is to equip people like you to be able to create your own data for your own projects.
You'll have to create a free account to access the files, but once you do, you'll have access to not only this colab file, but also all of our lessons and our digital twin library. ๐
Instructions for creating the synthetic data accessed by the colab notebook can be found here: https://falcon.duality.ai/secure/documentation/ex2-objdetection-newtwin?sidebarMode=learn
This method is a game-changer for cost-effective, scalable, and customizable datasets in computer vision.
Why Synthetic Data?๐ค
- Precise Annotations: Get bounding boxes, segmentation masks, and more without manual effort.
- Customizable Scenarios: Get comprehensive data and cover all corner cases by simulating diverse conditions like lighting, weather, visual occlusions, and more.
Whatโs in the Notebook?๐
- Training & Evaluation: Train YOLOv8 with synthetic data and test its performance on real-world samples.
Letโs Discuss!๐ฌ
Check out our discord to see how people are using the Falcon simulation software to develop strong datasets and train robust models. https://discord.com/invite/dualityfalconcommunity
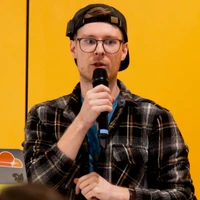
reacted to
prithivMLmods's
post with โค๏ธ
7 days ago
Post
3167
๐ Here Before - Xmas๐
โจ
๐ง๐ปโ๐Models
+ [ Xmas 2D Illustration ] : strangerzonehf/Flux-Xmas-Illustration-LoRA
+ [ Xmas 3D Art ] : strangerzonehf/Flux-Xmas-3D-LoRA
+ [ Xmas Chocolate ] : strangerzonehf/Flux-Xmas-Chocolate-LoRA
+ [ Xmas Isometric Kit ] : strangerzonehf/Flux-Xmas-Isometric-Kit-LoRA
+ [ Xmas Realpix ] : strangerzonehf/Flux-Xmas-Realpix-LoRA
+ [ Xmas Anime ] : strangerzonehf/Flux-Anime-Xmas-LoRA
โ๏ธCollections
+ [ Xmas Art ] : strangerzonehf/christmas-pack-6758b199487adafaddb68f82
+ [ Stranger Zone Collection ] : prithivMLmods/stranger-zone-collections-org-6737118adcf2cb40d66d0c7e
๐ฅถPage
+ [ Stranger Zone ] : https://huggingface.co/strangerzonehf
.
.
.
@prithivMLmods ๐ค
๐ง๐ปโ๐Models
+ [ Xmas 2D Illustration ] : strangerzonehf/Flux-Xmas-Illustration-LoRA
+ [ Xmas 3D Art ] : strangerzonehf/Flux-Xmas-3D-LoRA
+ [ Xmas Chocolate ] : strangerzonehf/Flux-Xmas-Chocolate-LoRA
+ [ Xmas Isometric Kit ] : strangerzonehf/Flux-Xmas-Isometric-Kit-LoRA
+ [ Xmas Realpix ] : strangerzonehf/Flux-Xmas-Realpix-LoRA
+ [ Xmas Anime ] : strangerzonehf/Flux-Anime-Xmas-LoRA
โ๏ธCollections
+ [ Xmas Art ] : strangerzonehf/christmas-pack-6758b199487adafaddb68f82
+ [ Stranger Zone Collection ] : prithivMLmods/stranger-zone-collections-org-6737118adcf2cb40d66d0c7e
๐ฅถPage
+ [ Stranger Zone ] : https://huggingface.co/strangerzonehf
.
.
.
@prithivMLmods ๐ค
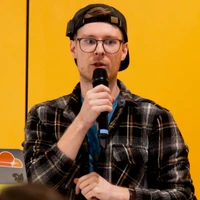
reacted to
davidberenstein1957's
post with ๐ฅ
7 days ago
Post
4105
Introducing the Synthetic Data Generator, a user-friendly application that takes a no-code approach to creating custom datasets with Large Language Models (LLMs). The best part: A simple step-by-step process, making dataset creation a non-technical breeze, allowing anyone to create datasets and models in minutes and without any code.
