File size: 22,300 Bytes
b860ca0 af20412 b860ca0 |
1 2 3 4 5 6 7 8 9 10 11 12 13 14 15 16 17 18 19 20 21 22 23 24 25 26 27 28 29 30 31 32 33 34 35 36 37 38 39 40 41 42 43 44 45 46 47 48 49 50 51 52 53 54 55 56 57 58 59 60 61 62 63 64 65 66 67 68 69 70 71 72 73 74 75 76 77 78 79 80 81 82 83 84 85 86 87 88 89 90 91 92 93 94 95 96 97 98 99 100 101 102 103 104 105 106 107 108 109 110 111 112 113 114 115 116 117 118 119 120 121 122 123 124 125 126 127 128 129 130 131 132 133 134 135 136 137 138 139 140 141 142 143 144 145 146 147 148 149 150 151 152 153 154 155 156 157 158 159 160 161 162 163 164 165 166 167 168 169 170 171 172 173 174 175 176 177 178 179 180 181 182 183 184 185 186 187 188 189 190 191 192 193 194 195 196 197 198 199 200 201 202 203 204 205 206 207 208 209 210 211 212 213 214 215 216 217 218 219 220 221 222 223 224 225 226 227 228 229 230 231 232 233 234 235 236 237 238 239 240 241 242 243 244 245 246 247 248 249 250 251 252 253 254 255 256 257 258 259 260 261 262 263 264 265 266 267 268 269 270 271 272 273 274 275 276 277 278 279 280 281 282 283 284 285 286 287 288 289 290 291 292 293 294 295 296 297 298 299 300 301 302 303 304 305 306 307 308 309 310 311 312 313 314 315 316 317 318 319 320 321 322 323 324 325 326 327 328 329 330 331 332 333 334 335 336 337 338 339 340 341 342 343 344 345 346 347 348 349 350 351 352 353 354 355 356 357 358 359 360 361 362 363 364 365 366 367 368 369 370 371 372 373 374 375 376 377 378 379 380 381 382 383 384 385 386 387 388 389 390 391 392 393 394 395 396 397 398 399 400 401 402 403 404 405 406 407 408 409 410 411 412 413 414 415 416 417 418 419 420 421 422 423 424 425 426 427 428 429 430 431 432 433 434 435 436 437 438 439 440 441 442 443 444 445 446 447 448 449 450 451 452 453 454 455 456 457 458 459 460 461 462 463 464 465 |
# CogVideoX-Fun
๐ Welcome!
[](https://huggingface.co/spaces/alibaba-pai/CogVideoX-Fun-5b)
English | [็ฎไฝไธญๆ](./README.md)
# Table of Contents
- [Table of Contents](#table-of-contents)
- [Introduction](#introduction)
- [Quick Start](#quick-start)
- [Video Result](#video-result)
- [How to use](#how-to-use)
- [Model zoo](#model-zoo)
- [TODO List](#todo-list)
- [Reference](#reference)
- [License](#license)
# Introduction
CogVideoX-Fun is a modified pipeline based on the CogVideoX structure, designed to provide more flexibility in generation. It can be used to create AI images and videos, as well as to train baseline models and Lora models for Diffusion Transformer. We support predictions directly from the already trained CogVideoX-Fun model, allowing the generation of videos at different resolutions, approximately 6 seconds long with 8 fps (1 to 49 frames). Users can also train their own baseline models and Lora models to achieve certain style transformations.
We will support quick pull-ups from different platforms, refer to [Quick Start](#quick-start).
