We have also had good success applying tts more broadly at a diverse set of tasks in optillm - https://github.com/codelion/optillm
Asankhaya Sharma
codelion
AI & ML interests
AI/ML, Dev Tools and Application Security
Recent Activity
upvoted
a
paper
about 14 hours ago
Native Sparse Attention: Hardware-Aligned and Natively Trainable Sparse
Attention
upvoted
a
paper
about 19 hours ago
SWE-Lancer: Can Frontier LLMs Earn $1 Million from Real-World Freelance
Software Engineering?
updated
a Space
1 day ago
codelion/responsive-image-generator
Organizations
codelion's activity
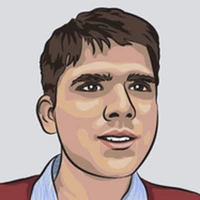
reacted to
Kseniase's
post with ππ₯
2 days ago
Post
2838
8 New Applications of Test-Time Scaling
We've noticed a huge interest in test-time scaling (TTS), so we decided to explore this concept further. Test-time compute (TTC) refers to the amount of computational power used by an AI model when generating a response. Many researchers are now focused on scaling TTC, as it enables slow, deep "thinking" and step-by-step reasoning, which improves overall models' performance.
Here are 8 fresh studies on test-time scaling:
1. Scaling up Test-Time Compute with Latent Reasoning: A Recurrent Depth Approach (2502.05171)
Introduces an LM that scales TTC by reasoning in latent space instead of generating more tokens with no special training. Here, a recurrent block to processes information iteratively.
2. Generating Symbolic World Models via Test-time Scaling of Large Language Models (2502.04728)
Shows how TTS is applied to enhance model's Planning Domain Definition Language (PDDL) reasoning capabilities, which can be used to generate a symbolic world model.
3. Can 1B LLM Surpass 405B LLM? Rethinking Compute-Optimal Test-Time Scaling (2502.06703)
Analyzes optimal TTS strategies and shows how small models can outperform much larger ones.
4. Llasa: Scaling Train-Time and Inference-Time Compute for Llama-based Speech Synthesis (2502.04128)
Shows how TTS improves expressiveness, timbre consistency and accuracy in speech synthesis with Llasa framework. It also dives into benefits of scaling train-time compute.
5. Rethinking Fine-Tuning when Scaling Test-Time Compute: Limiting Confidence Improves Mathematical Reasoning (2502.07154)
Suggests a modified training loss for better reasoning of LLMs when scaling TTC.
6. Adaptive Graph of Thoughts: Test-Time Adaptive Reasoning Unifying Chain, Tree, and Graph Structures (2502.05078)
Unifies the strengths of chain, tree, and graph paradigms into one framework that expands reasoning only on necessary subproblems.
7. Sample, Scrutinize and Scale: Effective Inference-Time Search by Scaling Verification (2502.01839)
Explores scaling trends of self-verification and how to improve its capabilities with TTC.
8. CodeMonkeys: Scaling Test-Time Compute for Software Engineering (2501.14723)
Explores how scaling serial compute (iterations) and parallel compute (trajectories), can improve accuracy in real-world software engineering issues.
Also, explore our article about TTS for more -> https://huggingface.co/blog/Kseniase/testtimecompute
We've noticed a huge interest in test-time scaling (TTS), so we decided to explore this concept further. Test-time compute (TTC) refers to the amount of computational power used by an AI model when generating a response. Many researchers are now focused on scaling TTC, as it enables slow, deep "thinking" and step-by-step reasoning, which improves overall models' performance.
Here are 8 fresh studies on test-time scaling:
1. Scaling up Test-Time Compute with Latent Reasoning: A Recurrent Depth Approach (2502.05171)
Introduces an LM that scales TTC by reasoning in latent space instead of generating more tokens with no special training. Here, a recurrent block to processes information iteratively.
2. Generating Symbolic World Models via Test-time Scaling of Large Language Models (2502.04728)
Shows how TTS is applied to enhance model's Planning Domain Definition Language (PDDL) reasoning capabilities, which can be used to generate a symbolic world model.
3. Can 1B LLM Surpass 405B LLM? Rethinking Compute-Optimal Test-Time Scaling (2502.06703)
Analyzes optimal TTS strategies and shows how small models can outperform much larger ones.
4. Llasa: Scaling Train-Time and Inference-Time Compute for Llama-based Speech Synthesis (2502.04128)
Shows how TTS improves expressiveness, timbre consistency and accuracy in speech synthesis with Llasa framework. It also dives into benefits of scaling train-time compute.
5. Rethinking Fine-Tuning when Scaling Test-Time Compute: Limiting Confidence Improves Mathematical Reasoning (2502.07154)
Suggests a modified training loss for better reasoning of LLMs when scaling TTC.
