uploaded readme
Browse files
README.md
ADDED
@@ -0,0 +1,898 @@
|
|
|
|
|
|
|
|
|
|
|
|
|
|
|
|
|
|
|
|
|
|
|
|
|
|
|
|
|
|
|
|
|
|
|
|
|
|
|
|
|
|
|
|
|
|
|
|
|
|
|
|
|
|
|
|
|
|
|
|
|
|
|
|
|
|
|
|
|
|
|
|
|
|
|
|
|
|
|
|
|
|
|
|
|
|
|
|
|
|
|
|
|
|
|
|
|
|
|
|
|
|
|
|
|
|
|
|
|
|
|
|
|
|
|
|
|
|
|
|
|
|
|
|
|
|
|
|
|
|
|
|
|
|
|
|
|
|
|
|
|
|
|
|
|
|
|
|
|
|
|
|
|
|
|
|
|
|
|
|
|
|
|
|
|
|
|
|
|
|
|
|
|
|
|
|
|
|
|
|
|
|
|
|
|
|
|
|
|
|
|
|
|
|
|
|
|
|
|
|
|
|
|
|
|
|
|
|
|
|
|
|
|
|
|
|
|
|
|
|
|
|
|
|
|
|
|
|
|
|
|
|
|
|
|
|
|
|
|
|
|
|
|
|
|
|
|
|
|
|
|
|
|
|
|
|
|
|
|
|
|
|
|
|
|
|
|
|
|
|
|
|
|
|
|
|
|
|
|
|
|
|
|
|
|
|
|
|
|
|
|
|
|
|
|
|
|
|
|
|
|
|
|
|
|
|
|
|
|
|
|
|
|
|
|
|
|
|
|
|
|
|
|
|
|
|
|
|
|
|
|
|
|
|
|
|
|
|
|
|
|
|
|
|
|
|
|
|
|
|
|
|
|
|
|
|
|
|
|
|
|
|
|
|
|
|
|
|
|
|
|
|
|
|
|
|
|
|
|
|
|
|
|
|
|
|
|
|
|
|
|
|
|
|
|
|
|
|
|
|
|
|
|
|
|
|
|
|
|
|
|
|
|
|
|
|
|
|
|
|
|
|
|
|
|
|
|
|
|
|
|
|
|
|
|
|
|
|
|
|
|
|
|
|
|
|
|
|
|
|
|
|
|
|
|
|
|
|
|
|
|
|
|
|
|
|
|
|
|
|
|
|
|
|
|
|
|
|
|
|
|
|
|
|
|
|
|
|
|
|
|
|
|
|
|
|
|
|
|
|
|
|
|
|
|
|
|
|
|
|
|
|
|
|
|
|
|
|
|
|
|
|
|
|
|
|
|
|
|
|
|
|
|
|
|
|
|
|
|
|
|
|
|
|
|
|
|
|
|
|
|
|
|
|
|
|
|
|
|
|
|
|
|
|
|
|
|
|
|
|
|
|
|
|
|
|
|
|
|
|
|
|
|
|
|
|
|
|
|
|
|
|
|
|
|
|
|
|
|
|
|
|
|
|
|
|
|
|
|
|
|
|
|
|
|
|
|
|
|
|
|
|
|
|
|
|
|
|
|
|
|
|
|
|
|
|
|
|
|
|
|
|
|
|
|
|
|
|
|
|
|
|
|
|
|
|
|
|
|
|
|
|
|
|
|
|
|
|
|
|
|
|
|
|
|
|
|
|
|
|
|
|
|
|
|
|
|
|
|
|
|
|
|
|
|
|
|
|
|
|
|
|
|
|
|
|
|
|
|
|
|
|
|
|
|
|
|
|
|
|
|
|
|
|
|
|
|
|
|
|
|
|
|
|
|
|
|
|
|
|
|
|
|
|
|
|
|
|
|
|
|
|
|
|
|
|
|
|
|
|
|
|
|
|
|
|
|
|
|
|
|
|
|
|
|
|
|
|
|
|
|
|
|
|
|
|
|
|
|
|
|
|
|
|
|
|
|
|
|
|
|
|
|
|
|
|
|
|
|
|
|
|
|
|
|
|
|
|
|
|
|
|
|
|
|
|
|
|
|
|
|
|
|
|
|
|
|
|
|
|
|
|
|
|
|
|
|
|
|
|
|
|
|
|
|
|
|
|
|
|
|
|
|
|
|
|
|
|
|
|
|
|
|
|
|
|
|
|
|
|
|
|
|
|
|
|
|
|
|
|
|
|
|
|
|
|
|
|
|
|
|
|
|
|
|
|
|
|
|
|
|
|
|
|
|
|
|
|
|
|
|
|
|
|
|
|
|
|
|
|
|
|
|
|
|
|
|
|
|
|
|
|
|
|
|
|
|
|
|
|
|
|
|
|
|
|
|
|
|
|
|
|
|
|
|
|
|
|
|
|
|
|
|
|
|
|
|
|
|
|
|
|
|
|
|
|
|
|
|
|
|
|
|
|
|
|
|
|
|
|
|
|
|
|
|
|
|
|
|
|
|
|
|
|
|
|
|
|
|
|
|
|
|
|
|
|
|
|
|
|
|
|
|
|
|
|
|
|
|
|
|
|
|
|
|
|
|
|
|
|
|
|
|
|
|
|
|
|
|
|
|
|
|
|
|
|
|
|
|
|
|
|
|
|
|
|
|
|
|
|
|
|
|
|
|
|
|
|
|
|
|
|
|
|
|
|
|
|
|
|
|
|
|
|
|
|
|
|
|
|
|
|
|
|
|
|
|
|
|
|
|
|
|
|
|
|
|
|
|
|
|
|
|
|
|
|
|
|
|
|
|
|
|
|
|
|
|
|
|
|
|
|
|
|
|
|
|
|
|
|
|
|
|
|
|
|
|
|
|
|
|
|
|
|
|
|
|
|
|
|
|
|
|
|
|
|
|
|
|
|
|
|
|
|
|
|
|
|
|
|
|
|
|
|
|
|
|
|
|
|
|
|
|
|
|
|
|
|
|
|
|
|
|
|
|
|
|
|
|
|
|
|
|
|
|
|
|
|
|
|
|
|
|
|
|
|
|
|
|
|
|
|
|
|
|
|
|
|
|
|
|
|
|
|
|
|
|
|
|
|
|
|
|
|
|
|
|
|
|
|
|
|
|
|
|
|
|
|
|
|
|
|
|
|
|
|
|
|
|
|
|
|
|
|
|
|
|
|
|
|
|
|
|
|
|
|
|
|
|
|
|
|
|
|
|
|
|
|
|
|
|
|
|
|
|
|
|
|
|
|
|
|
|
|
|
|
|
|
|
|
|
|
|
|
|
|
|
|
|
|
|
|
|
|
|
|
|
|
|
|
|
|
|
|
|
|
|
|
|
|
|
|
|
|
|
|
|
|
|
|
|
|
|
|
|
|
|
|
|
|
|
|
|
|
|
|
|
|
|
|
|
|
|
|
|
|
|
|
|
|
|
|
|
|
|
|
|
|
|
|
|
|
|
|
|
|
|
|
|
|
|
|
|
|
|
|
|
|
|
|
|
|
|
|
|
|
|
|
|
|
|
