Upload README.md with huggingface_hub
Browse files
README.md
CHANGED
@@ -1,198 +1,215 @@
|
|
1 |
---
|
|
|
|
|
|
|
|
|
|
|
|
|
2 |
library_name: diffusers
|
|
|
|
|
|
|
|
|
|
|
|
|
|
|
|
|
|
|
|
|
|
|
|
|
|
|
|
|
3 |
---
|
4 |
|
5 |
-
#
|
6 |
|
7 |
-
|
8 |
|
|
|
9 |
|
|
|
10 |
|
11 |
-
|
12 |
-
|
13 |
-
### Model Description
|
14 |
-
|
15 |
-
<!-- Provide a longer summary of what this model is. -->
|
16 |
-
|
17 |
-
This is the model card of a 🧨 diffusers model that has been pushed on the Hub. This model card has been automatically generated.
|
18 |
-
|
19 |
-
- **Developed by:** [More Information Needed]
|
20 |
-
- **Funded by [optional]:** [More Information Needed]
|
21 |
-
- **Shared by [optional]:** [More Information Needed]
|
22 |
-
- **Model type:** [More Information Needed]
|
23 |
-
- **Language(s) (NLP):** [More Information Needed]
|
24 |
-
- **License:** [More Information Needed]
|
25 |
-
- **Finetuned from model [optional]:** [More Information Needed]
|
26 |
-
|
27 |
-
### Model Sources [optional]
|
28 |
-
|
29 |
-
<!-- Provide the basic links for the model. -->
|
30 |
-
|
31 |
-
- **Repository:** [More Information Needed]
|
32 |
-
- **Paper [optional]:** [More Information Needed]
|
33 |
-
- **Demo [optional]:** [More Information Needed]
|
34 |
-
|
35 |
-
## Uses
|
36 |
-
|
37 |
-
<!-- Address questions around how the model is intended to be used, including the foreseeable users of the model and those affected by the model. -->
|
38 |
-
|
39 |
-
### Direct Use
|
40 |
-
|
41 |
-
<!-- This section is for the model use without fine-tuning or plugging into a larger ecosystem/app. -->
|
42 |
-
|
43 |
-
[More Information Needed]
|
44 |
-
|
45 |
-
### Downstream Use [optional]
|
46 |
-
|
47 |
-
<!-- This section is for the model use when fine-tuned for a task, or when plugged into a larger ecosystem/app -->
|
48 |
-
|
49 |
-
[More Information Needed]
|
50 |
-
|
51 |
-
### Out-of-Scope Use
|
52 |
-
|
53 |
-
<!-- This section addresses misuse, malicious use, and uses that the model will not work well for. -->
|
54 |
-
|
55 |
-
[More Information Needed]
|
56 |
-
|
57 |
-
## Bias, Risks, and Limitations
|
58 |
-
|
59 |
-
<!-- This section is meant to convey both technical and sociotechnical limitations. -->
|
60 |
-
|
61 |
-
[More Information Needed]
|
62 |
-
|
63 |
-
### Recommendations
|
64 |
-
|
65 |
-
<!-- This section is meant to convey recommendations with respect to the bias, risk, and technical limitations. -->
|
66 |
-
|
67 |
-
Users (both direct and downstream) should be made aware of the risks, biases and limitations of the model. More information needed for further recommendations.
|
68 |
-
|
69 |
-
## How to Get Started with the Model
|
70 |
-
|
71 |
-
Use the code below to get started with the model.
