File size: 16,006 Bytes
208b0eb |
1 2 3 4 5 6 7 8 9 10 11 12 13 14 15 16 17 18 19 20 21 22 23 24 25 26 27 28 29 30 31 32 33 34 35 36 37 38 39 40 41 42 43 44 45 46 47 48 49 50 51 52 53 54 55 56 57 58 59 60 61 62 63 64 65 66 67 68 69 70 71 72 73 74 75 76 77 78 79 80 81 82 83 84 85 86 87 88 89 90 91 92 93 94 95 96 97 98 99 100 101 102 103 104 105 106 107 108 109 110 111 112 113 114 115 116 117 118 119 120 121 122 123 124 125 126 127 128 129 130 131 132 133 134 135 136 137 138 139 140 141 142 143 144 145 146 147 148 149 150 151 152 153 154 155 156 157 158 159 160 161 162 163 164 165 166 167 168 169 170 171 172 173 174 175 176 177 178 179 180 181 182 183 184 185 186 187 188 189 190 191 192 193 194 195 196 197 198 199 200 201 202 203 204 205 206 207 208 209 210 211 212 213 214 215 216 217 218 219 220 221 222 223 224 225 226 227 228 229 230 231 232 233 234 235 236 237 238 239 240 241 242 243 244 245 246 247 248 249 250 251 252 253 254 255 256 257 258 259 260 261 262 263 264 265 266 267 268 269 270 271 272 273 274 275 276 277 278 279 280 281 282 283 284 285 286 287 288 289 290 291 292 293 294 295 296 297 298 299 300 301 302 303 304 305 306 307 308 309 310 311 312 313 314 315 316 317 318 319 320 321 322 323 324 325 326 327 328 329 330 331 332 333 334 335 336 337 338 339 340 341 342 343 344 345 346 347 348 349 350 351 352 353 354 355 356 357 358 359 360 361 362 363 364 365 366 367 368 369 370 371 372 373 374 375 376 |
# CogVideoX-Fun
😊 Welcome!
[](https://huggingface.co/spaces/alibaba-pai/CogVideoX-Fun-5b)
[English](./README.md) | 简体中文
# 目录
- [目录](#目录)
- [简介](#简介)
- [快速启动](#快速启动)
- [视频作品](#视频作品)
- [如何使用](#如何使用)
- [模型地址](#模型地址)
- [未来计划](#未来计划)
- [参考文献](#参考文献)
- [许可证](#许可证)
# 简介
CogVideoX-Fun是一个基于CogVideoX结构修改后的的pipeline,是一个生成条件更自由的CogVideoX,可用于生成AI图片与视频、训练Diffusion Transformer的基线模型与Lora模型,我们支持从已经训练好的CogVideoX-Fun模型直接进行预测,生成不同分辨率,6秒左右、fps8的视频(1 ~ 49帧),也支持用户训练自己的基线模型与Lora模型,进行一定的风格变换。
我们会逐渐支持从不同平台快速启动,请参阅 [快速启动](#快速启动)。
新特性:
- CogVideoX-Fun Control现在在diffusers中得到了支持。感谢 [a-r-r-o-w](https://github.com/a-r-r-o-w)在这个 [PR](https://github.com/huggingface/diffusers/pull/9671)中贡献了支持。查看[文档](https://huggingface.co/docs/diffusers/main/en/api/pipelines/cogvideox)以了解更多信息。[2024.10.16]