6. Adaptive Graph of Thoughts: Test-Time Adaptive Reasoning Unifying Chain, Tree, and Graph Structures (2502.05078)
Unifies the strengths of chain, tree, and graph paradigms into one framework that expands reasoning only on necessary subproblems.
7. Sample, Scrutinize and Scale: Effective Inference-Time Search by Scaling Verification (2502.01839)
Explores scaling trends of self-verification and how to improve its capabilities with TTC.
8. CodeMonkeys: Scaling Test-Time Compute for Software Engineering (2501.14723)
Explores how scaling serial compute (iterations) and parallel compute (trajectories), can improve accuracy in real-world software engineering issues.
Also, explore our article about TTS for more -> https://huggingface.co/blog/Kseniase/testtimecompute
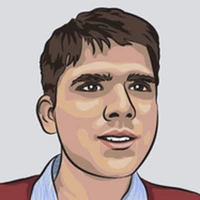
reacted to
melisa's
post with β€οΈπ₯
5 months ago
Post
3060
π₯ Introducing "Writing in the Margins (WiM)" - better inference pattern for long context LLMs that solves the Lost-in-the-Middle problem π₯
Paper page: Writing in the Margins: Better Inference Pattern for Long Context Retrieval (2408.14906)
TL;DR
Make your model write "margin notes" as you chunk prefill the KV cache. Then ask it reread all notes before it speaks up.
Works with humans, works with AI π€
WiM leverages the chunked prefill of the key-value cache, which concurrently generates query-based extractive summaries at each step of the prefill that are subsequently reintegrated at the end of the computation. We term these intermediate outputs βmarginsβ, drawing inspiration from the practice of making margin notes for improved comprehension of long contexts in human reading. We show that this technique, which adds only minimal additional computation, significantly improves LLMs long context reasoning capabilities.
Think: Every chunk has a chance to be attended to/ be at the end of the context at least once. π
π Results:
- An average accuracy boost of 7.5% in multi-hop reasoning tasks like HotpotQA and MultiHop-RAG.
- Even a 30% increase in F1-score for summarisation-like tasks (CWE).
Plus, WiM fits seamlessly into interactive applications (think: progress bar!). It can provide real-time progress updates during data retrieval and integration, making it user-friendly and transparent - a stark contrast to feeding 1mln tokens to an LLMs and waiting 6 min for the first token. π€―
π©βπ»π§βπ» Check it out and contribute to our open-source project here: https://github.com/writer/writing-in-the-margins
π§ More about chunked prefill: https://docs.vllm.ai/en/latest/models/performance.html#chunked-prefill
Paper page: Writing in the Margins: Better Inference Pattern for Long Context Retrieval (2408.14906)
TL;DR
Make your model write "margin notes" as you chunk prefill the KV cache. Then ask it reread all notes before it speaks up.
Works with humans, works with AI π€
WiM leverages the chunked prefill of the key-value cache, which concurrently generates query-based extractive summaries at each step of the prefill that are subsequently reintegrated at the end of the computation. We term these intermediate outputs βmarginsβ, drawing inspiration from the practice of making margin notes for improved comprehension of long contexts in human reading. We show that this technique, which adds only minimal additional computation, significantly improves LLMs long context reasoning capabilities.
Think: Every chunk has a chance to be attended to/ be at the end of the context at least once. π
π Results:
- An average accuracy boost of 7.5% in multi-hop reasoning tasks like HotpotQA and MultiHop-RAG.
- Even a 30% increase in F1-score for summarisation-like tasks (CWE).
Plus, WiM fits seamlessly into interactive applications (think: progress bar!). It can provide real-time progress updates during data retrieval and integration, making it user-friendly and transparent - a stark contrast to feeding 1mln tokens to an LLMs and waiting 6 min for the first token. π€―
π©βπ»π§βπ» Check it out and contribute to our open-source project here: https://github.com/writer/writing-in-the-margins
π§ More about chunked prefill: https://docs.vllm.ai/en/latest/models/performance.html#chunked-prefill
We actually recently did an independent implementation of this paper in our open-source optimizing llm proxy optillm - https://github.com/codelion/optillm/blob/main/optillm/plugins/memory_plugin.py
We were able to use it as a basis for the memory plugin in optillm that gives LLMs short term memory. It helps improve accuracy on long context retrieval and even enables LLMs to have unbounded context if needed.
We were able to match SOTA on a recent benchmark from Google Frames benchmark (https://huggingface.co/datasets/google/frames-benchmark) with only gpt-4o-mini v/s Gemini 1.5 Flash which has a context length that is 10x more.
Post
2095
We recently worked with OpenAI to fine-tune gpt-4o and built the SOTA model for the
patched-codes/static-analysis-eval benchmark. All the code and data
patched-codes/synth-vuln-fixes on how we did it is available on their GitHub - https://github.com/openai/build-hours/tree/main/5-4o_fine_tuning.