|
|
|
|
|
|
|
|
|
|
|
|
|
|
|
|
|
|
|
|
|
|
|
|
|
|
|
|
|
|
|
|
|
|
|
|
|
|
|
|
|
|
|
|
|
|
|
|
|
|
|
|
|
|
|
|
|
|
|
|
|
|
|
|
|
|
|
|
|
|
|
|
|
|
|
|
|
|
|
|
|
|
|
|
|
|
|
|
|
|
|
|
|
|
|
|
|
|
|
|
|
|
|
|
|
|
|
|
|
|
|
|
|
|
|
|
|
|
|
|
|
|
|
|
|
|
|
|
|
|
|
|
|
|
|
|
|
|
|
|
|
|
|
|
|
|
|
|
|
|
|
|
|
|
|
|
|
|
|
|
|
|
|
|
|
|
|
|
|
|
|
|
|
|
|
|
|
|
|
|
|
|
|
|
|
|
|
|
|
|
|
|
|
|
|
|
|
|
|
|
|
|
|
|
|
|
|
|
|
|
|
|
|
|
|
|
|
|
|
|
|
|
|
|
|
|
|
|
|
|
|
|
|
|
|
|
|
|
|
|
|
|
|
|
|
|
|
|
|
|
|
|
|
|
|
|
|
|
|
|
|
|
|
|
|
|
|
|
|
|
|
|
|
|
|
|
|
|
|
|
|
|
|
|
|
|
|
|
|
|
|
|
|
|
|
|
|
|
|
|
|
|
|
|
|
|
|
|
|
|
|
|
|
|
|
|
|
|
|
|
|
|
|
|
|
|
|
|
|
|
|
|
|
|
|
|
|
|
1 |
+
Quantization made by Richard Erkhov.
|
2 |
+
|
3 |
+
[Github](https://github.com/RichardErkhov)
|
4 |
+
|
5 |
+
[Discord](https://discord.gg/pvy7H8DZMG)
|
6 |
+
|
7 |
+
[Request more models](https://github.com/RichardErkhov/quant_request)
|
8 |
+
|
9 |
+
|
10 |
+
bloomz-3b - bnb 4bits
|
11 |
+
- Model creator: https://huggingface.co/bigscience/
|
12 |
+
- Original model: https://huggingface.co/bigscience/bloomz-3b/
|
13 |
+
|
14 |
+
|
15 |
+
|
16 |
+
|
17 |
+
Original model description:
|
18 |
+
---
|
19 |
+
datasets:
|
20 |
+
- bigscience/xP3
|
21 |
+
license: bigscience-bloom-rail-1.0
|
22 |
+
language:
|
23 |
+
- ak
|
24 |
+
- ar
|
25 |
+
- as
|
26 |
+
- bm
|
27 |
+
- bn
|
28 |
+
- ca
|
29 |
+
- code
|
30 |
+
- en
|
31 |
+
- es
|
32 |
+
- eu
|
33 |
+
- fon
|
34 |
+
- fr
|
35 |
+
- gu
|
36 |
+
- hi
|
37 |
+
- id
|
38 |
+
- ig
|
39 |
+
- ki
|
40 |
+
- kn
|
41 |
+
- lg
|
42 |
+
- ln
|
43 |
+
- ml
|
44 |
+
- mr
|
45 |
+
- ne
|
46 |
+
- nso
|
47 |
+
- ny
|
48 |
+
- or
|
49 |
+
- pa
|
50 |
+
- pt
|
51 |
+
- rn
|
52 |
+
- rw
|
53 |
+
- sn
|
54 |
+
- st
|
55 |
+
- sw
|
56 |
+
- ta
|
57 |
+
- te
|
58 |
+
- tn
|
59 |
+
- ts
|
60 |
+
- tum
|
61 |
+
- tw
|
62 |
+
- ur
|
63 |
+
- vi
|
64 |
+
- wo
|
65 |
+
- xh
|
66 |
+
- yo
|
67 |
+
- zh
|
68 |
+
- zu
|
69 |
+
programming_language:
|
70 |
+
- C
|
71 |
+
- C++
|
72 |
+
- C#
|
73 |
+
- Go
|
74 |
+
- Java
|
75 |
+
- JavaScript
|
76 |
+
- Lua
|
77 |
+
- PHP
|
78 |
+
- Python
|
79 |
+
- Ruby
|
80 |
+
- Rust
|
81 |
+
- Scala
|
82 |
+
- TypeScript
|
83 |
+
pipeline_tag: text-generation
|
84 |
+
widget:
|
85 |
+
- text: "一个传奇的开端,一个不灭的神话,这不仅仅是一部电影,而是作为一个走进新时代的标签,永远彪炳史册。Would you rate the previous review as positive, neutral or negative?"
|
86 |
+
example_title: "zh-en sentiment"
|
87 |
+
- text: "一个传奇的开端,一个不灭的神话,这不仅仅是一部电影,而是作为一个走进新时代的标签,永远彪炳史册。你认为这句话的立场是赞扬、中立还是批评?"
|
88 |
+
example_title: "zh-zh sentiment"
|
89 |
+
- text: "Suggest at least five related search terms to \"Mạng neural nhân tạo\"."
|
90 |
+
example_title: "vi-en query"
|
91 |
+
- text: "Proposez au moins cinq mots clés concernant «Réseau de neurones artificiels»."
|
92 |
+
example_title: "fr-fr query"
|
93 |
+
- text: "Explain in a sentence in Telugu what is backpropagation in neural networks."
|
94 |
+
example_title: "te-en qa"
|
95 |
+
- text: "Why is the sky blue?"