What's New:
- Use reward backpropagation to train Lora and optimize the video, aligning it better with human preferences, detailes in [here](scripts/README_TRAIN_REWARD.md). A new version of the control model supports various conditions (e.g., Canny, Depth, Pose, MLSD, etc.). [2024.11.21]
- CogVideoX-Fun Control is now supported in diffusers. Thanks to [a-r-r-o-w](https://github.com/a-r-r-o-w) who contributed the support in this [PR](https://github.com/huggingface/diffusers/pull/9671). Check out the [docs](https://huggingface.co/docs/diffusers/main/en/api/pipelines/cogvideox) to know more. [ 2024.10.16 ]
- Retrain the i2v model and add noise to increase the motion amplitude of the video. Upload the control model training code and control model. [ 2024.09.29 ]
- Create code! Now supporting Windows and Linux. Supports 2b and 5b models. Supports video generation at any resolution from 256x256x49 to 1024x1024x49. [ 2024.09.18 ]
Function๏ผ
- [Data Preprocessing](#data-preprocess)
- [Train DiT](#dit-train)
- [Video Generation](#video-gen)
Our UI interface is as follows:
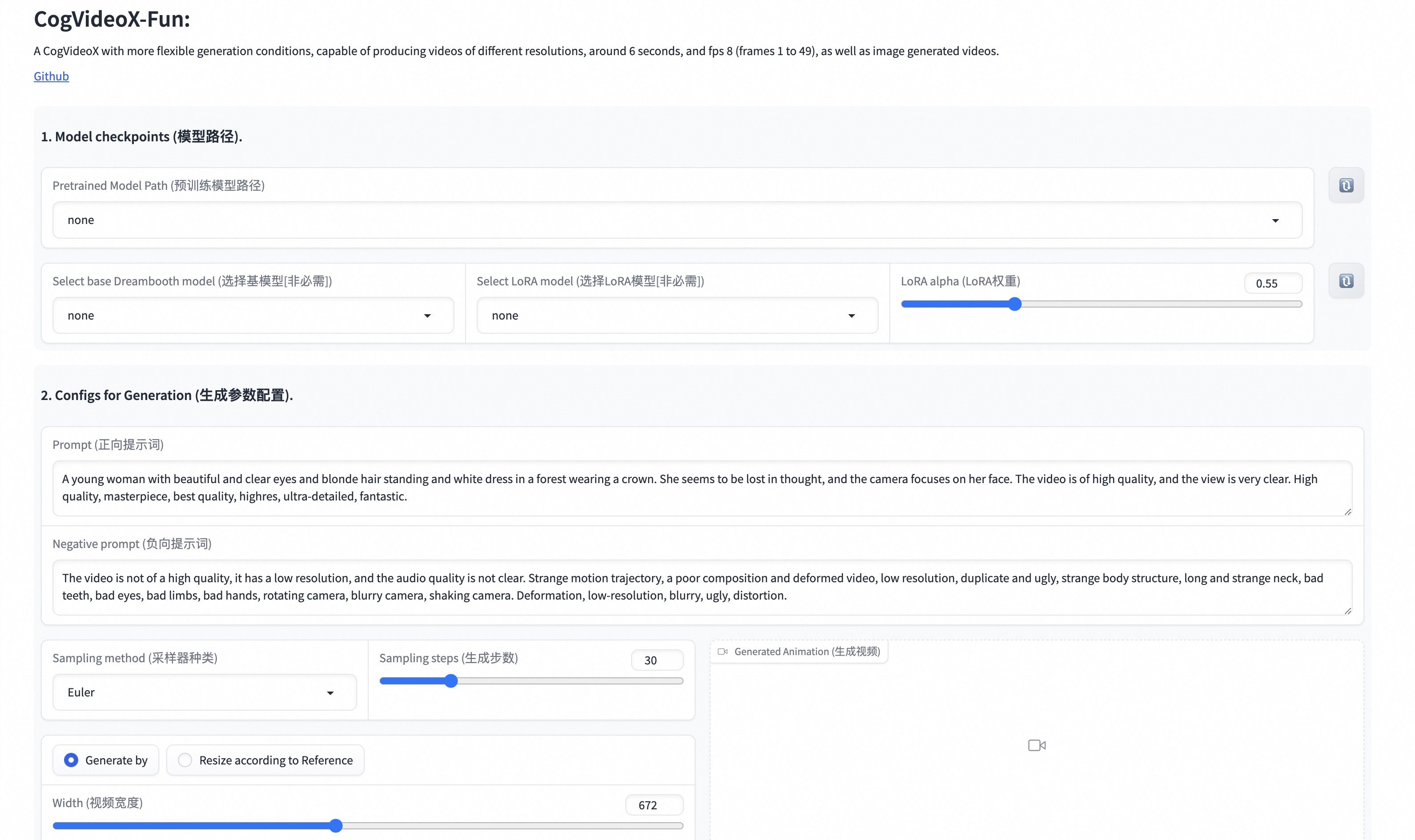
# Quick Start
### 1. Cloud usage: AliyunDSW/Docker
#### a. From AliyunDSW
DSW has free GPU time, which can be applied once by a user and is valid for 3 months after applying.