|
72 |
-
|
73 |
-
[More Information Needed]
|
74 |
-
|
75 |
-
## Training Details
|
76 |
-
|
77 |
-
### Training Data
|
78 |
-
|
79 |
-
<!-- This should link to a Dataset Card, perhaps with a short stub of information on what the training data is all about as well as documentation related to data pre-processing or additional filtering. -->
|
80 |
-
|
81 |
-
[More Information Needed]
|
82 |
-
|
83 |
-
### Training Procedure
|
84 |
-
|
85 |
-
<!-- This relates heavily to the Technical Specifications. Content here should link to that section when it is relevant to the training procedure. -->
|
86 |
-
|
87 |
-
#### Preprocessing [optional]
|
88 |
-
|
89 |
-
[More Information Needed]
|
90 |
-
|
91 |
-
|
92 |
-
#### Training Hyperparameters
|
93 |
|
94 |
-
|
|
|
95 |
|
96 |
-
|
|
|
97 |
|
98 |
-
|
99 |
|
100 |
-
|
|
|
|
|
|
|
101 |
|
102 |
-
|
|
|
|
|
|
|
|
|
103 |
|
104 |
-
|
|
|
|
|
|
|
|
|
105 |
|
106 |
-
|
107 |
|
108 |
-
#### Testing Data
|
109 |
-
|
110 |
-
<!-- This should link to a Dataset Card if possible. -->
|
111 |
-
|
112 |
-
[More Information Needed]
|
113 |
-
|
114 |
-
#### Factors
|
115 |
-
|
116 |
-
<!-- These are the things the evaluation is disaggregating by, e.g., subpopulations or domains. -->
|
117 |
-
|
118 |
-
[More Information Needed]
|
119 |
-
|
120 |
-
#### Metrics
|
121 |
-
|
122 |
-
<!-- These are the evaluation metrics being used, ideally with a description of why. -->
|
123 |
-
|
124 |
-
[More Information Needed]
|
125 |
-
|
126 |
-
### Results
|
127 |
-
|
128 |
-
[More Information Needed]
|
129 |
-
|
130 |
-
#### Summary
|
131 |
-
|
132 |
-
|
133 |
-
|
134 |
-
## Model Examination [optional]
|
135 |
-
|
136 |
-
<!-- Relevant interpretability work for the model goes here -->
|
137 |
-
|
138 |
-
[More Information Needed]
|
139 |
|
|
|
|
|
|
|
|
|
|
|
|
|
|
|
|
|
|
|
|
|
|
|
|
|
|
|
|
|
|
|
|
|
|
|
|
|
|
|
|
|
|
|
|
|
|
|
|
|
|
|
|
|
|
|
|
|
|
|
|
|
|
|
|
|
|
|
|
|
|
|
|
|
|
|
|
|
|
|
|
|
|
|
|
|
|
|
|
|
|
|
|
|
|
|
|
|
|
|
|
|
|
|
|
|
|
|
|
|
|
|
|
|
|
|
|
|
|
|
|
|
|
|
|
|
|
|
|
|
|
|
|
|
|
|
|
|
|
|
|
|
|
|
|
|
|
|
|
|
|
|
|
|
|
|
|
|
|
|
|
|
|
|
|
|
|
|
|
|
|
|
|
|
|
|
|
|
|
|
|
|
|
|
|
|
|
|
|
|
|
|
|
|
|
|
|
|
|
|
|
|
|
|
|
|
|
|
|
|
|
|
|
|
|
|
|
|
|
|
|
|
|
|
|
|
|
|
|
|
|
|
|
|
|
|
|
|
|
|
|
|
|
|
|
|
|
|
|
|
|
|
|
|
|
|
140 |
## Environmental Impact
|
141 |
|
142 |
-
|
143 |
-
|
144 |
-
Carbon emissions can be estimated using the [Machine Learning Impact calculator](https://mlco2.github.io/impact#compute) presented in [Lacoste et al. (2019)](https://arxiv.org/abs/1910.09700).
|
145 |
-
|
146 |
-
- **Hardware Type:** [More Information Needed]
|
147 |
-
- **Hours used:** [More Information Needed]
|
148 |
-
- **Cloud Provider:** [More Information Needed]
|
149 |
-
- **Compute Region:** [More Information Needed]
|
150 |
-
- **Carbon Emitted:** [More Information Needed]
|
151 |
-
|
152 |
-
## Technical Specifications [optional]
|
153 |
-
|
154 |
-
### Model Architecture and Objective
|
155 |
-
|
156 |
-
[More Information Needed]
|
157 |
-
|
158 |
-
### Compute Infrastructure
|
159 |
-
|
160 |
-
[More Information Needed]
|
161 |
-
|
162 |
-
#### Hardware
|
163 |
-
|
164 |
-
[More Information Needed]
|
165 |
-
|
166 |
-
#### Software
|
167 |
-
|
168 |
-
[More Information Needed]
|
169 |
-
|
170 |
-
## Citation [optional]
|
171 |
-
|
172 |
-
<!-- If there is a paper or blog post introducing the model, the APA and Bibtex information for that should go in this section. -->
|
173 |
-
|
174 |
-
**BibTeX:**
|
175 |
-
|
176 |
-
[More Information Needed]
|
177 |
-
|
178 |
-
**APA:**
|
179 |
-
|
180 |
-
[More Information Needed]
|
181 |
-
|
182 |
-
## Glossary [optional]
|
183 |
-
|
184 |
-
<!-- If relevant, include terms and calculations in this section that can help readers understand the model or model card. -->
|
185 |
-
|
186 |
-
[More Information Needed]
|
187 |
-
|
188 |
-
## More Information [optional]
|
189 |
|
190 |
-
|
|
|
|
|
|
|
|
|
191 |
|
192 |
-
## Model Card Authors [optional]
|
193 |
|
194 |
-
|
195 |
|
196 |
-
|
|
|
|
|
|
|
|
|
|
|
|
|
|
|
|
|
|
|
197 |
|
198 |
-
[
|
|
|
1 |
---
|
2 |
+
license: creativeml-openrail-m
|
3 |
+
tags:
|
4 |
+
- stable-diffusion
|
5 |
+
- stable-diffusion-diffusers
|
6 |
+
- text-to-image
|
7 |
+
inference: false
|
8 |
library_name: diffusers
|
9 |
+
extra_gated_prompt: |-
|
10 |
+
One more step before getting this model.