|
96 |
+
example_title: "en-en qa"
|
97 |
+
- text: "Write a fairy tale about a troll saving a princess from a dangerous dragon. The fairy tale is a masterpiece that has achieved praise worldwide and its moral is \"Heroes Come in All Shapes and Sizes\". Story (in Spanish):"
|
98 |
+
example_title: "es-en fable"
|
99 |
+
- text: "Write a fable about wood elves living in a forest that is suddenly invaded by ogres. The fable is a masterpiece that has achieved praise worldwide and its moral is \"Violence is the last refuge of the incompetent\". Fable (in Hindi):"
|
100 |
+
example_title: "hi-en fable"
|
101 |
+
model-index:
|
102 |
+
- name: bloomz-3b1
|
103 |
+
results:
|
104 |
+
- task:
|
105 |
+
type: Coreference resolution
|
106 |
+
dataset:
|
107 |
+
type: winogrande
|
108 |
+
name: Winogrande XL (xl)
|
109 |
+
config: xl
|
110 |
+
split: validation
|
111 |
+
revision: a80f460359d1e9a67c006011c94de42a8759430c
|
112 |
+
metrics:
|
113 |
+
- type: Accuracy
|
114 |
+
value: 53.67
|
115 |
+
- task:
|
116 |
+
type: Coreference resolution
|
117 |
+
dataset:
|
118 |
+
type: Muennighoff/xwinograd
|
119 |
+
name: XWinograd (en)
|
120 |
+
config: en
|
121 |
+
split: test
|
122 |
+
revision: 9dd5ea5505fad86b7bedad667955577815300cee
|
123 |
+
metrics:
|
124 |
+
- type: Accuracy
|
125 |
+
value: 59.23
|
126 |
+
- task:
|
127 |
+
type: Coreference resolution
|
128 |
+
dataset:
|
129 |
+
type: Muennighoff/xwinograd
|
130 |
+
name: XWinograd (fr)
|
131 |
+
config: fr
|
132 |
+
split: test
|
133 |
+
revision: 9dd5ea5505fad86b7bedad667955577815300cee
|
134 |
+
metrics:
|
135 |
+
- type: Accuracy
|
136 |
+
value: 53.01
|
137 |
+
- task:
|
138 |
+
type: Coreference resolution
|
139 |
+
dataset:
|
140 |
+
type: Muennighoff/xwinograd
|
141 |
+
name: XWinograd (jp)
|
142 |
+
config: jp
|
143 |
+
split: test
|
144 |
+
revision: 9dd5ea5505fad86b7bedad667955577815300cee
|
145 |
+
metrics:
|
146 |
+
- type: Accuracy
|
147 |
+
value: 52.45
|
148 |
+
- task:
|
149 |
+
type: Coreference resolution
|
150 |
+
dataset:
|
151 |
+
type: Muennighoff/xwinograd
|
152 |
+
name: XWinograd (pt)
|
153 |
+
config: pt
|
154 |
+
split: test
|
155 |
+
revision: 9dd5ea5505fad86b7bedad667955577815300cee
|
156 |
+
metrics:
|
157 |
+
- type: Accuracy
|
158 |
+
value: 53.61
|
159 |
+
- task:
|
160 |
+
type: Coreference resolution
|
161 |
+
dataset:
|
162 |
+
type: Muennighoff/xwinograd
|
163 |
+
name: XWinograd (ru)
|
164 |
+
config: ru
|
165 |
+
split: test
|
166 |
+
revision: 9dd5ea5505fad86b7bedad667955577815300cee
|
167 |
+
metrics:
|
168 |
+
- type: Accuracy
|
169 |
+
value: 53.97
|
170 |
+
- task:
|
171 |
+
type: Coreference resolution
|
172 |
+
dataset:
|
173 |
+
type: Muennighoff/xwinograd
|
174 |
+
name: XWinograd (zh)
|
175 |
+
config: zh
|
176 |
+
split: test
|
177 |
+
revision: 9dd5ea5505fad86b7bedad667955577815300cee
|
178 |
+
metrics:
|
179 |
+
- type: Accuracy
|
180 |
+
value: 60.91
|
181 |
+
- task:
|
182 |
+
type: Natural language inference
|
183 |
+
dataset:
|
184 |
+
type: anli
|
185 |
+
name: ANLI (r1)
|
186 |
+
config: r1
|
187 |
+
split: validation
|
188 |
+
revision: 9dbd830a06fea8b1c49d6e5ef2004a08d9f45094
|
189 |
+
metrics:
|
190 |
+
- type: Accuracy
|
191 |
+
value: 40.1
|
192 |
+
- task:
|
193 |
+
type: Natural language inference
|
194 |
+
dataset:
|
195 |
+
type: anli
|
196 |
+
name: ANLI (r2)
|
197 |
+
config: r2
|
198 |
+
split: validation
|
199 |
+
revision: 9dbd830a06fea8b1c49d6e5ef2004a08d9f45094
|
200 |
+
metrics:
|
201 |
+
- type: Accuracy
|
202 |
+
value: 36.8
|
203 |
+
- task:
|
204 |
+
type: Natural language inference
|
205 |
+
dataset:
|
206 |
+
type: anli
|
207 |
+
name: ANLI (r3)
|
208 |
+
config: r3
|
209 |
+
split: validation
|
210 |
+
revision: 9dbd830a06fea8b1c49d6e5ef2004a08d9f45094
|
211 |
+
metrics:
|
212 |
+
- type: Accuracy
|
213 |
+
value: 40.0
|
214 |
+
- task:
|
215 |
+
type: Natural language inference
|
216 |
+
dataset:
|
217 |
+
type: super_glue
|
218 |
+
name: SuperGLUE (cb)
|
219 |
+
config: cb
|
220 |
+
split: validation
|
221 |
+
revision: 9e12063561e7e6c79099feb6d5a493142584e9e2
|
222 |
+
metrics:
|
223 |
+
- type: Accuracy
|
224 |
+
value: 75.0
|
225 |
+
- task:
|
226 |
+
type: Natural language inference
|
227 |
+
dataset:
|
228 |
+
type: super_glue
|
229 |
+
name: SuperGLUE (rte)
|
230 |
+
config: rte
|
231 |
+
split: validation
|
232 |
+
revision: 9e12063561e7e6c79099feb6d5a493142584e9e2
|
233 |
+
metrics:
|
234 |
+
- type: Accuracy
|
235 |
+
value: 76.17
|
236 |
+
- task:
|
237 |