Aliyun provide free GPU time in [Freetier](https://free.aliyun.com/?product=9602825&crowd=enterprise&spm=5176.28055625.J_5831864660.1.e939154aRgha4e&scm=20140722.M_9974135.P_110.MO_1806-ID_9974135-MID_9974135-CID_30683-ST_8512-V_1), get it and use in Aliyun PAI-DSW to start CogVideoX-Fun within 5min!
[](https://gallery.pai-ml.com/#/preview/deepLearning/cv/cogvideox_fun)
#### b. From ComfyUI
Our ComfyUI is as follows, please refer to [ComfyUI README](comfyui/README.md) for details.

#### c. From docker
If you are using docker, please make sure that the graphics card driver and CUDA environment have been installed correctly in your machine.
Then execute the following commands in this way:
```
# pull image
docker pull mybigpai-public-registry.cn-beijing.cr.aliyuncs.com/easycv/torch_cuda:cogvideox_fun
# enter image
docker run -it -p 7860:7860 --network host --gpus all --security-opt seccomp:unconfined --shm-size 200g mybigpai-public-registry.cn-beijing.cr.aliyuncs.com/easycv/torch_cuda:cogvideox_fun
# clone code
git clone https://github.com/aigc-apps/CogVideoX-Fun.git
# enter CogVideoX-Fun's dir
cd CogVideoX-Fun
# download weights
mkdir models/Diffusion_Transformer
mkdir models/Personalized_Model
wget https://pai-aigc-photog.oss-cn-hangzhou.aliyuncs.com/cogvideox_fun/Diffusion_Transformer/CogVideoX-Fun-V1.1-2b-InP.tar.gz -O models/Diffusion_Transformer/CogVideoX-Fun-V1.1-2b-InP.tar.gz
cd models/Diffusion_Transformer/
tar -xvf CogVideoX-Fun-V1.1-2b-InP.tar.gz
cd ../../
```
### 2. Local install: Environment Check/Downloading/Installation
#### a. Environment Check
We have verified CogVideoX-Fun execution on the following environment:
The detailed of Windows:
- OS: Windows 10
- python: python3.10 & python3.11
- pytorch: torch2.2.0
- CUDA: 11.8 & 12.1
- CUDNN: 8+
- GPU๏ผ Nvidia-3060 12G & Nvidia-3090 24G
The detailed of Linux:
- OS: Ubuntu 20.04, CentOS
- python: python3.10 & python3.11
- pytorch: torch2.2.0
- CUDA: 11.8 & 12.1
- CUDNN: 8+
- GPU๏ผNvidia-V100 16G & Nvidia-A10 24G & Nvidia-A100 40G & Nvidia-A100 80G
We need about 60GB available on disk (for saving weights), please check!
#### b. Weights
We'd better place the [weights](#model-zoo) along the specified path:
```
๐ฆ models/
โโโ ๐ Diffusion_Transformer/
โ โโโ ๐ CogVideoX-Fun-V1.1-2b-InP/
โ โโโ ๐ CogVideoX-Fun-V1.1-5b-InP/
โโโ ๐ Personalized_Model/
โ โโโ your trained trainformer model / your trained lora model (for UI load)
```
# Video Result
The results displayed are all based on image.