- 重新训练i2v模型,添加Noise,使得视频的运动幅度更大。上传控制模型训练代码与Control模型。[ 2024.09.29 ]
- 创建代码!现在支持 Windows 和 Linux。支持2b与5b最大256x256x49到1024x1024x49的任意分辨率的视频生成。[ 2024.09.18 ]
功能概览:
- [数据预处理](#data-preprocess)
- [训练DiT](#dit-train)
- [模型生成](#video-gen)
我们的ui界面如下:
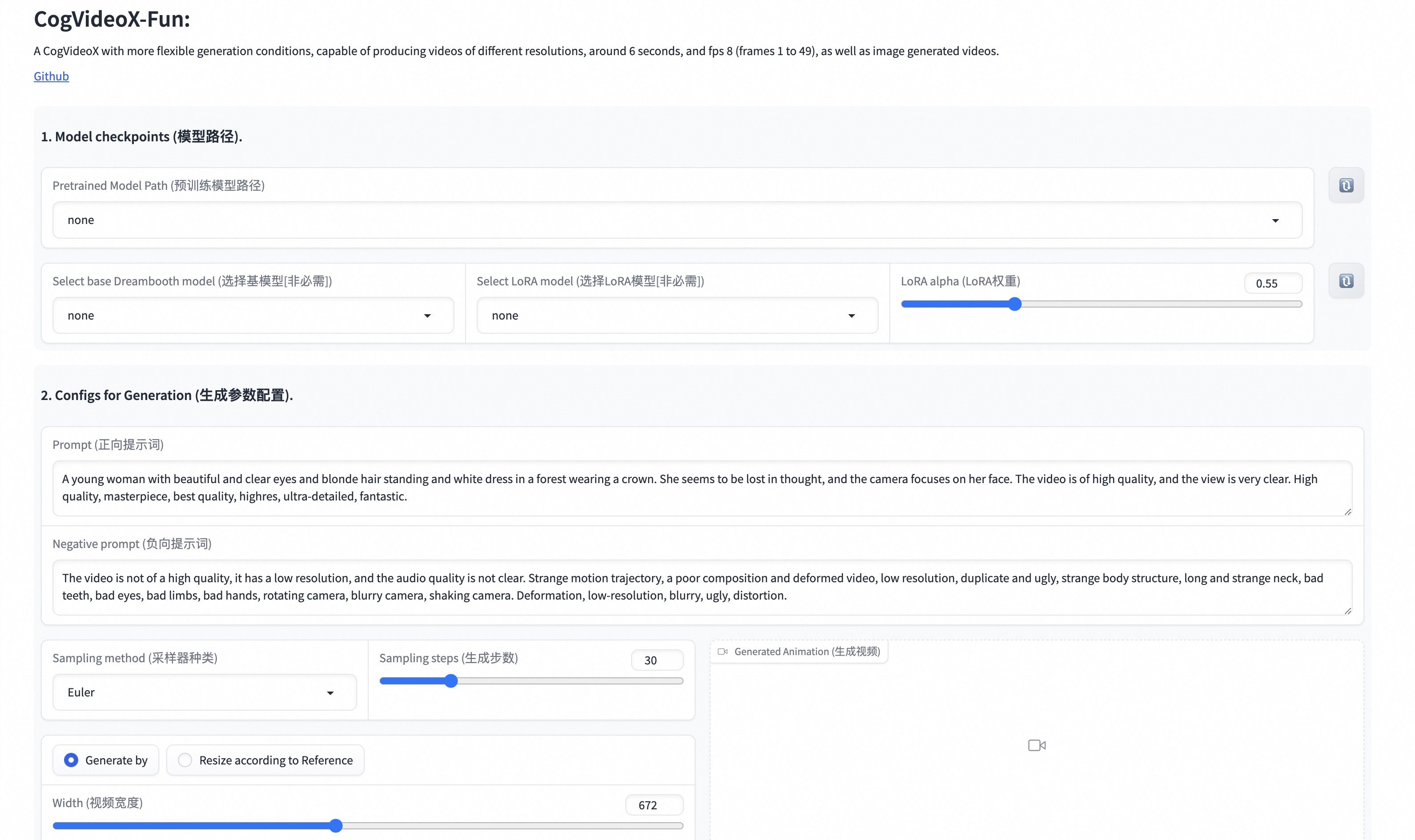
# 快速启动
### 1. 云使用: AliyunDSW/Docker
#### a. 通过阿里云 DSW
DSW 有免费 GPU 时间,用户可申请一次,申请后3个月内有效。
阿里云在[Freetier](https://free.aliyun.com/?product=9602825&crowd=enterprise&spm=5176.28055625.J_5831864660.1.e939154aRgha4e&scm=20140722.M_9974135.P_110.MO_1806-ID_9974135-MID_9974135-CID_30683-ST_8512-V_1)提供免费GPU时间,获取并在阿里云PAI-DSW中使用,5分钟内即可启动CogVideoX-Fun。
[](https://gallery.pai-ml.com/#/preview/deepLearning/cv/cogvideox_fun)
#### b. 通过ComfyUI
我们的ComfyUI界面如下,具体查看[ComfyUI README](comfyui/README.md)。

#### c. 通过docker
使用docker的情况下,请保证机器中已经正确安装显卡驱动与CUDA环境,然后以此执行以下命令:
```
# pull image
docker pull mybigpai-public-registry.cn-beijing.cr.aliyuncs.com/easycv/torch_cuda:cogvideox_fun
# enter image
docker run -it -p 7860:7860 --network host --gpus all --security-opt seccomp:unconfined --shm-size 200g mybigpai-public-registry.cn-beijing.cr.aliyuncs.com/easycv/torch_cuda:cogvideox_fun
# clone code
git clone https://github.com/aigc-apps/CogVideoX-Fun.git
# enter CogVideoX-Fun's dir
cd CogVideoX-Fun
# download weights
mkdir models/Diffusion_Transformer
mkdir models/Personalized_Model
wget https://pai-aigc-photog.oss-cn-hangzhou.aliyuncs.com/cogvideox_fun/Diffusion_Transformer/CogVideoX-Fun-V1.1-2b-InP.tar.gz -O models/Diffusion_Transformer/CogVideoX-Fun-V1.1-2b-InP.tar.gz
cd models/Diffusion_Transformer/
tar -xvf CogVideoX-Fun-V1.1-2b-InP.tar.gz
cd ../../
```
### 2. 本地安装: 环境检查/下载/安装
#### a. 环境检查
我们已验证CogVideoX-Fun可在以下环境中执行:
Windows 的详细信息:
- 操作系统 Windows 10
- python: python3.10 & python3.11
- pytorch: torch2.2.0
- CUDA: 11.8 & 12.1
- CUDNN: 8+
- GPU: Nvidia-3060 12G & Nvidia-3090 24G
Linux 的详细信息:
- 操作系统 Ubuntu 20.04, CentOS
- python: python3.10 & python3.11
- pytorch: torch2.2.0
- CUDA: 11.8 & 12.1
- CUDNN: 8+
- GPU:Nvidia-V100 16G & Nvidia-A10 24G & Nvidia-A100 40G & Nvidia-A100 80G
我们需要大约 60GB 的可用磁盘空间,请检查!
#### b. 权重放置
我们最好将[权重](#model-zoo)按照指定路径进行放置:
```
📦 models/
├── 📂 Diffusion_Transformer/
│ ├── 📂 CogVideoX-Fun-V1.1-2b-InP/
│ └── 📂 CogVideoX-Fun-V1.1-5b-InP/
├── 📂 Personalized_Model/
│ └── your trained trainformer model / your trained lora model (for UI load)
```
# 视频作品
所展示的结果都是图生视频获得。
### CogVideoX-Fun-V1.1-5B
Resolution-1024
<table border="0" style="width: 100%; text-align: left; margin-top: 20px;">
<tr>
<td>
<video src="https://github.com/user-attachments/assets/34e7ec8f-293e-4655-bb14-5e1ee476f788" width="100%" controls autoplay loop></video>
</td>
<td>
<video src="https://github.com/user-attachments/assets/7809c64f-eb8c-48a9-8bdc-ca9261fd5434" width="100%" controls autoplay loop></video>
</td>
<td>
<video src="https://github.com/user-attachments/assets/8e76aaa4-c602-44ac-bcb4-8b24b72c386c" width="100%" controls autoplay loop></video>
</td>
<td>
<video src="https://github.com/user-attachments/assets/19dba894-7c35-4f25-b15c-384167ab3b03" width="100%" controls autoplay loop></video>
</td>
</tr>
</table>
Resolution-768
<table border="0" style="width: 100%; text-align: left; margin-top: 20px;">
<tr>
<td>
<video src="https://github.com/user-attachments/assets/0bc339b9-455b-44fd-8917-80272d702737" width="100%" controls autoplay loop></video>
</td>
<td>
<video src="https://github.com/user-attachments/assets/70a043b9-6721-4bd9-be47-78b7ec5c27e9" width="100%" controls autoplay loop></video>
</td>
<td>
<video src="https://github.com/user-attachments/assets/d5dd6c09-14f3-40f8-8b6d-91e26519b8ac" width="100%" controls autoplay loop></video>
</td>
<td>
<video src="https://github.com/user-attachments/assets/9327e8bc-4f17-46b0-b50d-38c250a9483a" width="100%" controls autoplay loop></video>
</td>
</tr>
</table>
Resolution-512
<table border="0" style="width: 100%; text-align: left; margin-top: 20px;">
<tr>
<td>
<video src="https://github.com/user-attachments/assets/ef407030-8062-454d-aba3-131c21e6b58c" width="100%" controls autoplay loop></video>
</td>
<td>
<video src="https://github.com/user-attachments/assets/7610f49e-38b6-4214-aa48-723ae4d1b07e" width="100%" controls autoplay loop></video>
</td>
<td>