|
11 |
+
This model is open access and available to all, with a CreativeML OpenRAIL-M license further specifying rights and usage.
|
12 |
+
The CreativeML OpenRAIL License specifies:
|
13 |
+
|
14 |
+
1. You can't use the model to deliberately produce nor share illegal or harmful outputs or content
|
15 |
+
2. CompVis claims no rights on the outputs you generate, you are free to use them and are accountable for their use which must not go against the provisions set in the license
|
16 |
+
3. You may re-distribute the weights and use the model commercially and/or as a service. If you do, please be aware you have to include the same use restrictions as the ones in the license and share a copy of the CreativeML OpenRAIL-M to all your users (please read the license entirely and carefully)
|
17 |
+
Please read the full license here: https://huggingface.co/spaces/CompVis/stable-diffusion-license
|
18 |
+
|
19 |
+
By clicking on "Access repository" below, you accept that your *contact information* (email address and username) can be shared with the model authors as well.
|
20 |
+
|
21 |
+
extra_gated_fields:
|
22 |
+
I have read the License and agree with its terms: checkbox
|
23 |
---
|
24 |
|
25 |
+
# Re-upload
|
26 |
|
27 |
+
This repository is being re-uploaded to HuggingFace in accordance with [The CreativeML OpenRAIL-M License](https://huggingface.co/spaces/CompVis/stable-diffusion-license) under which this repository was originally uploaded, specifically **Section II** which grants:
|
28 |
|
29 |
+
> ...a perpetual, worldwide, non-exclusive, no-charge, royalty-free, irrevocable copyright license to reproduce, prepare, publicly display, publicly perform, sublicense, and distribute the Complementary Material, the Model, and Derivatives of the Model.
|
30 |
|
31 |
+
Note that these files did not come from HuggingFace, but instead from [modelscope](https://www.modelscope.cn/models/AI-ModelScope/stable-diffusion-v1-5). As such, there may be modifications to the weights that are unknown. If you have copies of the original weights and would be willing to perform a checksum for me to be sure, I'd be grateful - [Make a post in the Community tab](https://huggingface.co/benjamin-paine/stable-diffusion-v1-5/discussions) if you can.
|
32 |
|
33 |
+
# Stable Diffusion v1-5 Model Card
|
|
|
|
|
|
|
|
|
|
|
|
|
|
|
|
|
|
|
|
|
|
|
|
|
|
|
|
|
|
|
|
|
|
|
|
|
|
|
|
|
|
|
|
|
|
|
|
|
|
|
|
|
|
|
|
|
|
|
|
|
|
|
|
|
|
|
|
|
|
|
|
|
|
|
|
|
|
|
|
|
|
|
|
|
|
|
|
|
|
|
|
|
|
|
|
|
|
|
|
|
|
|
|
|
|
|
|
|
|
|
|
|
|
|
|
|
|
|
|
|
|
|
|
|
|
|
|
|
|
|
|
|
|
|
|
|
|
|
|
|
|
|
|
|
|
|
|
|
|
|
|
|
|
|
|
|
|
|
|
|
|
|
34 |
|
35 |
+
Stable Diffusion is a latent text-to-image diffusion model capable of generating photo-realistic images given any text input.