### CogVideoX-Fun-V1.1-5B
Resolution-1024
<table border="0" style="width: 100%; text-align: left; margin-top: 20px;">
<tr>
<td>
<video src="https://github.com/user-attachments/assets/34e7ec8f-293e-4655-bb14-5e1ee476f788" width="100%" controls autoplay loop></video>
</td>
<td>
<video src="https://github.com/user-attachments/assets/7809c64f-eb8c-48a9-8bdc-ca9261fd5434" width="100%" controls autoplay loop></video>
</td>
<td>
<video src="https://github.com/user-attachments/assets/8e76aaa4-c602-44ac-bcb4-8b24b72c386c" width="100%" controls autoplay loop></video>
</td>
<td>
<video src="https://github.com/user-attachments/assets/19dba894-7c35-4f25-b15c-384167ab3b03" width="100%" controls autoplay loop></video>
</td>
</tr>
</table>
Resolution-768
<table border="0" style="width: 100%; text-align: left; margin-top: 20px;">
<tr>
<td>
<video src="https://github.com/user-attachments/assets/0bc339b9-455b-44fd-8917-80272d702737" width="100%" controls autoplay loop></video>
</td>
<td>
<video src="https://github.com/user-attachments/assets/70a043b9-6721-4bd9-be47-78b7ec5c27e9" width="100%" controls autoplay loop></video>
</td>
<td>
<video src="https://github.com/user-attachments/assets/d5dd6c09-14f3-40f8-8b6d-91e26519b8ac" width="100%" controls autoplay loop></video>
</td>
<td>
<video src="https://github.com/user-attachments/assets/9327e8bc-4f17-46b0-b50d-38c250a9483a" width="100%" controls autoplay loop></video>
</td>
</tr>
</table>
Resolution-512
<table border="0" style="width: 100%; text-align: left; margin-top: 20px;">
<tr>
<td>
<video src="https://github.com/user-attachments/assets/ef407030-8062-454d-aba3-131c21e6b58c" width="100%" controls autoplay loop></video>
</td>
<td>
<video src="https://github.com/user-attachments/assets/7610f49e-38b6-4214-aa48-723ae4d1b07e" width="100%" controls autoplay loop></video>
</td>
<td>
<video src="https://github.com/user-attachments/assets/1fff0567-1e15-415c-941e-53ee8ae2c841" width="100%" controls autoplay loop></video>
</td>
<td>
<video src="https://github.com/user-attachments/assets/bcec48da-b91b-43a0-9d50-cf026e00fa4f" width="100%" controls autoplay loop></video>
</td>
</tr>
</table>
### CogVideoX-Fun-V1.1-5B with Reward Backpropagation
<table border="0" style="width: 100%; text-align: center; margin-top: 20px;">
<thead>
<tr>
<th style="text-align: center;" width="10%">Prompt</sup></th>
<th style="text-align: center;" width="30%">CogVideoX-Fun-V1.1-5B</th>
<th style="text-align: center;" width="30%">CogVideoX-Fun-V1.1-5B <br> HPSv2.1 Reward LoRA</th>
<th style="text-align: center;" width="30%">CogVideoX-Fun-V1.1-5B <br> MPS Reward LoRA</th>
</tr>
</thead>
<tr>
<td>
Pig with wings flying above a diamond mountain
</td>
<td>
<video src="https://github.com/user-attachments/assets/6682f507-4ca2-45e9-9d76-86e2d709efb3" width="100%" controls autoplay loop></video>
</td>
<td>
<video src="https://github.com/user-attachments/assets/ec9219a2-96b3-44dd-b918-8176b2beb3b0" width="100%" controls autoplay loop></video>
</td>
<td>
<video src="https://github.com/user-attachments/assets/a75c6a6a-0b69-4448-afc0-fda3c7955ba0" width="100%" controls autoplay loop></video>
</td>
</tr>
<tr>
<td>
A dog runs through a field while a cat climbs a tree
</td>
<td>
<video src="https://github.com/user-attachments/assets/0392d632-2ec3-46b4-8867-0da1db577b6d" width="100%" controls autoplay loop></video>
</td>
<td>
<video src="https://github.com/user-attachments/assets/7d8c729d-6afb-408e-b812-67c40c3aaa96" width="100%" controls autoplay loop></video>
</td>
<td>
<video src="https://github.com/user-attachments/assets/dcd1343c-7435-4558-b602-9c0fa08cbd59" width="100%" controls autoplay loop></video>
</td>
</tr>
</table>
### CogVideoX-Fun-V1.1-5B-Control
<table border="0" style="width: 100%; text-align: left; margin-top: 20px;">
<tr>
<td>
<video src="https://github.com/user-attachments/assets/53002ce2-dd18-4d4f-8135-b6f68364cabd" width="100%" controls autoplay loop></video>
</td>
<td>
<video src="https://github.com/user-attachments/assets/fce43c0b-81fa-4ab2-9ca7-78d786f520e6" width="100%" controls autoplay loop></video>
</td>
<td>
<video src="https://github.com/user-attachments/assets/b208b92c-5add-4ece-a200-3dbbe47b93c3" width="100%" controls autoplay loop></video>
</td>
<tr>
<td>
A young woman with beautiful clear eyes and blonde hair, wearing white clothes and twisting her body, with the camera focused on her face. High quality, masterpiece, best quality, high resolution, ultra-fine, dreamlike.