+
type: Natural language inference
|
238 |
+
dataset:
|
239 |
+
type: xnli
|
240 |
+
name: XNLI (ar)
|
241 |
+
config: ar
|
242 |
+
split: validation
|
243 |
+
revision: a5a45e4ff92d5d3f34de70aaf4b72c3bdf9f7f16
|
244 |
+
metrics:
|
245 |
+
- type: Accuracy
|
246 |
+
value: 53.29
|
247 |
+
- task:
|
248 |
+
type: Natural language inference
|
249 |
+
dataset:
|
250 |
+
type: xnli
|
251 |
+
name: XNLI (bg)
|
252 |
+
config: bg
|
253 |
+
split: validation
|
254 |
+
revision: a5a45e4ff92d5d3f34de70aaf4b72c3bdf9f7f16
|
255 |
+
metrics:
|
256 |
+
- type: Accuracy
|
257 |
+
value: 43.82
|
258 |
+
- task:
|
259 |
+
type: Natural language inference
|
260 |
+
dataset:
|
261 |
+
type: xnli
|
262 |
+
name: XNLI (de)
|
263 |
+
config: de
|
264 |
+
split: validation
|
265 |
+
revision: a5a45e4ff92d5d3f34de70aaf4b72c3bdf9f7f16
|
266 |
+
metrics:
|
267 |
+
- type: Accuracy
|
268 |
+
value: 45.26
|
269 |
+
- task:
|
270 |
+
type: Natural language inference
|
271 |
+
dataset:
|
272 |
+
type: xnli
|
273 |
+
name: XNLI (el)
|
274 |
+
config: el
|
275 |
+
split: validation
|
276 |
+
revision: a5a45e4ff92d5d3f34de70aaf4b72c3bdf9f7f16
|
277 |
+
metrics:
|
278 |
+
- type: Accuracy
|
279 |
+
value: 42.61
|
280 |
+
- task:
|
281 |
+
type: Natural language inference
|
282 |
+
dataset:
|
283 |
+
type: xnli
|
284 |
+
name: XNLI (en)
|
285 |
+
config: en
|
286 |
+
split: validation
|
287 |
+
revision: a5a45e4ff92d5d3f34de70aaf4b72c3bdf9f7f16
|
288 |
+
metrics:
|
289 |
+
- type: Accuracy
|
290 |
+
value: 57.31
|
291 |
+
- task:
|
292 |
+
type: Natural language inference
|
293 |
+
dataset:
|
294 |
+
type: xnli
|
295 |
+
name: XNLI (es)
|
296 |
+
config: es
|
297 |
+
split: validation
|
298 |
+
revision: a5a45e4ff92d5d3f34de70aaf4b72c3bdf9f7f16
|
299 |
+
metrics:
|
300 |
+
- type: Accuracy
|
301 |
+
value: 56.14
|
302 |
+
- task:
|
303 |
+
type: Natural language inference
|
304 |
+
dataset:
|
305 |
+
type: xnli
|
306 |
+
name: XNLI (fr)
|
307 |
+
config: fr
|
308 |
+
split: validation
|
309 |
+
revision: a5a45e4ff92d5d3f34de70aaf4b72c3bdf9f7f16
|
310 |
+
metrics:
|
311 |
+
- type: Accuracy
|
312 |
+
value: 55.78
|
313 |
+
- task:
|
314 |
+
type: Natural language inference
|
315 |
+
dataset:
|
316 |
+
type: xnli
|
317 |
+
name: XNLI (hi)
|
318 |
+
config: hi
|
319 |
+
split: validation
|
320 |
+
revision: a5a45e4ff92d5d3f34de70aaf4b72c3bdf9f7f16
|
321 |
+
metrics:
|
322 |
+
- type: Accuracy
|
323 |
+
value: 51.49
|
324 |
+
- task:
|
325 |
+
type: Natural language inference
|
326 |
+
dataset:
|
327 |
+
type: xnli
|
328 |
+
name: XNLI (ru)
|
329 |
+
config: ru
|
330 |
+
split: validation
|
331 |
+
revision: a5a45e4ff92d5d3f34de70aaf4b72c3bdf9f7f16
|
332 |
+
metrics:
|
333 |
+
- type: Accuracy
|
334 |
+
value: 47.11
|
335 |
+
- task:
|
336 |
+
type: Natural language inference
|
337 |
+
dataset:
|
338 |
+
type: xnli
|
339 |
+
name: XNLI (sw)
|
340 |
+
config: sw
|
341 |
+
split: validation
|
342 |
+
revision: a5a45e4ff92d5d3f34de70aaf4b72c3bdf9f7f16
|
343 |
+
metrics:
|
344 |
+
- type: Accuracy
|
345 |
+
value: 47.83
|
346 |
+
- task:
|
347 |
+
type: Natural language inference
|
348 |
+
dataset:
|
349 |
+
type: xnli
|
350 |
+
name: XNLI (th)
|
351 |
+
config: th
|
352 |
+
split: validation
|
353 |
+
revision: a5a45e4ff92d5d3f34de70aaf4b72c3bdf9f7f16
|
354 |
+
metrics:
|
355 |
+
- type: Accuracy
|
356 |
+
value: 42.93
|
357 |
+
- task:
|
358 |
+
type: Natural language inference
|
359 |
+
dataset:
|
360 |
+
type: xnli
|
361 |
+
name: XNLI (tr)
|
362 |
+
config: tr
|
363 |
+
split: validation
|
364 |
+
revision: a5a45e4ff92d5d3f34de70aaf4b72c3bdf9f7f16
|
365 |
+
metrics:
|
366 |
+
- type: Accuracy
|
367 |
+
value: 37.23
|
368 |
+
- task:
|
369 |
+
type: Natural language inference
|
370 |
+
dataset:
|
371 |
+
type: xnli
|
372 |
+
name: XNLI (ur)
|
373 |
+
config: ur
|
374 |
+
split: validation
|
375 |
+
revision: a5a45e4ff92d5d3f34de70aaf4b72c3bdf9f7f16
|
376 |
+
metrics:
|
377 |
+
- type: Accuracy
|
378 |
+
value: 49.04
|
379 |
+
- task:
|
380 |
+
type: Natural language inference
|
381 |
+
dataset:
|
382 |
+
type: xnli
|
383 |
+
name: XNLI (vi)
|
384 |
+
config: vi
|
385 |
+
split: validation
|
386 |
+
revision: a5a45e4ff92d5d3f34de70aaf4b72c3bdf9f7f16
|
387 |
+
metrics:
|
388 |
+
- type: Accuracy
|
389 |
+
value: 53.98
|
390 |
+
- task:
|
391 |
+
type: Natural language inference
|
392 |
+
dataset:
|
393 |
+
type: xnli
|
394 |
+
name: XNLI (zh)
|
395 |
+
config: zh
|
396 |
+
split: validation
|
397 |
+
revision: a5a45e4ff92d5d3f34de70aaf4b72c3bdf9f7f16