|
36 |
+
For more information about how Stable Diffusion functions, please have a look at [🤗's Stable Diffusion blog](https://huggingface.co/blog/stable_diffusion).
|
37 |
|
38 |
+
The **Stable-Diffusion-v1-5** checkpoint was initialized with the weights of the [Stable-Diffusion-v1-2](https:/steps/huggingface.co/CompVis/stable-diffusion-v1-2)
|
39 |
+
checkpoint and subsequently fine-tuned on 595k steps at resolution 512x512 on "laion-aesthetics v2 5+" and 10% dropping of the text-conditioning to improve [classifier-free guidance sampling](https://arxiv.org/abs/2207.12598).
|
40 |
|
41 |
+
You can use this with the [🧨Diffusers library](https://github.com/huggingface/diffusers).
|
42 |
|
43 |
+
### Diffusers usage
|
44 |
+
```py
|
45 |
+
from diffusers import StableDiffusionPipeline
|
46 |
+
import torch
|
47 |
|
48 |
+
pipe = StableDiffusionPipeline.from_pretrained(
|
49 |
+
"benjamin-paine/stable-diffusion-v1-5",
|
50 |
+
torch_dtype=torch.float16
|
51 |
+
)
|
52 |
+
pipe = pipe.to("cuda")
|
53 |
|
54 |
+
prompt = "a photo of an astronaut riding a horse on mars"
|
55 |
+
image = pipe(prompt).images[0]
|
56 |
+
|
57 |
+
image.save("astronaut_rides_horse.png")
|
58 |
+
```
|
59 |
|
60 |
+
For more detailed instructions, use-cases and examples in JAX follow the instructions [here](https://github.com/huggingface/diffusers#text-to-image-generation-with-stable-diffusion)
|
61 |
|
|
|
|
|
|
|
|
|
|
|
|
|
|
|
|
|
|
|
|
|
|
|
|
|
|
|
|
|
|
|
|
|
|
|
|
|
|
|
|
|
|
|
|
|
|
|
|
|
|
|
|
|
|
|
|
|
|
|
|
|
|
|
62 |
|
63 |
+
## Model Details
|
64 |
+
- **Developed by:** Robin Rombach, Patrick Esser
|
65 |
+
- **Model type:** Diffusion-based text-to-image generation model
|
66 |
+
- **Language(s):** English
|
67 |
+
- **License:** [The CreativeML OpenRAIL M license](https://huggingface.co/spaces/CompVis/stable-diffusion-license) is an [Open RAIL M license](https://www.licenses.ai/blog/2022/8/18/naming-convention-of-responsible-ai-licenses), adapted from the work that [BigScience](https://bigscience.huggingface.co/) and [the RAIL Initiative](https://www.licenses.ai/) are jointly carrying in the area of responsible AI licensing. See also [the article about the BLOOM Open RAIL license](https://bigscience.huggingface.co/blog/the-bigscience-rail-license) on which our license is based.
|
68 |
+
- **Model Description:** This is a model that can be used to generate and modify images based on text prompts. It is a [Latent Diffusion Model](https://arxiv.org/abs/2112.10752) that uses a fixed, pretrained text encoder ([CLIP ViT-L/14](https://arxiv.org/abs/2103.00020)) as suggested in the [Imagen paper](https://arxiv.org/abs/2205.11487).
|
69 |
+
- **Resources for more information:** [GitHub Repository](https://github.com/CompVis/stable-diffusion), [Paper](https://arxiv.org/abs/2112.10752).
|
70 |
+
- **Cite as:**
|
71 |
+
|
72 |
+
@InProceedings{Rombach_2022_CVPR,
|
73 |
+
author = {Rombach, Robin and Blattmann, Andreas and Lorenz, Dominik and Esser, Patrick and Ommer, Bj\"orn},
|
74 |
+
title = {High-Resolution Image Synthesis With Latent Diffusion Models},
|
75 |
+
booktitle = {Proceedings of the IEEE/CVF Conference on Computer Vision and Pattern Recognition (CVPR)},
|
76 |
+
month = {June},
|
77 |
+
year = {2022},
|
78 |
+
pages = {10684-10695}
|
79 |
+
}
|
80 |
+
|
81 |
+
# Uses
|
82 |
+
|
83 |
+
## Direct Use
|
84 |
+
The model is intended for research purposes only. Possible research areas and
|
85 |
+
tasks include
|
86 |
+
|
87 |
+
- Safe deployment of models which have the potential to generate harmful content.