</td>
<td>
A young woman with beautiful clear eyes and blonde hair, wearing white clothes and twisting her body, with the camera focused on her face. High quality, masterpiece, best quality, high resolution, ultra-fine, dreamlike.
</td>
<td>
A young bear.
</td>
</tr>
<tr>
<td>
<video src="https://github.com/user-attachments/assets/ea908454-684b-4d60-b562-3db229a250a9" width="100%" controls autoplay loop></video>
</td>
<td>
<video src="https://github.com/user-attachments/assets/ffb7c6fc-8b69-453b-8aad-70dfae3899b9" width="100%" controls autoplay loop></video>
</td>
<td>
<video src="https://github.com/user-attachments/assets/d3f757a3-3551-4dcb-9372-7a61469813f5" width="100%" controls autoplay loop></video>
</td>
</tr>
</table>
### CogVideoX-Fun-V1.1-5B-Pose
<table border="0" style="width: 100%; text-align: left; margin-top: 20px;">
<tr>
<td>
Resolution-512
</td>
<td>
Resolution-768
</td>
<td>
Resolution-1024
</td>
<tr>
<td>
<video src="https://github.com/user-attachments/assets/a746df51-9eb7-4446-bee5-2ee30285c143" width="100%" controls autoplay loop></video>
</td>
<td>
<video src="https://github.com/user-attachments/assets/db295245-e6aa-43be-8c81-32cb411f1473" width="100%" controls autoplay loop></video>
</td>
<td>
<video src="https://github.com/user-attachments/assets/ec9875b2-fde0-48e1-ab7e-490cee51ef40" width="100%" controls autoplay loop></video>
</td>
</tr>
</table>
### CogVideoX-Fun-V1.1-2B
Resolution-768
<table border="0" style="width: 100%; text-align: left; margin-top: 20px;">
<tr>
<td>
<video src="https://github.com/user-attachments/assets/03235dea-980e-4fc5-9c41-e40a5bc1b6d0" width="100%" controls autoplay loop></video>
</td>
<td>
<video src="https://github.com/user-attachments/assets/f7302648-5017-47db-bdeb-4d893e620b37" width="100%" controls autoplay loop></video>
</td>
<td>
<video src="https://github.com/user-attachments/assets/cbadf411-28fa-4b87-813d-da63ff481904" width="100%" controls autoplay loop></video>
</td>
<td>
<video src="https://github.com/user-attachments/assets/87cc9d0b-b6fe-4d2d-b447-174513d169ab" width="100%" controls autoplay loop></video>
</td>
</tr>
</table>
### CogVideoX-Fun-V1.1-2B-Pose
<table border="0" style="width: 100%; text-align: left; margin-top: 20px;">
<tr>
<td>
Resolution-512
</td>
<td>
Resolution-768
</td>
<td>
Resolution-1024
</td>
<tr>
<td>
<video src="https://github.com/user-attachments/assets/487bcd7b-1b7f-4bb4-95b5-96a6b6548b3e" width="100%" controls autoplay loop></video>
</td>
<td>
<video src="https://github.com/user-attachments/assets/2710fd18-8489-46e4-8086-c237309ae7f6" width="100%" controls autoplay loop></video>
</td>
<td>
<video src="https://github.com/user-attachments/assets/b79513db-7747-4512-b86c-94f9ca447fe2" width="100%" controls autoplay loop></video>
</td>
</tr>
</table>
# How to use
<h3 id="video-gen">1. Inference </h3>
#### a. Using Python Code
- Step 1: Download the corresponding [weights](#model-zoo) and place them in the models folder.
- Step 2: Modify prompt, neg_prompt, guidance_scale, and seed in the predict_t2v.py file.
- Step 3: Run the predict_t2v.py file, wait for the generated results, and save the results in the samples/cogvideox-fun-videos-t2v folder.
- Step 4: If you want to combine other backbones you have trained with Lora, modify the predict_t2v.py and Lora_path in predict_t2v.py depending on the situation.
#### b. Using webui
- Step 1: Download the corresponding [weights](#model-zoo) and place them in the models folder.
- Step 2: Run the app.py file to enter the graph page.
- Step 3: Select the generated model based on the page, fill in prompt, neg_prompt, guidance_scale, and seed, click on generate, wait for the generated result, and save the result in the samples folder.