|
398 |
+
metrics:
|
399 |
+
- type: Accuracy
|
400 |
+
value: 54.18
|
401 |
+
- task:
|
402 |
+
type: Program synthesis
|
403 |
+
dataset:
|
404 |
+
type: openai_humaneval
|
405 |
+
name: HumanEval
|
406 |
+
config: None
|
407 |
+
split: test
|
408 |
+
revision: e8dc562f5de170c54b5481011dd9f4fa04845771
|
409 |
+
metrics:
|
410 |
+
- type: Pass@1
|
411 |
+
value: 6.29
|
412 |
+
- type: Pass@10
|
413 |
+
value: 11.94
|
414 |
+
- type: Pass@100
|
415 |
+
value: 19.06
|
416 |
+
- task:
|
417 |
+
type: Sentence completion
|
418 |
+
dataset:
|
419 |
+
type: story_cloze
|
420 |
+
name: StoryCloze (2016)
|
421 |
+
config: "2016"
|
422 |
+
split: validation
|
423 |
+
revision: e724c6f8cdf7c7a2fb229d862226e15b023ee4db
|
424 |
+
metrics:
|
425 |
+
- type: Accuracy
|
426 |
+
value: 87.33
|
427 |
+
- task:
|
428 |
+
type: Sentence completion
|
429 |
+
dataset:
|
430 |
+
type: super_glue
|
431 |
+
name: SuperGLUE (copa)
|
432 |
+
config: copa
|
433 |
+
split: validation
|
434 |
+
revision: 9e12063561e7e6c79099feb6d5a493142584e9e2
|
435 |
+
metrics:
|
436 |
+
- type: Accuracy
|
437 |
+
value: 76.0
|
438 |
+
- task:
|
439 |
+
type: Sentence completion
|
440 |
+
dataset:
|
441 |
+
type: xcopa
|
442 |
+
name: XCOPA (et)
|
443 |
+
config: et
|
444 |
+
split: validation
|
445 |
+
revision: 37f73c60fb123111fa5af5f9b705d0b3747fd187
|
446 |
+
metrics:
|
447 |
+
- type: Accuracy
|
448 |
+
value: 53.0
|
449 |
+
- task:
|
450 |
+
type: Sentence completion
|
451 |
+
dataset:
|
452 |
+
type: xcopa
|
453 |
+
name: XCOPA (ht)
|
454 |
+
config: ht
|
455 |
+
split: validation
|
456 |
+
revision: 37f73c60fb123111fa5af5f9b705d0b3747fd187
|
457 |
+
metrics:
|
458 |
+
- type: Accuracy
|
459 |
+
value: 64.0
|
460 |
+
- task:
|
461 |
+
type: Sentence completion
|
462 |
+
dataset:
|
463 |
+
type: xcopa
|
464 |
+
name: XCOPA (id)
|
465 |
+
config: id
|
466 |
+
split: validation
|
467 |
+
revision: 37f73c60fb123111fa5af5f9b705d0b3747fd187
|
468 |
+
metrics:
|
469 |
+
- type: Accuracy
|
470 |
+
value: 70.0
|
471 |
+
- task:
|
472 |
+
type: Sentence completion
|
473 |
+
dataset:
|
474 |
+
type: xcopa
|
475 |
+
name: XCOPA (it)
|
476 |
+
config: it
|
477 |
+
split: validation
|
478 |
+
revision: 37f73c60fb123111fa5af5f9b705d0b3747fd187
|
479 |
+
metrics:
|
480 |
+
- type: Accuracy
|
481 |
+
value: 53.0
|
482 |
+
- task:
|
483 |
+
type: Sentence completion
|
484 |
+
dataset:
|
485 |
+
type: xcopa
|
486 |
+
name: XCOPA (qu)
|
487 |
+
config: qu
|
488 |
+
split: validation
|
489 |
+
revision: 37f73c60fb123111fa5af5f9b705d0b3747fd187
|
490 |
+
metrics:
|
491 |
+
- type: Accuracy
|
492 |
+
value: 56.0
|
493 |
+
- task:
|
494 |
+
type: Sentence completion
|
495 |
+
dataset:
|
496 |
+
type: xcopa
|
497 |
+
name: XCOPA (sw)
|
498 |
+
config: sw
|
499 |
+
split: validation
|
500 |
+
revision: 37f73c60fb123111fa5af5f9b705d0b3747fd187
|
501 |
+
metrics:
|
502 |
+
- type: Accuracy
|
503 |
+
value: 66.0
|
504 |
+
- task:
|
505 |
+
type: Sentence completion
|
506 |
+
dataset:
|
507 |
+
type: xcopa
|
508 |
+
name: XCOPA (ta)
|
509 |
+
config: ta
|
510 |
+
split: validation
|
511 |
+
revision: 37f73c60fb123111fa5af5f9b705d0b3747fd187
|
512 |
+
metrics:
|
513 |
+
- type: Accuracy
|
514 |
+
value: 59.0
|
515 |
+
- task:
|
516 |
+
type: Sentence completion
|
517 |
+
dataset:
|
518 |
+
type: xcopa
|
519 |
+
name: XCOPA (th)
|
520 |
+
config: th
|
521 |
+
split: validation
|
522 |
+
revision: 37f73c60fb123111fa5af5f9b705d0b3747fd187
|
523 |
+
metrics:
|
524 |
+
- type: Accuracy
|
525 |
+
value: 63.0
|
526 |
+
- task:
|
527 |
+
type: Sentence completion
|
528 |
+
dataset:
|
529 |
+
type: xcopa
|
530 |
+
name: XCOPA (tr)
|
531 |
+
config: tr
|
532 |
+
split: validation
|
533 |
+
revision: 37f73c60fb123111fa5af5f9b705d0b3747fd187
|
534 |
+
metrics:
|
535 |
+
- type: Accuracy
|
536 |
+
value: 61.0
|
537 |
+
- task:
|
538 |
+
type: Sentence completion
|
539 |
+
dataset:
|
540 |
+
type: xcopa
|
541 |
+
name: XCOPA (vi)
|
542 |
+
config: vi
|
543 |
+
split: validation
|
544 |
+
revision: 37f73c60fb123111fa5af5f9b705d0b3747fd187
|
545 |
+
metrics:
|
546 |
+
- type: Accuracy
|
547 |
+
value: 77.0
|
548 |
+
- task:
|
549 |
+
type: Sentence completion
|
550 |
+
dataset:
|
551 |
+
type: xcopa
|
552 |
+
name: XCOPA (zh)
|
553 |
+
config: zh
|
554 |
+
split: validation
|
555 |
+
revision: 37f73c60fb123111fa5af5f9b705d0b3747fd187
|
556 |
+
metrics:
|
557 |
+
- type: Accuracy
|
558 |
+
value: 73.0
|
559 |
+
- task:
|
560 |
+
type: Sentence completion
|
561 |