Blog: https://huggingface.co/blog/synthetic-data-generator
Space: argilla/synthetic-data-generator
Blog: https://huggingface.co/blog/synthetic-data-generator
Space: argilla/synthetic-data-generator
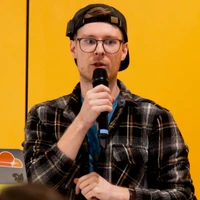
reacted to
cutechicken's
post with โค๏ธ
7 days ago
Post
2822
๐ RAGOndevice: High-Performance Local AI Document Analysis Assistant
๐ซ Core Value
RAGOndevice is a high-performance AI system running locally without cloud dependency. Using CohereForAI's optimized 7B model, it enables professional-grade document analysis on standard PCs. โจ
๐ Ondevice AI Advantages
1. ๐ Efficient Resource Utilization
๐ฏ Optimized 7B Model: Runs on standard PCs
โก Local Processing: Instant response without cloud
๐ป Low-Spec Compatible: Performs well on regular GPUs
๐ Optimized Memory: Ensures stable operation
2. ๐ก๏ธ Data Security & Cost Efficiency
๐ Complete Privacy: No external data transmission
๐ Offline Operation: No internet required
๐ฐ No Subscription: One-time installation
โ๏ธ Resource Optimization: Uses existing hardware
๐ฎ Key Features
1. ๐ Powerful Document Analysis
๐ Multi-Format Support: TXT, CSV, PDF, Parquet
๐ง Intelligent Analysis: Automatic structure recognition
๐๏ธ OCR Support: Advanced PDF text extraction
๐ฌ Real-time Chat: Natural language interaction
2. ๐ Local RAG System
๐ฏ Efficient Search: TF-IDF based local search
๐งฉ Context Understanding: Accurate information retrieval
๐ Wikipedia Integration: Rich background knowledge
๐ฏ Use Cases
๐ข Enterprise: Secure confidential document processing
๐ฌ Personal Research: Private data analysis
๐ Education: Personal learning material analysis
๐ป Development: Local codebase analysis
โญ Differentiators
๐โโ๏ธ Independent Operation: Zero cloud dependency
โก Instant Response: No network latency
๐ Complete Security: Full data control
๐ Cost Efficiency: No ongoing costs
๐ฎ Future Plans
๐ Enhanced model optimization
๐ Local knowledge base expansion
โก Hardware optimization
๐ Extended file support
๐ RAGOndevice democratizes high-performance AI, providing the optimal local AI solution for security-sensitive environments. ๐
๐ฅ Power of Local AI: Experience enterprise-grade AI capabilities right on your device!
VIDraft/RAGOndevice
๐ซ Core Value
RAGOndevice is a high-performance AI system running locally without cloud dependency. Using CohereForAI's optimized 7B model, it enables professional-grade document analysis on standard PCs. โจ
๐ Ondevice AI Advantages
1. ๐ Efficient Resource Utilization
๐ฏ Optimized 7B Model: Runs on standard PCs
โก Local Processing: Instant response without cloud
๐ป Low-Spec Compatible: Performs well on regular GPUs
๐ Optimized Memory: Ensures stable operation
2. ๐ก๏ธ Data Security & Cost Efficiency
๐ Complete Privacy: No external data transmission
๐ Offline Operation: No internet required
๐ฐ No Subscription: One-time installation
โ๏ธ Resource Optimization: Uses existing hardware
๐ฎ Key Features
1. ๐ Powerful Document Analysis
๐ Multi-Format Support: TXT, CSV, PDF, Parquet
๐ง Intelligent Analysis: Automatic structure recognition
๐๏ธ OCR Support: Advanced PDF text extraction
๐ฌ Real-time Chat: Natural language interaction
2. ๐ Local RAG System
๐ฏ Efficient Search: TF-IDF based local search
๐งฉ Context Understanding: Accurate information retrieval
๐ Wikipedia Integration: Rich background knowledge
๐ฏ Use Cases
๐ข Enterprise: Secure confidential document processing
๐ฌ Personal Research: Private data analysis
๐ Education: Personal learning material analysis
๐ป Development: Local codebase analysis
โญ Differentiators
๐โโ๏ธ Independent Operation: Zero cloud dependency
โก Instant Response: No network latency
๐ Complete Security: Full data control
๐ Cost Efficiency: No ongoing costs
๐ฎ Future Plans
๐ Enhanced model optimization
๐ Local knowledge base expansion
โก Hardware optimization
๐ Extended file support
๐ RAGOndevice democratizes high-performance AI, providing the optimal local AI solution for security-sensitive environments. ๐
๐ฅ Power of Local AI: Experience enterprise-grade AI capabilities right on your device!
VIDraft/RAGOndevice
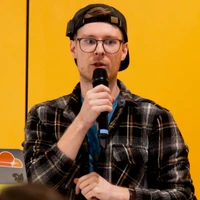
reacted to
lewtun's
post with ๐ฅ๐
7 days ago
Post
6427
We outperform Llama 70B with Llama 3B on hard math by scaling test-time compute ๐ฅ
How? By combining step-wise reward models with tree search algorithms :)
We show that smol models can match or exceed the performance of their much larger siblings when given enough "time to think"
We're open sourcing the full recipe and sharing a detailed blog post.
In our blog post we cover:
๐ Compute-optimal scaling: How we implemented DeepMind's recipe to boost the mathematical capabilities of open models at test-time.
๐ Diverse Verifier Tree Search (DVTS): An unpublished extension we developed to the verifier-guided tree search technique. This simple yet effective method improves diversity and delivers better performance, particularly at large test-time compute budgets.
๐งญ Search and Learn: A lightweight toolkit for implementing search strategies with LLMs and built for speed with vLLM
Here's the links:
- Blog post: HuggingFaceH4/blogpost-scaling-test-time-compute
- Code: https://github.com/huggingface/search-and-learn
Enjoy!
How? By combining step-wise reward models with tree search algorithms :)
We show that smol models can match or exceed the performance of their much larger siblings when given enough "time to think"
We're open sourcing the full recipe and sharing a detailed blog post.