<video src="https://github.com/user-attachments/assets/1fff0567-1e15-415c-941e-53ee8ae2c841" width="100%" controls autoplay loop></video>
</td>
<td>
<video src="https://github.com/user-attachments/assets/bcec48da-b91b-43a0-9d50-cf026e00fa4f" width="100%" controls autoplay loop></video>
</td>
</tr>
</table>
### CogVideoX-Fun-V1.1-5B-Pose
<table border="0" style="width: 100%; text-align: left; margin-top: 20px;">
<tr>
<td>
Resolution-512
</td>
<td>
Resolution-768
</td>
<td>
Resolution-1024
</td>
<tr>
<td>
<video src="https://github.com/user-attachments/assets/a746df51-9eb7-4446-bee5-2ee30285c143" width="100%" controls autoplay loop></video>
</td>
<td>
<video src="https://github.com/user-attachments/assets/db295245-e6aa-43be-8c81-32cb411f1473" width="100%" controls autoplay loop></video>
</td>
<td>
<video src="https://github.com/user-attachments/assets/ec9875b2-fde0-48e1-ab7e-490cee51ef40" width="100%" controls autoplay loop></video>
</td>
</tr>
</table>
### CogVideoX-Fun-V1.1-2B
Resolution-768
<table border="0" style="width: 100%; text-align: left; margin-top: 20px;">
<tr>
<td>
<video src="https://github.com/user-attachments/assets/03235dea-980e-4fc5-9c41-e40a5bc1b6d0" width="100%" controls autoplay loop></video>
</td>
<td>
<video src="https://github.com/user-attachments/assets/f7302648-5017-47db-bdeb-4d893e620b37" width="100%" controls autoplay loop></video>
</td>
<td>
<video src="https://github.com/user-attachments/assets/cbadf411-28fa-4b87-813d-da63ff481904" width="100%" controls autoplay loop></video>
</td>
<td>
<video src="https://github.com/user-attachments/assets/87cc9d0b-b6fe-4d2d-b447-174513d169ab" width="100%" controls autoplay loop></video>
</td>
</tr>
</table>
### CogVideoX-Fun-V1.1-2B-Pose
<table border="0" style="width: 100%; text-align: left; margin-top: 20px;">
<tr>
<td>
Resolution-512
</td>
<td>
Resolution-768
</td>
<td>
Resolution-1024
</td>
<tr>
<td>
<video src="https://github.com/user-attachments/assets/487bcd7b-1b7f-4bb4-95b5-96a6b6548b3e" width="100%" controls autoplay loop></video>
</td>
<td>
<video src="https://github.com/user-attachments/assets/2710fd18-8489-46e4-8086-c237309ae7f6" width="100%" controls autoplay loop></video>
</td>
<td>