Here are some tips based on our experience:
β Establish baseline with "conditioning" / prompting
β Task-specific datasets are ideal for PEFT; hard to beat gpt-4o on "broad" tasks
β Add your best system prompt to each example
β Ensure training data distribution is similar to inference data
β Shorten instructions with concise prompts; may require more examples.
β Define clear evaluation metrics (seriously, please eval!)
You can see more details on the benchmark and process here - https://www.patched.codes/blog/the-static-analysis-evaluation-benchmark-measuring-llm-performance-in-fixing-software-vulnerabilities
Here are some tips based on our experience:
β Establish baseline with "conditioning" / prompting
β Task-specific datasets are ideal for PEFT; hard to beat gpt-4o on "broad" tasks
β Add your best system prompt to each example
β Ensure training data distribution is similar to inference data
β Shorten instructions with concise prompts; may require more examples.
β Define clear evaluation metrics (seriously, please eval!)
You can see more details on the benchmark and process here - https://www.patched.codes/blog/the-static-analysis-evaluation-benchmark-measuring-llm-performance-in-fixing-software-vulnerabilities
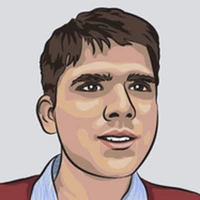
posted
an
update
6 months ago
Post
2095
We recently worked with OpenAI to fine-tune gpt-4o and built the SOTA model for the
patched-codes/static-analysis-eval benchmark. All the code and data
patched-codes/synth-vuln-fixes on how we did it is available on their GitHub - https://github.com/openai/build-hours/tree/main/5-4o_fine_tuning.
Here are some tips based on our experience:
β Establish baseline with "conditioning" / prompting
β Task-specific datasets are ideal for PEFT; hard to beat gpt-4o on "broad" tasks
β Add your best system prompt to each example
β Ensure training data distribution is similar to inference data
β Shorten instructions with concise prompts; may require more examples.
β Define clear evaluation metrics (seriously, please eval!)
You can see more details on the benchmark and process here - https://www.patched.codes/blog/the-static-analysis-evaluation-benchmark-measuring-llm-performance-in-fixing-software-vulnerabilities
Here are some tips based on our experience:
β Establish baseline with "conditioning" / prompting
β Task-specific datasets are ideal for PEFT; hard to beat gpt-4o on "broad" tasks
β Add your best system prompt to each example
β Ensure training data distribution is similar to inference data
β Shorten instructions with concise prompts; may require more examples.
β Define clear evaluation metrics (seriously, please eval!)
You can see more details on the benchmark and process here - https://www.patched.codes/blog/the-static-analysis-evaluation-benchmark-measuring-llm-performance-in-fixing-software-vulnerabilities
Post
2655
A new paper titled "STALL+: Boosting LLM-based Repository-level Code Completion with Static Analysis" shows the benefits of integrating static analysis with LLMs. (https://arxiv.org/abs/2406.10018)
Authors evaluate 4 key questions:
- How does each static analysis integration strategy perform in LLM-based repository-level code completion?
> They found that integrating static analysis in the prompting phase (especially with file-level dependencies) can achieve the substantially larger improvements than other phases.
- How do different combinations of integration strategies affect LLM-based repository-level code completion?
> Languages that are easier to analyze like Java show more improvements compared to dynamic languages like Python.
- How do static analysis integration strategies perform when compared or combined with RAG in LLM-based repository-level code completion?
> Static analysis and RAG are complementary and boost the overall accuracy.
- What are the online costs of different integration strategies in LLM-based repository-level code completion?
> Combining prompting-phase static analysis and RAG is the best option for cost-effectiveness.
In my @owasp App Sec keynote last year, I had described how one can do static analysis augmented generation (SaAG) to boost the accuracy of LLM based patches for vulnerability remediation. (you can see the talk here - https://www.youtube.com/watch?v=Cw4-ZnUNVLs)
Authors evaluate 4 key questions:
- How does each static analysis integration strategy perform in LLM-based repository-level code completion?
> They found that integrating static analysis in the prompting phase (especially with file-level dependencies) can achieve the substantially larger improvements than other phases.
- How do different combinations of integration strategies affect LLM-based repository-level code completion?
> Languages that are easier to analyze like Java show more improvements compared to dynamic languages like Python.
- How do static analysis integration strategies perform when compared or combined with RAG in LLM-based repository-level code completion?
> Static analysis and RAG are complementary and boost the overall accuracy.
- What are the online costs of different integration strategies in LLM-based repository-level code completion?
> Combining prompting-phase static analysis and RAG is the best option for cost-effectiveness.