#### c. From ComfyUI
Please refer to [ComfyUI README](comfyui/README.md) for details.
### 2. Model Training
A complete CogVideoX-Fun training pipeline should include data preprocessing, and Video DiT training.
<h4 id="data-preprocess">a. data preprocessing</h4>
We have provided a simple demo of training the Lora model through image data, which can be found in the [wiki](https://github.com/aigc-apps/CogVideoX-Fun/wiki/Training-Lora) for details.
A complete data preprocessing link for long video segmentation, cleaning, and description can refer to [README](cogvideox/video_caption/README.md) in the video captions section.
If you want to train a text to image and video generation model. You need to arrange the dataset in this format.
```
๐ฆ project/
โโโ ๐ datasets/
โ โโโ ๐ internal_datasets/
โ โโโ ๐ train/
โ โ โโโ ๐ 00000001.mp4
โ โ โโโ ๐ 00000002.jpg
โ โ โโโ ๐ .....
โ โโโ ๐ json_of_internal_datasets.json
```
The json_of_internal_datasets.json is a standard JSON file. The file_path in the json can to be set as relative path, as shown in below:
```json
[
{
"file_path": "train/00000001.mp4",
"text": "A group of young men in suits and sunglasses are walking down a city street.",
"type": "video"
},
{
"file_path": "train/00000002.jpg",
"text": "A group of young men in suits and sunglasses are walking down a city street.",
"type": "image"
},
.....
]
```
You can also set the path as absolute path as follow:
```json
[
{
"file_path": "/mnt/data/videos/00000001.mp4",
"text": "A group of young men in suits and sunglasses are walking down a city street.",
"type": "video"
},
{
"file_path": "/mnt/data/train/00000001.jpg",
"text": "A group of young men in suits and sunglasses are walking down a city street.",
"type": "image"
},
.....
]
```
<h4 id="dit-train">b. Video DiT training </h4>
If the data format is relative path during data preprocessing, please set ```scripts/train.sh``` as follow.
```
export DATASET_NAME="datasets/internal_datasets/"
export DATASET_META_NAME="datasets/internal_datasets/json_of_internal_datasets.json"
```
If the data format is absolute path during data preprocessing, please set ```scripts/train.sh``` as follow.
```
export DATASET_NAME=""
export DATASET_META_NAME="/mnt/data/json_of_internal_datasets.json"
```
Then, we run scripts/train.sh.
```sh
sh scripts/train.sh
```
For details on setting some parameters, please refer to [Readme Train](scripts/README_TRAIN.md), [Readme Lora](scripts/README_TRAIN_LORA.md) and [Readme Control](scripts/README_TRAIN_CONTROL.md).
# Model zoo
V1.5:
| Name | Storage Space | Hugging Face | Model Scope | Description |
|--|--|--|--|--|
| CogVideoX-Fun-V1.5-5b-InP | 20.0 GB | [๐คLink](https://huggingface.co/alibaba-pai/CogVideoX-Fun-V1.5-5b-InP) | [๐Link](https://modelscope.cn/models/PAI/CogVideoX-Fun-V1.5-5b-InP) | Our official graph-generated video model is capable of predicting videos at multiple resolutions (512, 768, 1024) and has been trained on 85 frames at a rate of 8 frames per second. |
V1.1:
| Name | Storage Space | Hugging Face | Model Scope | Description |
|--|--|--|--|--|
| CogVideoX-Fun-V1.1-2b-InP | 13.0 GB | [๐คLink](https://huggingface.co/alibaba-pai/CogVideoX-Fun-V1.1-2b-InP) | [๐Link](https://modelscope.cn/models/PAI/CogVideoX-Fun-V1.1-2b-InP) | Our official graph-generated video model is capable of predicting videos at multiple resolutions (512, 768, 1024, 1280) and has been trained on 49 frames at a rate of 8 frames per second. |
