File size: 5,957 Bytes
49ee142 5b1efcc e7930d4 5b1efcc e7930d4 4bce7b6 e7930d4 988edaf e7930d4 |
1 2 3 4 5 6 7 8 9 10 11 12 13 14 15 16 17 18 19 20 21 22 23 24 25 26 27 28 29 30 31 32 33 34 35 36 37 38 39 40 41 42 43 44 45 46 47 48 49 50 51 52 53 54 55 56 57 58 59 60 61 62 63 64 65 66 67 68 69 70 71 72 73 74 75 76 77 78 79 80 81 82 83 84 85 86 87 88 89 90 91 92 93 94 95 96 97 98 99 100 101 102 103 104 105 106 107 108 109 110 111 112 113 114 115 116 117 118 119 120 121 122 123 124 125 126 127 128 129 130 131 132 133 134 135 136 137 138 139 140 141 142 143 144 145 146 147 148 149 150 151 152 153 154 155 156 157 158 159 160 161 162 163 164 165 166 167 168 169 170 171 172 173 |
---
pipeline_tag: text-generation
tags:
- text-generation-inference
- backpack
- backpackmodel
library_name: transformers
license: apache-2.0
datasets:
- openwebtext
language:
- en
---
---
---
# Model Card for Backpack-GPT2
<!-- Provide a quick summary of what the model is/does. [Optional] -->
The Backpack-GPT2 language model is an instance of the [Backpack architecture](https://arxiv.org/abs/2305.16765), intended to combine strong modeling performance with an interface for interpretability and control.
Most details about this model and its training should be accessed in the paper, [Backpack Language Models](https://arxiv.org/abs/2305.16765).
See also [backpackmodels.science](backpackmodels.science).
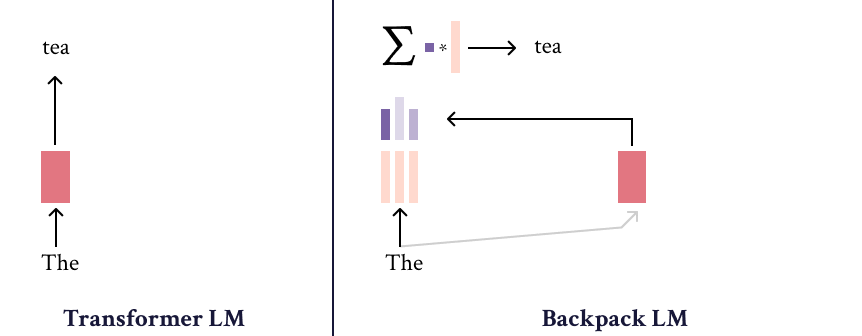
# Table of Contents
- [Model Card for Backpack-GPT2](#model-card-for--model_id-)
- [Table of Contents](#table-of-contents)
- [Model Details](#model-details)
- [Model Description](#model-description)
- [Uses](#uses)
- [Bias, Risks, and Limitations](#bias-risks-and-limitations)
- [Training Details](#training-details)
- [Training Data](#training-data)
- [Training Procedure](#training-procedure)
- [Environmental Impact](#environmental-impact)
- [Technical Specifications [optional]](#technical-specifications-optional)
- [Model Architecture and Objective](#model-architecture-and-objective)
- [Compute Infrastructure](#compute-infrastructure)
- [Hardware](#hardware)
- [Software](#software)
- [Citation](#citation)
- [Model Card Authors [optional]](#model-card-authors-optional)
- [Model Card Contact](#model-card-contact)
- [How to Get Started with the Model](#how-to-get-started-with-the-model)
# Model Details
## Model Description
<!-- Provide a longer summary of what this model is/does. -->
The Backpack-GPT2 is a [Backpack-based language model](https://arxiv.org/abs/2305.16765), an architecture intended to combine strong modeling performance with an interface for interpretability and control.
- **Developed by:** John Hewitt, John Thickstun, Christopher D. Manning, Percy Liang
- **Shared by [Optional]:** More information needed
- **Model type:** Language model
- **Language(s) (NLP):** en
- **License:** apache-2.0
- **Resources for more information:**
- [GitHub Repo](https://github.com/john-hewitt/backpacks-flash-attn)
- [Associated Paper](https://huggingface.co/datasets/openwebtext)
# Uses
This model is intended for use in the study and development of increasingly interpretable methods in natural language processing.
It is not directly fit for any production use.
# Bias, Risks, and Limitations
<!-- This section is meant to convey both technical and sociotechnical limitations. -->
Significant research has explored bias and fairness issues with language models (see, e.g., [Sheng et al. (2021)](https://aclanthology.org/2021.acl-long.330.pdf) and [Bender et al. (2021)](https://dl.acm.org/doi/pdf/10.1145/3442188.3445922)). Predictions generated by the model may include disturbing and harmful stereotypes across protected classes; identity characteristics; and sensitive, social, and occupational groups.
This model in particular is limited in its capabilities, and with a brand new architecture, less is known about its biases than, e.g., Transformer-based models.
# Training Details
## Training Data
<!-- This should link to a Data Card, perhaps with a short stub of information on what the training data is all about as well as documentation related to data pre-processing or additional filtering. -->
This model was trained on the [OpenWebText](https://huggingface.co/datasets/openwebtext) corpus.
## Training Procedure
This model was trained for 100k gradient steps with a batch size of 512k tokens and a linearly decaying learning rate from 6e-4 to zero, with a linear warmup of 5k steps.
# Environmental Impact
- **Hardware Type:** 4 A100 GPUs (40G)
- **Hours used:** Roughly 4 days.
- **Cloud Provider:** Stanford compute.
- **Compute Region:** Stanford energy grid.
## Model Architecture and Objective
This model was trained to minimize the cross-entropy loss, and is a [Backpack language model](https://arxiv.org/pdf/2305.16765.pdf).
## Compute Infrastructure
This model was trained on a slurm cluster.
### Hardware
This model was trained on 4 A100s.
### Software
This model was trained with [FlashAttention](https://github.com/HazyResearch/flash-attention) and [PyTorch](https://pytorch.org/)
# Citation
**BibTeX:**
```
@InProceedings{hewitt2023backpack,
author = "Hewitt, John and Thickstun, John and Manning, Christopher D. and Liang, Percy",
title = "Backpack Language Models",
booktitle = "Proceedings of the Association for Computational Linguistics",
year = "2023",
publisher = "Association for Computational Linguistics",
location = "Toronto, Canada",
}
```
# Model Card Authors [optional]
<!-- This section provides another layer of transparency and accountability. Whose views is this model card representing? How many voices were included in its construction? Etc. -->
John Hewitt
# Model Card Contact
[email protected]
# How to Get Started with the Model
```
import torch
import transformers
from transformers import AutoModelForCausalLM
model_id = "stanfordnlp/backpack-gpt2"
config = transformers.AutoConfig.from_pretrained(model_id, trust_remote_code=True)
torch_model = AutoModelForCausalLM.from_pretrained(model_id, config=config, trust_remote_code=True)
torch_model.eval()
input = torch.randint(0, 50264, (1, 512), dtype=torch.long)
torch_out = torch_model(
input,
position_ids=None,
)
torch_out = torch.nn.functional.softmax(torch_out.logits, dim=-1)
print(torch_out)
```
<details>
<summary> Click to expand </summary>
More information needed
</details> |