|
88 |
+
- Probing and understanding the limitations and biases of generative models.
|
89 |
+
- Generation of artworks and use in design and other artistic processes.
|
90 |
+
- Applications in educational or creative tools.
|
91 |
+
- Research on generative models.
|
92 |
+
|
93 |
+
Excluded uses are described below.
|
94 |
+
|
95 |
+
### Misuse, Malicious Use, and Out-of-Scope Use
|
96 |
+
_Note: This section is taken from the [DALLE-MINI model card](https://huggingface.co/dalle-mini/dalle-mini), but applies in the same way to Stable Diffusion v1_.
|
97 |
+
|
98 |
+
|
99 |
+
The model should not be used to intentionally create or disseminate images that create hostile or alienating environments for people. This includes generating images that people would foreseeably find disturbing, distressing, or offensive; or content that propagates historical or current stereotypes.
|
100 |
+
|
101 |
+
#### Out-of-Scope Use
|
102 |
+
The model was not trained to be factual or true representations of people or events, and therefore using the model to generate such content is out-of-scope for the abilities of this model.
|
103 |
+
|
104 |
+
#### Misuse and Malicious Use
|
105 |
+
Using the model to generate content that is cruel to individuals is a misuse of this model. This includes, but is not limited to:
|
106 |
+
|
107 |
+
- Generating demeaning, dehumanizing, or otherwise harmful representations of people or their environments, cultures, religions, etc.
|
108 |
+
- Intentionally promoting or propagating discriminatory content or harmful stereotypes.
|
109 |
+
- Impersonating individuals without their consent.
|
110 |
+
- Sexual content without consent of the people who might see it.
|
111 |
+
- Mis- and disinformation
|
112 |
+
- Representations of egregious violence and gore
|
113 |
+
- Sharing of copyrighted or licensed material in violation of its terms of use.
|
114 |
+
- Sharing content that is an alteration of copyrighted or licensed material in violation of its terms of use.
|
115 |
+
|
116 |
+
## Limitations and Bias
|
117 |
+
|
118 |
+
### Limitations
|
119 |
+
|
120 |
+
- The model does not achieve perfect photorealism
|
121 |
+
- The model cannot render legible text
|
122 |
+
- The model does not perform well on more difficult tasks which involve compositionality, such as rendering an image corresponding to “A red cube on top of a blue sphere”
|
123 |
+
- Faces and people in general may not be generated properly.
|
124 |
+
- The model was trained mainly with English captions and will not work as well in other languages.
|
125 |
+
- The autoencoding part of the model is lossy
|
126 |
+
- The model was trained on a large-scale dataset
|
127 |
+
[LAION-5B](https://laion.ai/blog/laion-5b/) which contains adult material
|
128 |
+
and is not fit for product use without additional safety mechanisms and
|
129 |
+
considerations.
|
130 |
+
- No additional measures were used to deduplicate the dataset. As a result, we observe some degree of memorization for images that are duplicated in the training data.
|
131 |
+
The training data can be searched at [https://rom1504.github.io/clip-retrieval/](https://rom1504.github.io/clip-retrieval/) to possibly assist in the detection of memorized images.
|
132 |
+
|
133 |
+
### Bias
|
134 |
+
|
135 |
+
While the capabilities of image generation models are impressive, they can also reinforce or exacerbate social biases.
|
136 |
+
Stable Diffusion v1 was trained on subsets of [LAION-2B(en)](https://laion.ai/blog/laion-5b/),
|
137 |
+
which consists of images that are primarily limited to English descriptions.
|
138 |
+
Texts and images from communities and cultures that use other languages are likely to be insufficiently accounted for.
|
139 |
+
This affects the overall output of the model, as white and western cultures are often set as the default. Further, the
|
140 |
+
ability of the model to generate content with non-English prompts is significantly worse than with English-language prompts.
|
141 |
+
|
142 |
+
### Safety Module
|
143 |
+
|
144 |
+
The intended use of this model is with the [Safety Checker](https://github.com/huggingface/diffusers/blob/main/src/diffusers/pipelines/stable_diffusion/safety_checker.py) in Diffusers.
|
145 |
+
This checker works by checking model outputs against known hard-coded NSFW concepts.
|
146 |
+
The concepts are intentionally hidden to reduce the likelihood of reverse-engineering this filter.