+
dataset:
|
562 |
+
type: Muennighoff/xstory_cloze
|
563 |
+
name: XStoryCloze (ar)
|
564 |
+
config: ar
|
565 |
+
split: validation
|
566 |
+
revision: 8bb76e594b68147f1a430e86829d07189622b90d
|
567 |
+
metrics:
|
568 |
+
- type: Accuracy
|
569 |
+
value: 80.61
|
570 |
+
- task:
|
571 |
+
type: Sentence completion
|
572 |
+
dataset:
|
573 |
+
type: Muennighoff/xstory_cloze
|
574 |
+
name: XStoryCloze (es)
|
575 |
+
config: es
|
576 |
+
split: validation
|
577 |
+
revision: 8bb76e594b68147f1a430e86829d07189622b90d
|
578 |
+
metrics:
|
579 |
+
- type: Accuracy
|
580 |
+
value: 85.9
|
581 |
+
- task:
|
582 |
+
type: Sentence completion
|
583 |
+
dataset:
|
584 |
+
type: Muennighoff/xstory_cloze
|
585 |
+
name: XStoryCloze (eu)
|
586 |
+
config: eu
|
587 |
+
split: validation
|
588 |
+
revision: 8bb76e594b68147f1a430e86829d07189622b90d
|
589 |
+
metrics:
|
590 |
+
- type: Accuracy
|
591 |
+
value: 70.95
|
592 |
+
- task:
|
593 |
+
type: Sentence completion
|
594 |
+
dataset:
|
595 |
+
type: Muennighoff/xstory_cloze
|
596 |
+
name: XStoryCloze (hi)
|
597 |
+
config: hi
|
598 |
+
split: validation
|
599 |
+
revision: 8bb76e594b68147f1a430e86829d07189622b90d
|
600 |
+
metrics:
|
601 |
+
- type: Accuracy
|
602 |
+
value: 78.89
|
603 |
+
- task:
|
604 |
+
type: Sentence completion
|
605 |
+
dataset:
|
606 |
+
type: Muennighoff/xstory_cloze
|
607 |
+
name: XStoryCloze (id)
|
608 |
+
config: id
|
609 |
+
split: validation
|
610 |
+
revision: 8bb76e594b68147f1a430e86829d07189622b90d
|
611 |
+
metrics:
|
612 |
+
- type: Accuracy
|
613 |
+
value: 82.99
|
614 |
+
- task:
|
615 |
+
type: Sentence completion
|
616 |
+
dataset:
|
617 |
+
type: Muennighoff/xstory_cloze
|
618 |
+
name: XStoryCloze (my)
|
619 |
+
config: my
|
620 |
+
split: validation
|
621 |
+
revision: 8bb76e594b68147f1a430e86829d07189622b90d
|
622 |
+
metrics:
|
623 |
+
- type: Accuracy
|
624 |
+
value: 49.9
|
625 |
+
- task:
|
626 |
+
type: Sentence completion
|
627 |
+
dataset:
|
628 |
+
type: Muennighoff/xstory_cloze
|
629 |
+
name: XStoryCloze (ru)
|
630 |
+
config: ru
|
631 |
+
split: validation
|
632 |
+
revision: 8bb76e594b68147f1a430e86829d07189622b90d
|
633 |
+
metrics:
|
634 |
+
- type: Accuracy
|
635 |
+
value: 61.42
|
636 |
+
- task:
|
637 |
+
type: Sentence completion
|
638 |
+
dataset:
|
639 |
+
type: Muennighoff/xstory_cloze
|
640 |
+
name: XStoryCloze (sw)
|
641 |
+
config: sw
|
642 |
+
split: validation
|
643 |
+
revision: 8bb76e594b68147f1a430e86829d07189622b90d
|
644 |
+
metrics:
|
645 |
+
- type: Accuracy
|
646 |
+
value: 69.69
|
647 |
+
- task:
|
648 |
+
type: Sentence completion
|
649 |
+
dataset:
|
650 |
+
type: Muennighoff/xstory_cloze
|
651 |
+
name: XStoryCloze (te)
|
652 |
+
config: te
|
653 |
+
split: validation
|
654 |
+
revision: 8bb76e594b68147f1a430e86829d07189622b90d
|
655 |
+
metrics:
|
656 |
+
- type: Accuracy
|
657 |
+
value: 73.66
|
658 |
+
- task:
|
659 |
+
type: Sentence completion
|
660 |
+
dataset:
|
661 |
+
type: Muennighoff/xstory_cloze
|
662 |
+
name: XStoryCloze (zh)
|
663 |
+
config: zh
|
664 |
+
split: validation
|
665 |
+
revision: 8bb76e594b68147f1a430e86829d07189622b90d
|
666 |
+
metrics:
|
667 |
+
- type: Accuracy
|
668 |
+
value: 84.32
|
669 |
+
---
|
670 |
+
|
671 |
+
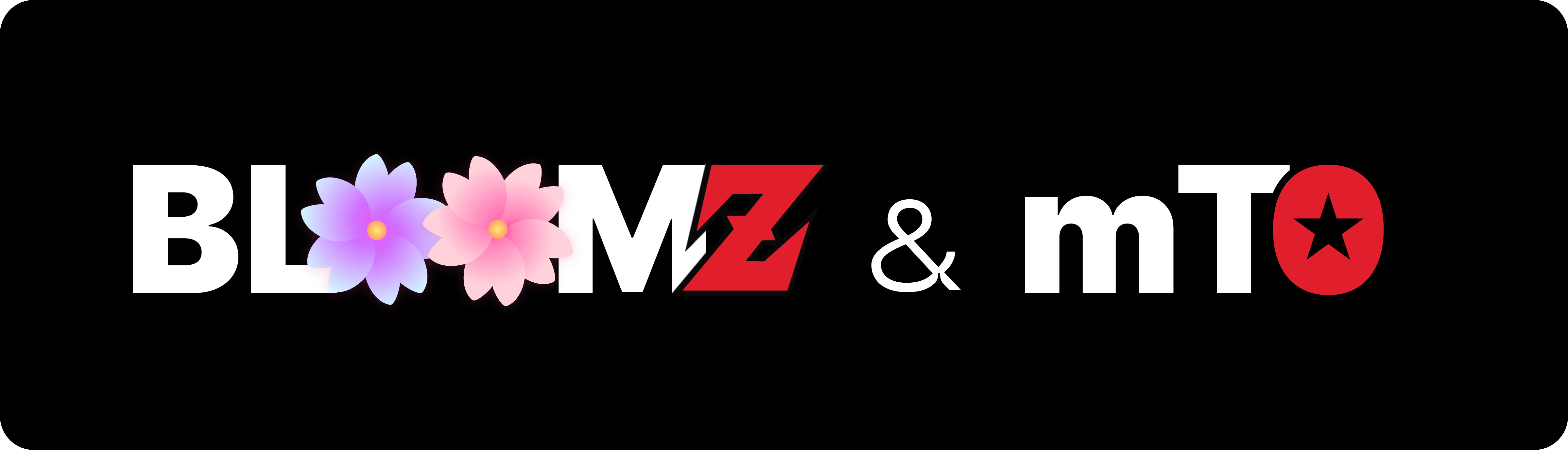
|
672 |
+
|
673 |
+
# Table of Contents
|
674 |
+
|
675 |
+
1. [Model Summary](#model-summary)
|
676 |
+
2. [Use](#use)
|
677 |
+
3. [Limitations](#limitations)
|
678 |
+
4. [Training](#training)
|
679 |
+
5. [Evaluation](#evaluation)
|
680 |
+
7. [Citation](#citation)
|
681 |
+
|
682 |
+
# Model Summary
|
683 |
+
|
684 |
+
> We present BLOOMZ & mT0, a family of models capable of following human instructions in dozens of languages zero-shot. We finetune BLOOM & mT5 pretrained multilingual language models on our crosslingual task mixture (xP3) and find the resulting models capable of crosslingual generalization to unseen tasks & languages.
|
685 |
+
|
686 |
+
- **Repository:** [bigscience-workshop/xmtf](https://github.com/bigscience-workshop/xmtf)
|
687 |
+
- **Paper:** [Crosslingual Generalization through Multitask Finetuning](https://arxiv.org/abs/2211.01786)
|
688 |
+
- **Point of Contact:** [Niklas Muennighoff](mailto:[email protected])
|
689 |
+
- **Languages:** Refer to [bloom](https://huggingface.co/bigscience/bloom) for pretraining & [xP3](https://huggingface.co/datasets/bigscience/xP3) for finetuning language proportions. It understands both pretraining & finetuning languages.