In our blog post we cover:
๐ Compute-optimal scaling: How we implemented DeepMind's recipe to boost the mathematical capabilities of open models at test-time.
๐ Diverse Verifier Tree Search (DVTS): An unpublished extension we developed to the verifier-guided tree search technique. This simple yet effective method improves diversity and delivers better performance, particularly at large test-time compute budgets.
๐งญ Search and Learn: A lightweight toolkit for implementing search strategies with LLMs and built for speed with vLLM
Here's the links:
- Blog post: HuggingFaceH4/blogpost-scaling-test-time-compute
- Code: https://github.com/huggingface/search-and-learn
Enjoy!
Post
1045
I made an RSS feed for HuggingFace Daily Papers!! ๐ค
Just Subscribe here: https://papers.takara.ai/api/feed
It updates every 24 hours, completely written as a serverless go script with a Redis cache (to avoid hitting HF all the time).
I'm open sourcing the code, you can check out my repo and deploy it on Vercel extremely easily!
https://github.com/404missinglink/HF-Daily-Papers-Feeds
thanks to @John6666 @p3nGu1nZz for your early support
Just Subscribe here: https://papers.takara.ai/api/feed
It updates every 24 hours, completely written as a serverless go script with a Redis cache (to avoid hitting HF all the time).
I'm open sourcing the code, you can check out my repo and deploy it on Vercel extremely easily!
https://github.com/404missinglink/HF-Daily-Papers-Feeds
thanks to @John6666 @p3nGu1nZz for your early support
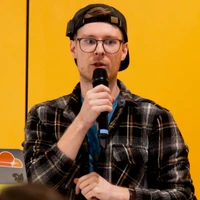
replied to
their
post
7 days ago
Thanks Clem!!
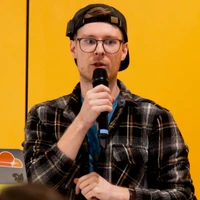
replied to
their
post
7 days ago
I preprocessed this into ChatML format to train the model takarajordan/WorldScenario-3.2B_GGUF and I used Unsloth to finetune it!
If you want more help join the HuggingFace discord, I'm always in there.
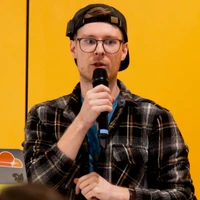
reacted to
prithivMLmods's
post with ๐ค
7 days ago
Post
3167
๐ Here Before - Xmas๐
โจ
๐ง๐ปโ๐Models
+ [ Xmas 2D Illustration ] : strangerzonehf/Flux-Xmas-Illustration-LoRA
+ [ Xmas 3D Art ] : strangerzonehf/Flux-Xmas-3D-LoRA
+ [ Xmas Chocolate ] : strangerzonehf/Flux-Xmas-Chocolate-LoRA
+ [ Xmas Isometric Kit ] : strangerzonehf/Flux-Xmas-Isometric-Kit-LoRA
+ [ Xmas Realpix ] : strangerzonehf/Flux-Xmas-Realpix-LoRA
+ [ Xmas Anime ] : strangerzonehf/Flux-Anime-Xmas-LoRA
โ๏ธCollections
+ [ Xmas Art ] : strangerzonehf/christmas-pack-6758b199487adafaddb68f82
+ [ Stranger Zone Collection ] : prithivMLmods/stranger-zone-collections-org-6737118adcf2cb40d66d0c7e
๐ฅถPage
+ [ Stranger Zone ] : https://huggingface.co/strangerzonehf
.
.
.
@prithivMLmods ๐ค
๐ง๐ปโ๐Models
+ [ Xmas 2D Illustration ] : strangerzonehf/Flux-Xmas-Illustration-LoRA
+ [ Xmas 3D Art ] : strangerzonehf/Flux-Xmas-3D-LoRA
+ [ Xmas Chocolate ] : strangerzonehf/Flux-Xmas-Chocolate-LoRA
+ [ Xmas Isometric Kit ] : strangerzonehf/Flux-Xmas-Isometric-Kit-LoRA
+ [ Xmas Realpix ] : strangerzonehf/Flux-Xmas-Realpix-LoRA
+ [ Xmas Anime ] : strangerzonehf/Flux-Anime-Xmas-LoRA
โ๏ธCollections
+ [ Xmas Art ] : strangerzonehf/christmas-pack-6758b199487adafaddb68f82
+ [ Stranger Zone Collection ] : prithivMLmods/stranger-zone-collections-org-6737118adcf2cb40d66d0c7e
๐ฅถPage
+ [ Stranger Zone ] : https://huggingface.co/strangerzonehf
.
.
.
@prithivMLmods ๐ค
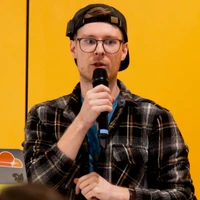
reacted to
freddyaboulton's
post with ๐
7 days ago
Post
1103
Just created a Gradio space for playing with the new OAI realtime voice API!
freddyaboulton/openai-realtime-voice
freddyaboulton/openai-realtime-voice