|
147 |
+
Specifically, the checker compares the class probability of harmful concepts in the embedding space of the `CLIPTextModel` *after generation* of the images.
|
148 |
+
The concepts are passed into the model with the generated image and compared to a hand-engineered weight for each NSFW concept.
|
149 |
+
|
150 |
+
## Training
|
151 |
+
|
152 |
+
**Training Data**
|
153 |
+
The model developers used the following dataset for training the model:
|
154 |
+
|
155 |
+
- LAION-2B (en) and subsets thereof (see next section)
|
156 |
+
|
157 |
+
**Training Procedure**
|
158 |
+
Stable Diffusion v1-5 is a latent diffusion model which combines an autoencoder with a diffusion model that is trained in the latent space of the autoencoder. During training,
|
159 |
+
|
160 |
+
- Images are encoded through an encoder, which turns images into latent representations. The autoencoder uses a relative downsampling factor of 8 and maps images of shape H x W x 3 to latents of shape H/f x W/f x 4
|
161 |
+
- Text prompts are encoded through a ViT-L/14 text-encoder.
|
162 |
+
- The non-pooled output of the text encoder is fed into the UNet backbone of the latent diffusion model via cross-attention.
|
163 |
+
- The loss is a reconstruction objective between the noise that was added to the latent and the prediction made by the UNet.
|
164 |
+
|
165 |
+
Currently six Stable Diffusion checkpoints are provided, which were trained as follows.
|
166 |
+
- [`stable-diffusion-v1-1`](https://huggingface.co/CompVis/stable-diffusion-v1-1): 237,000 steps at resolution `256x256` on [laion2B-en](https://huggingface.co/datasets/laion/laion2B-en).
|
167 |
+
194,000 steps at resolution `512x512` on [laion-high-resolution](https://huggingface.co/datasets/laion/laion-high-resolution) (170M examples from LAION-5B with resolution `>= 1024x1024`).
|
168 |
+
- [`stable-diffusion-v1-2`](https://huggingface.co/CompVis/stable-diffusion-v1-2): Resumed from `stable-diffusion-v1-1`.
|
169 |
+
515,000 steps at resolution `512x512` on "laion-improved-aesthetics" (a subset of laion2B-en,
|
170 |
+
filtered to images with an original size `>= 512x512`, estimated aesthetics score `> 5.0`, and an estimated watermark probability `< 0.5`. The watermark estimate is from the LAION-5B metadata, the aesthetics score is estimated using an [improved aesthetics estimator](https://github.com/christophschuhmann/improved-aesthetic-predictor)).
|
171 |
+
- [`stable-diffusion-v1-3`](https://huggingface.co/CompVis/stable-diffusion-v1-3): Resumed from `stable-diffusion-v1-2` - 195,000 steps at resolution `512x512` on "laion-improved-aesthetics" and 10 % dropping of the text-conditioning to improve [classifier-free guidance sampling](https://arxiv.org/abs/2207.12598).
|
172 |
+
- [`stable-diffusion-v1-4`](https://huggingface.co/CompVis/stable-diffusion-v1-4) Resumed from `stable-diffusion-v1-2` - 225,000 steps at resolution `512x512` on "laion-aesthetics v2 5+" and 10 % dropping of the text-conditioning to improve [classifier-free guidance sampling](https://arxiv.org/abs/2207.12598).
|
173 |
+
- [`stable-diffusion-v1-5`](https://huggingface.co/benjamin-paine/stable-diffusion-v1-5) Resumed from `stable-diffusion-v1-2` - 595,000 steps at resolution `512x512` on "laion-aesthetics v2 5+" and 10 % dropping of the text-conditioning to improve [classifier-free guidance sampling](https://arxiv.org/abs/2207.12598).
|
174 |
+
- [`stable-diffusion-inpainting`](https://huggingface.co/benjamin-paine/stable-diffusion-inpainting) Resumed from `stable-diffusion-v1-5` - then 440,000 steps of inpainting training at resolution 512x512 on “laion-aesthetics v2 5+” and 10% dropping of the text-conditioning. For inpainting, the UNet has 5 additional input channels (4 for the encoded masked-image and 1 for the mask itself) whose weights were zero-initialized after restoring the non-inpainting checkpoint. During training, we generate synthetic masks and in 25% mask everything.