<video src="https://github.com/user-attachments/assets/b79513db-7747-4512-b86c-94f9ca447fe2" width="100%" controls autoplay loop></video>
</td>
</tr>
</table>
# 如何使用
<h3 id="video-gen">1. 生成 </h3>
#### a. 视频生成
##### i、运行python文件
- 步骤1:下载对应[权重](#model-zoo)放入models文件夹。
- 步骤2:在predict_t2v.py文件中修改prompt、neg_prompt、guidance_scale和seed。
- 步骤3:运行predict_t2v.py文件,等待生成结果,结果保存在samples/cogvideox-fun-videos-t2v文件夹中。
- 步骤4:如果想结合自己训练的其他backbone与Lora,则看情况修改predict_t2v.py中的predict_t2v.py和lora_path。
##### ii、通过ui界面
- 步骤1:下载对应[权重](#model-zoo)放入models文件夹。
- 步骤2:运行app.py文件,进入gradio页面。
- 步骤3:根据页面选择生成模型,填入prompt、neg_prompt、guidance_scale和seed等,点击生成,等待生成结果,结果保存在sample文件夹中。
##### iii、通过comfyui
具体查看[ComfyUI README](comfyui/README.md)。
### 2. 模型训练
一个完整的CogVideoX-Fun训练链路应该包括数据预处理和Video DiT训练。
<h4 id="data-preprocess">a.数据预处理</h4>
我们给出了一个简单的demo通过图片数据训练lora模型,详情可以查看[wiki](https://github.com/aigc-apps/CogVideoX-Fun/wiki/Training-Lora)。
一个完整的长视频切分、清洗、描述的数据预处理链路可以参考video caption部分的[README](cogvideox/video_caption/README.md)进行。
如果期望训练一个文生图视频的生成模型,您需要以这种格式排列数据集。
```
📦 project/
├── 📂 datasets/
│ ├── 📂 internal_datasets/
│ ├── 📂 train/
│ │ ├── 📄 00000001.mp4
│ │ ├── 📄 00000002.jpg
│ │ └── 📄 .....
│ └── 📄 json_of_internal_datasets.json
```
json_of_internal_datasets.json是一个标准的json文件。json中的file_path可以被设置为相对路径,如下所示:
```json
[
{
"file_path": "train/00000001.mp4",
"text": "A group of young men in suits and sunglasses are walking down a city street.",
"type": "video"
},
{
"file_path": "train/00000002.jpg",
"text": "A group of young men in suits and sunglasses are walking down a city street.",
"type": "image"
},
.....
]
```
你也可以将路径设置为绝对路径:
```json
[
{
"file_path": "/mnt/data/videos/00000001.mp4",
"text": "A group of young men in suits and sunglasses are walking down a city street.",
"type": "video"
},
{
"file_path": "/mnt/data/train/00000001.jpg",
"text": "A group of young men in suits and sunglasses are walking down a city street.",
"type": "image"
},
.....
]
```
<h4 id="dit-train">b. Video DiT训练 </h4>
如果数据预处理时,数据的格式为相对路径,则进入scripts/train.sh进行如下设置。
```
export DATASET_NAME="datasets/internal_datasets/"
export DATASET_META_NAME="datasets/internal_datasets/json_of_internal_datasets.json"
...