|
690 |
+
- **BLOOMZ & mT0 Model Family:**
|
691 |
+
|
692 |
+
<div class="max-w-full overflow-auto">
|
693 |
+
<table>
|
694 |
+
<tr>
|
695 |
+
<th colspan="12">Multitask finetuned on <a style="font-weight:bold" href=https://huggingface.co/datasets/bigscience/xP3>xP3</a>. Recommended for prompting in English.
|
696 |
+
</tr>
|
697 |
+
<tr>
|
698 |
+
<td>Parameters</td>
|
699 |
+
<td>300M</td>
|
700 |
+
<td>580M</td>
|
701 |
+
<td>1.2B</td>
|
702 |
+
<td>3.7B</td>
|
703 |
+
<td>13B</td>
|
704 |
+
<td>560M</td>
|
705 |
+
<td>1.1B</td>
|
706 |
+
<td>1.7B</td>
|
707 |
+
<td>3B</td>
|
708 |
+
<td>7.1B</td>
|
709 |
+
<td>176B</td>
|
710 |
+
</tr>
|
711 |
+
<tr>
|
712 |
+
<td>Finetuned Model</td>
|
713 |
+
<td><a href=https://huggingface.co/bigscience/mt0-small>mt0-small</a></td>
|
714 |
+
<td><a href=https://huggingface.co/bigscience/mt0-base>mt0-base</a></td>
|
715 |
+
<td><a href=https://huggingface.co/bigscience/mt0-large>mt0-large</a></td>
|
716 |
+
<td><a href=https://huggingface.co/bigscience/mt0-xl>mt0-xl</a></td>
|
717 |
+
<td><a href=https://huggingface.co/bigscience/mt0-xxl>mt0-xxl</a></td>
|
718 |
+
<td><a href=https://huggingface.co/bigscience/bloomz-560m>bloomz-560m</a></td>
|
719 |
+
<td><a href=https://huggingface.co/bigscience/bloomz-1b1>bloomz-1b1</a></td>
|
720 |
+
<td><a href=https://huggingface.co/bigscience/bloomz-1b7>bloomz-1b7</a></td>
|
721 |
+
<td><a href=https://huggingface.co/bigscience/bloomz-3b>bloomz-3b</a></td>
|
722 |
+
<td><a href=https://huggingface.co/bigscience/bloomz-7b1>bloomz-7b1</a></td>
|
723 |
+
<td><a href=https://huggingface.co/bigscience/bloomz>bloomz</a></td>
|
724 |
+
</tr>
|
725 |
+
</tr>
|
726 |
+
<tr>
|
727 |
+
<th colspan="12">Multitask finetuned on <a style="font-weight:bold" href=https://huggingface.co/datasets/bigscience/xP3mt>xP3mt</a>. Recommended for prompting in non-English.</th>
|
728 |
+
</tr>
|
729 |
+
<tr>
|
730 |
+
<td>Finetuned Model</td>
|
731 |
+
<td></td>
|
732 |
+
<td></td>
|
733 |
+
<td></td>
|
734 |
+
<td></td>
|
735 |
+
<td><a href=https://huggingface.co/bigscience/mt0-xxl-mt>mt0-xxl-mt</a></td>
|
736 |
+
<td></td>
|
737 |
+
<td></td>
|
738 |
+
<td></td>
|
739 |
+
<td></td>
|
740 |
+
<td><a href=https://huggingface.co/bigscience/bloomz-7b1-mt>bloomz-7b1-mt</a></td>
|
741 |
+
<td><a href=https://huggingface.co/bigscience/bloomz-mt>bloomz-mt</a></td>
|
742 |
+
</tr>
|
743 |
+
<th colspan="12">Multitask finetuned on <a style="font-weight:bold" href=https://huggingface.co/datasets/Muennighoff/P3>P3</a>. Released for research purposes only. Strictly inferior to above models!</th>
|
744 |
+
</tr>
|
745 |
+
<tr>
|
746 |
+
<td>Finetuned Model</td>
|
747 |
+
<td></td>
|
748 |
+
<td></td>
|
749 |
+
<td></td>
|
750 |
+
<td></td>
|
751 |
+
<td><a href=https://huggingface.co/bigscience/mt0-xxl-p3>mt0-xxl-p3</a></td>
|
752 |
+
<td></td>
|
753 |
+
<td></td>
|
754 |
+
<td></td>
|
755 |
+
<td></td>
|
756 |
+
<td><a href=https://huggingface.co/bigscience/bloomz-7b1-p3>bloomz-7b1-p3</a></td>
|
757 |
+
<td><a href=https://huggingface.co/bigscience/bloomz-p3>bloomz-p3</a></td>
|
758 |
+
</tr>
|
759 |
+
<th colspan="12">Original pretrained checkpoints. Not recommended.</th>
|
760 |
+
<tr>
|
761 |
+
<td>Pretrained Model</td>
|
762 |
+
<td><a href=https://huggingface.co/google/mt5-small>mt5-small</a></td>
|
763 |
+
<td><a href=https://huggingface.co/google/mt5-base>mt5-base</a></td>
|
764 |
+
<td><a href=https://huggingface.co/google/mt5-large>mt5-large</a></td>
|
765 |
+
<td><a href=https://huggingface.co/google/mt5-xl>mt5-xl</a></td>
|
766 |
+
<td><a href=https://huggingface.co/google/mt5-xxl>mt5-xxl</a></td>
|
767 |
+
<td><a href=https://huggingface.co/bigscience/bloom-560m>bloom-560m</a></td>
|
768 |
+
<td><a href=https://huggingface.co/bigscience/bloom-1b1>bloom-1b1</a></td>
|
769 |
+
<td><a href=https://huggingface.co/bigscience/bloom-1b7>bloom-1b7</a></td>
|
770 |
+
<td><a href=https://huggingface.co/bigscience/bloom-3b>bloom-3b</a></td>
|
771 |
+
<td><a href=https://huggingface.co/bigscience/bloom-7b1>bloom-7b1</a></td>
|
772 |
+
<td><a href=https://huggingface.co/bigscience/bloom>bloom</a></td>
|
773 |
+
</tr>
|
774 |
+
</table>
|
775 |
+
</div>
|
776 |
+
|
777 |
+
|
778 |
+
# Use
|
779 |
+
|
780 |
+
## Intended use
|
781 |
+
|
782 |
+
We recommend using the model to perform tasks expressed in natural language. For example, given the prompt "*Translate to English: Je t’aime.*", the model will most likely answer "*I love you.*". Some prompt ideas from our paper:
|
783 |
+
- 一个传奇的开端,一个不灭的神话,这不仅仅是一部电影,而是作为一个走进新时代的标签,永远彪炳史册。你认为这句话的立场是赞扬、中立还是批评?
|
784 |
+
- Suggest at least five related search terms to "Mạng neural nhân tạo".