In my @owasp App Sec keynote last year, I had described how one can do static analysis augmented generation (SaAG) to boost the accuracy of LLM based patches for vulnerability remediation. (you can see the talk here - https://www.youtube.com/watch?v=Cw4-ZnUNVLs)
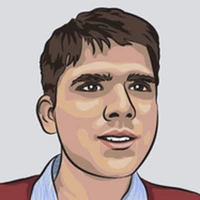
posted
an
update
8 months ago
Post
2655
A new paper titled "STALL+: Boosting LLM-based Repository-level Code Completion with Static Analysis" shows the benefits of integrating static analysis with LLMs. (https://arxiv.org/abs/2406.10018)
Authors evaluate 4 key questions:
- How does each static analysis integration strategy perform in LLM-based repository-level code completion?
> They found that integrating static analysis in the prompting phase (especially with file-level dependencies) can achieve the substantially larger improvements than other phases.
- How do different combinations of integration strategies affect LLM-based repository-level code completion?
> Languages that are easier to analyze like Java show more improvements compared to dynamic languages like Python.
- How do static analysis integration strategies perform when compared or combined with RAG in LLM-based repository-level code completion?
> Static analysis and RAG are complementary and boost the overall accuracy.
- What are the online costs of different integration strategies in LLM-based repository-level code completion?
> Combining prompting-phase static analysis and RAG is the best option for cost-effectiveness.
In my @owasp App Sec keynote last year, I had described how one can do static analysis augmented generation (SaAG) to boost the accuracy of LLM based patches for vulnerability remediation. (you can see the talk here - https://www.youtube.com/watch?v=Cw4-ZnUNVLs)
Authors evaluate 4 key questions:
- How does each static analysis integration strategy perform in LLM-based repository-level code completion?
> They found that integrating static analysis in the prompting phase (especially with file-level dependencies) can achieve the substantially larger improvements than other phases.
- How do different combinations of integration strategies affect LLM-based repository-level code completion?
> Languages that are easier to analyze like Java show more improvements compared to dynamic languages like Python.
- How do static analysis integration strategies perform when compared or combined with RAG in LLM-based repository-level code completion?
> Static analysis and RAG are complementary and boost the overall accuracy.
- What are the online costs of different integration strategies in LLM-based repository-level code completion?
> Combining prompting-phase static analysis and RAG is the best option for cost-effectiveness.
In my @owasp App Sec keynote last year, I had described how one can do static analysis augmented generation (SaAG) to boost the accuracy of LLM based patches for vulnerability remediation. (you can see the talk here - https://www.youtube.com/watch?v=Cw4-ZnUNVLs)
Post
2238
LLM-Assisted Patching of Polyfill Supply Chain Attack
A recent supply chain attack on polyfill.io affected over 100,000 websites (see https://www.patched.codes/blog/patching-the-polyfill-supply-chain-attack). To address this issue, we show how developers can leverage Large Language Models (LLMs) for efficient vulnerability patching:
1. Automated Detection: Using Semgrep rules (see https://semgrep.dev/playground/r/KxUvD7w/asankhaya_personal_org.polyfill-compromise-copy) to identify vulnerable code.
2. LLM-Powered Patching: Utilizing Patchwork (https://github.com/patched-codes/patchwork), an open-source solution that employs LLMs to automatically fix vulnerabilities.
3. Custom Workflows: The "Fixpolyfill" patchflow (https://github.com/patched-codes/patchwork-configs/tree/main/patchflows/Fixpolyfill) , tailored for this specific attack, can be easily run across multiple repositories.
4. Scalable Solutions: Options to scan and patch entire GitHub/GitLab organizations, with automated pull request generation.
5. Rapid Response: LLM-assisted patching enables swift action to minimize damage from supply chain attacks.
This approach demonstrates how LLMs can be effectively used to quickly respond to and remediate widespread security vulnerabilities in code.
A recent supply chain attack on polyfill.io affected over 100,000 websites (see https://www.patched.codes/blog/patching-the-polyfill-supply-chain-attack). To address this issue, we show how developers can leverage Large Language Models (LLMs) for efficient vulnerability patching:
1. Automated Detection: Using Semgrep rules (see https://semgrep.dev/playground/r/KxUvD7w/asankhaya_personal_org.polyfill-compromise-copy) to identify vulnerable code.
2. LLM-Powered Patching: Utilizing Patchwork (https://github.com/patched-codes/patchwork), an open-source solution that employs LLMs to automatically fix vulnerabilities.
3. Custom Workflows: The "Fixpolyfill" patchflow (https://github.com/patched-codes/patchwork-configs/tree/main/patchflows/Fixpolyfill) , tailored for this specific attack, can be easily run across multiple repositories.
4. Scalable Solutions: Options to scan and patch entire GitHub/GitLab organizations, with automated pull request generation.
5. Rapid Response: LLM-assisted patching enables swift action to minimize damage from supply chain attacks.
This approach demonstrates how LLMs can be effectively used to quickly respond to and remediate widespread security vulnerabilities in code.