train_data_format="normal"
```
如果数据的格式为绝对路径,则进入scripts/train.sh进行如下设置。
```
export DATASET_NAME=""
export DATASET_META_NAME="/mnt/data/json_of_internal_datasets.json"
```
最后运行scripts/train.sh。
```sh
sh scripts/train.sh
```
关于一些参数的设置细节,可以查看[Readme Train](scripts/README_TRAIN.md)与[Readme Lora](scripts/README_TRAIN_LORA.md)
# 模型地址
V1.1:
| 名称 | 存储空间 | Hugging Face | Model Scope | 描述 |
|--|--|--|--|--|
| CogVideoX-Fun-V1.1-2b-InP.tar.gz | 解压前 9.7 GB / 解压后 13.0 GB | [🤗Link](https://huggingface.co/alibaba-pai/CogVideoX-Fun-V1.1-2b-InP) | [😄Link](https://modelscope.cn/models/PAI/CogVideoX-Fun-V1.1-2b-InP) | 官方的图生视频权重。添加了Noise,运动幅度相比于V1.0更大。支持多分辨率(512,768,1024,1280)的视频预测,以49帧、每秒8帧进行训练 |
| CogVideoX-Fun-V1.1-5b-InP.tar.gz | 解压前 16.0GB / 解压后 20.0 GB | [🤗Link](https://huggingface.co/alibaba-pai/CogVideoX-Fun-V1.1-5b-InP) | [😄Link](https://modelscope.cn/models/PAI/CogVideoX-Fun-V1.1-5b-InP) | 官方的图生视频权重。添加了Noise,运动幅度相比于V1.0更大。支持多分辨率(512,768,1024,1280)的视频预测,以49帧、每秒8帧进行训练 |
| CogVideoX-Fun-V1.1-2b-Pose.tar.gz | 解压前 9.7 GB / 解压后 13.0 GB | [🤗Link](https://huggingface.co/alibaba-pai/CogVideoX-Fun-V1.1-2b-Pose) | [😄Link](https://modelscope.cn/models/PAI/CogVideoX-Fun-V1.1-2b-Pose) | 官方的姿态控制生视频权重。支持多分辨率(512,768,1024,1280)的视频预测,以49帧、每秒8帧进行训练 |
| CogVideoX-Fun-V1.1-5b-Pose.tar.gz | 解压前 16.0GB / 解压后 20.0 GB | [🤗Link](https://huggingface.co/alibaba-pai/CogVideoX-Fun-V1.1-5b-Pose) | [😄Link](https://modelscope.cn/models/PAI/CogVideoX-Fun-V1.1-5b-Pose) | 官方的姿态控制生视频权重。支持多分辨率(512,768,1024,1280)的视频预测,以49帧、每秒8帧进行训练 |
V1.0:
| 名称 | 存储空间 | Hugging Face | Model Scope | 描述 |
|--|--|--|--|--|
| CogVideoX-Fun-2b-InP.tar.gz | 解压前 9.7 GB / 解压后 13.0 GB | [🤗Link](https://huggingface.co/alibaba-pai/CogVideoX-Fun-2b-InP) | [😄Link](https://modelscope.cn/models/PAI/CogVideoX-Fun-2b-InP) | 官方的图生视频权重。支持多分辨率(512,768,1024,1280)的视频预测,以49帧、每秒8帧进行训练 |
| CogVideoX-Fun-5b-InP.tar.gz | 解压前 16.0GB / 解压后 20.0 GB | [🤗Link](https://huggingface.co/alibaba-pai/CogVideoX-Fun-5b-InP) | [😄Link](https://modelscope.cn/models/PAI/CogVideoX-Fun-5b-InP) | 官方的图生视频权重。支持多分辨率(512,768,1024,1280)的视频预测,以49帧、每秒8帧进行训练 |
# 未来计划
- 支持中文。
# 参考文献
- CogVideo: https://github.com/THUDM/CogVideo/
- EasyAnimate: https://github.com/aigc-apps/EasyAnimate
# 许可证
本项目采用 [Apache License (Version 2.0)](https://github.com/modelscope/modelscope/blob/master/LICENSE).
CogVideoX-2B 模型 (包括其对应的Transformers模块,VAE模块) 根据 [Apache 2.0 协议](LICENSE) 许可证发布。
CogVideoX-5B 模型(Transformer 模块)在[CogVideoX许可证](https://huggingface.co/THUDM/CogVideoX-5b/blob/main/LICENSE)下发布.
|