|
175 |
+
|
176 |
+
- **Hardware:** 32 x 8 x A100 GPUs
|
177 |
+
- **Optimizer:** AdamW
|
178 |
+
- **Gradient Accumulations**: 2
|
179 |
+
- **Batch:** 32 x 8 x 2 x 4 = 2048
|
180 |
+
- **Learning rate:** warmup to 0.0001 for 10,000 steps and then kept constant
|
181 |
+
|
182 |
+
## Evaluation Results
|
183 |
+
Evaluations with different classifier-free guidance scales (1.5, 2.0, 3.0, 4.0,
|
184 |
+
5.0, 6.0, 7.0, 8.0) and 50 PNDM/PLMS sampling
|
185 |
+
steps show the relative improvements of the checkpoints:
|
186 |
+
|
187 |
+
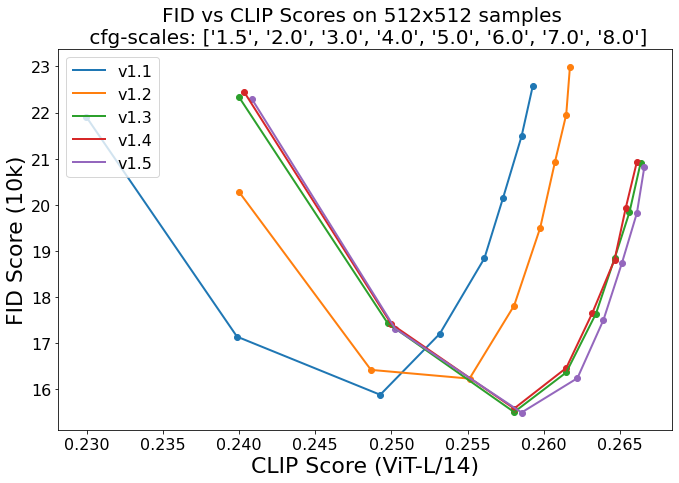
|
188 |
+
|
189 |
+
Evaluated using 50 PLMS steps and 10000 random prompts from the COCO2017 validation set, evaluated at 512x512 resolution. Not optimized for FID scores.
|
190 |
## Environmental Impact
|
191 |
|
192 |
+
**Stable Diffusion v1** **Estimated Emissions**
|
193 |
+
Based on that information, we estimate the following CO2 emissions using the [Machine Learning Impact calculator](https://mlco2.github.io/impact#compute) presented in [Lacoste et al. (2019)](https://arxiv.org/abs/1910.09700). The hardware, runtime, cloud provider, and compute region were utilized to estimate the carbon impact.
|
|
|
|
|
|
|
|
|
|
|
|
|
|
|
|
|
|
|
|
|
|
|
|
|
|
|
|
|
|
|
|
|
|
|
|
|
|
|
|
|
|
|
|
|
|
|
|
|
|
|
|
|
|
|
|
|
|
|
|
|
|
|
|
|
|
|
|
|
|
|
|
|
|
|
|
|
|
|
|
|
|
|
|
|
|
|
|
|
|
|
194 |
|
195 |
+
- **Hardware Type:** A100 PCIe 40GB
|
196 |
+
- **Hours used:** 150000
|
197 |
+
- **Cloud Provider:** AWS
|
198 |
+
- **Compute Region:** US-east
|
199 |
+
- **Carbon Emitted (Power consumption x Time x Carbon produced based on location of power grid):** 11250 kg CO2 eq.
|
200 |
|
|
|
201 |
|
202 |
+
## Citation
|
203 |
|
204 |
+
```bibtex
|
205 |
+
@InProceedings{Rombach_2022_CVPR,
|
206 |
+
author = {Rombach, Robin and Blattmann, Andreas and Lorenz, Dominik and Esser, Patrick and Ommer, Bj\"orn},
|
207 |
+
title = {High-Resolution Image Synthesis With Latent Diffusion Models},
|
208 |
+
booktitle = {Proceedings of the IEEE/CVF Conference on Computer Vision and Pattern Recognition (CVPR)},
|
209 |
+
month = {June},
|
210 |
+
year = {2022},
|
211 |
+
pages = {10684-10695}
|
212 |
+
}
|
213 |
+
```
|
214 |
|
215 |
+
*This model card was written by: Robin Rombach and Patrick Esser and is based on the [DALL-E Mini model card](https://huggingface.co/dalle-mini/dalle-mini).*
|