| CogVideoX-Fun-V1.1-5b-InP | 20.0 GB | [๐คLink](https://huggingface.co/alibaba-pai/CogVideoX-Fun-V1.1-5b-InP) | [๐Link](https://modelscope.cn/models/PAI/CogVideoX-Fun-V1.1-5b-InP) | Our official graph-generated video model is capable of predicting videos at multiple resolutions (512, 768, 1024, 1280) and has been trained on 49 frames at a rate of 8 frames per second. Noise has been added to the reference image, and the amplitude of motion is greater compared to V1.0. |
| CogVideoX-Fun-V1.1-2b-Pose | 13.0 GB | [๐คLink](https://huggingface.co/alibaba-pai/CogVideoX-Fun-V1.1-2b-Pose) | [๐Link](https://modelscope.cn/models/PAI/CogVideoX-Fun-V1.1-2b-Pose) | Our official pose-control video model is capable of predicting videos at multiple resolutions (512, 768, 1024, 1280) and has been trained on 49 frames at a rate of 8 frames per second.|
| CogVideoX-Fun-V1.1-2b-Control | 13.0 GB | [๐คLink](https://huggingface.co/alibaba-pai/CogVideoX-Fun-V1.1-2b-Control) | [๐Link](https://modelscope.cn/models/PAI/CogVideoX-Fun-V1.1-2b-Control) | Our official control video model is capable of predicting videos at multiple resolutions (512, 768, 1024, 1280) and has been trained on 49 frames at a rate of 8 frames per second. Supporting various control conditions such as Canny, Depth, Pose, MLSD, etc.|
| CogVideoX-Fun-V1.1-5b-Pose | 20.0 GB | [๐คLink](https://huggingface.co/alibaba-pai/CogVideoX-Fun-V1.1-5b-Pose) | [๐Link](https://modelscope.cn/models/PAI/CogVideoX-Fun-V1.1-5b-Pose) | Our official pose-control video model is capable of predicting videos at multiple resolutions (512, 768, 1024, 1280) and has been trained on 49 frames at a rate of 8 frames per second.|
| CogVideoX-Fun-V1.1-5b-Control | 20.0 GB | [๐คLink](https://huggingface.co/alibaba-pai/CogVideoX-Fun-V1.1-5b-Control) | [๐Link](https://modelscope.cn/models/PAI/CogVideoX-Fun-V1.1-5b-Control) | Our official control video model is capable of predicting videos at multiple resolutions (512, 768, 1024, 1280) and has been trained on 49 frames at a rate of 8 frames per second. Supporting various control conditions such as Canny, Depth, Pose, MLSD, etc.|
| CogVideoX-Fun-V1.1-Reward-LoRAs | - | [๐คLink](https://huggingface.co/alibaba-pai/CogVideoX-Fun-V1.1-Reward-LoRAs) | [๐Link](https://modelscope.cn/models/PAI/CogVideoX-Fun-V1.1-Reward-LoRAs) | The official reward backpropagation technology model optimizes the videos generated by CogVideoX-Fun-V1.1 to better match human preferences. ๏ฝ
V1.0:
| Name | Storage Space | Hugging Face | Model Scope | Description |
|--|--|--|--|--|
| CogVideoX-Fun-2b-InP | 13.0 GB | [๐คLink](https://huggingface.co/alibaba-pai/CogVideoX-Fun-2b-InP) | [๐Link](https://modelscope.cn/models/PAI/CogVideoX-Fun-2b-InP) | Our official graph-generated video model is capable of predicting videos at multiple resolutions (512, 768, 1024, 1280) and has been trained on 49 frames at a rate of 8 frames per second. |
| CogVideoX-Fun-5b-InP | 20.0 GB | [๐คLink](https://huggingface.co/alibaba-pai/CogVideoX-Fun-5b-InP)| [๐Link](https://modelscope.cn/models/PAI/CogVideoX-Fun-5b-InP)| Our official graph-generated video model is capable of predicting videos at multiple resolutions (512, 768, 1024, 1280) and has been trained on 49 frames at a rate of 8 frames per second. |
# TODO List
- Support Chinese.
# Reference
- CogVideo: https://github.com/THUDM/CogVideo/
- EasyAnimate: https://github.com/aigc-apps/EasyAnimate
# License
This project is licensed under the [Apache License (Version 2.0)](https://github.com/modelscope/modelscope/blob/master/LICENSE).
The CogVideoX-2B model (including its corresponding Transformers module and VAE module) is released under the [Apache 2.0 License](LICENSE).
The CogVideoX-5B model (Transformers module) is released under the [CogVideoX LICENSE](https://huggingface.co/THUDM/CogVideoX-5b/blob/main/LICENSE).
|