|
785 |
+
- Write a fairy tale about a troll saving a princess from a dangerous dragon. The fairy tale is a masterpiece that has achieved praise worldwide and its moral is "Heroes Come in All Shapes and Sizes". Story (in Spanish):
|
786 |
+
- Explain in a sentence in Telugu what is backpropagation in neural networks.
|
787 |
+
|
788 |
+
**Feel free to share your generations in the Community tab!**
|
789 |
+
|
790 |
+
## How to use
|
791 |
+
|
792 |
+
### CPU
|
793 |
+
|
794 |
+
<details>
|
795 |
+
<summary> Click to expand </summary>
|
796 |
+
|
797 |
+
```python
|
798 |
+
# pip install -q transformers
|
799 |
+
from transformers import AutoModelForCausalLM, AutoTokenizer
|
800 |
+
|
801 |
+
checkpoint = "bigscience/bloomz-3b"
|
802 |
+
|
803 |
+
tokenizer = AutoTokenizer.from_pretrained(checkpoint)
|
804 |
+
model = AutoModelForCausalLM.from_pretrained(checkpoint)
|
805 |
+
|
806 |
+
inputs = tokenizer.encode("Translate to English: Je t’aime.", return_tensors="pt")
|
807 |
+
outputs = model.generate(inputs)
|
808 |
+
print(tokenizer.decode(outputs[0]))
|
809 |
+
```
|
810 |
+
|
811 |
+
</details>
|
812 |
+
|
813 |
+
### GPU
|
814 |
+
|
815 |
+
<details>
|
816 |
+
<summary> Click to expand </summary>
|
817 |
+
|
818 |
+
```python
|
819 |
+
# pip install -q transformers accelerate
|
820 |
+
from transformers import AutoModelForCausalLM, AutoTokenizer
|
821 |
+
|
822 |
+
checkpoint = "bigscience/bloomz-3b"
|
823 |
+
|
824 |
+
tokenizer = AutoTokenizer.from_pretrained(checkpoint)
|
825 |
+
model = AutoModelForCausalLM.from_pretrained(checkpoint, torch_dtype="auto", device_map="auto")
|
826 |
+
|
827 |
+
inputs = tokenizer.encode("Translate to English: Je t’aime.", return_tensors="pt").to("cuda")
|
828 |
+
outputs = model.generate(inputs)
|
829 |
+
print(tokenizer.decode(outputs[0]))
|
830 |
+
```
|
831 |
+
|
832 |
+
</details>
|
833 |
+
|
834 |
+
### GPU in 8bit
|
835 |
+
|
836 |
+
<details>
|
837 |
+
<summary> Click to expand </summary>
|
838 |
+
|
839 |
+
```python
|
840 |
+
# pip install -q transformers accelerate bitsandbytes
|
841 |
+
from transformers import AutoModelForCausalLM, AutoTokenizer
|
842 |
+
|
843 |
+
checkpoint = "bigscience/bloomz-3b"
|
844 |
+
|
845 |
+
tokenizer = AutoTokenizer.from_pretrained(checkpoint)
|
846 |
+
model = AutoModelForCausalLM.from_pretrained(checkpoint, device_map="auto", load_in_8bit=True)
|
847 |
+
|
848 |
+
inputs = tokenizer.encode("Translate to English: Je t’aime.", return_tensors="pt").to("cuda")
|
849 |
+
outputs = model.generate(inputs)
|
850 |
+
print(tokenizer.decode(outputs[0]))
|
851 |
+
```
|
852 |
+
|
853 |
+
</details>
|
854 |
+
|
855 |
+
<!-- Necessary for whitespace -->
|
856 |
+
###
|
857 |
+
|
858 |
+
# Limitations
|
859 |
+
|
860 |
+
**Prompt Engineering:** The performance may vary depending on the prompt. For BLOOMZ models, we recommend making it very clear when the input stops to avoid the model trying to continue it. For example, the prompt "*Translate to English: Je t'aime*" without the full stop (.) at the end, may result in the model trying to continue the French sentence. Better prompts are e.g. "*Translate to English: Je t'aime.*", "*Translate to English: Je t'aime. Translation:*" "*What is "Je t'aime." in English?*", where it is clear for the model when it should answer. Further, we recommend providing the model as much context as possible. For example, if you want it to answer in Telugu, then tell the model, e.g. "*Explain in a sentence in Telugu what is backpropagation in neural networks.*".
|
861 |
+
|
862 |
+
# Training
|
863 |
+
|
864 |
+
## Model
|
865 |
+
|
866 |
+
- **Architecture:** Same as [bloom-3b](https://huggingface.co/bigscience/bloom-3b), also refer to the `config.json` file
|
867 |
+
- **Finetuning steps:** 2000
|
868 |
+
- **Finetuning tokens:** 8.39 billion
|
869 |
+
- **Finetuning layout:** 2x pipeline parallel, 1x tensor parallel, 64x data parallel
|
870 |
+
- **Precision:** float16
|
871 |
+
|
872 |
+
## Hardware
|
873 |
+
|
874 |
+
- **CPUs:** AMD CPUs with 512GB memory per node
|
875 |
+
- **GPUs:** 128 A100 80GB GPUs with 8 GPUs per node (16 nodes) using NVLink 4 inter-gpu connects, 4 OmniPath links
|
876 |
+
- **Communication:** NCCL-communications network with a fully dedicated subnet
|
877 |
+
|
878 |
+
## Software
|
879 |
+
|
880 |
+
- **Orchestration:** [Megatron-DeepSpeed](https://github.com/bigscience-workshop/Megatron-DeepSpeed)
|
881 |
+
- **Optimizer & parallelism:** [DeepSpeed](https://github.com/microsoft/DeepSpeed)
|
882 |
+
- **Neural networks:** [PyTorch](https://github.com/pytorch/pytorch) (pytorch-1.11 w/ CUDA-11.5)
|
883 |
+
- **FP16 if applicable:** [apex](https://github.com/NVIDIA/apex)
|
884 |
+
|
885 |
+
# Evaluation
|
886 |
+
|
887 |
+
We refer to Table 7 from our [paper](https://arxiv.org/abs/2211.01786) & [bigscience/evaluation-results](https://huggingface.co/datasets/bigscience/evaluation-results) for zero-shot results on unseen tasks. The sidebar reports zero-shot performance of the best prompt per dataset config.
|
888 |
+
|
889 |
+
# Citation
|
890 |
+
```bibtex
|
891 |
+
@article{muennighoff2022crosslingual,
|
892 |
+
title={Crosslingual generalization through multitask finetuning},
|
893 |
+
author={Muennighoff, Niklas and Wang, Thomas and Sutawika, Lintang and Roberts, Adam and Biderman, Stella and Scao, Teven Le and Bari, M Saiful and Shen, Sheng and Yong, Zheng-Xin and Schoelkopf, Hailey and others},
|
894 |
+
journal={arXiv preprint arXiv:2211.01786},
|
895 |
+
year={2022}
|
896 |
+
}
|
897 |
+
```
|
898 |
+
|